PERFEX: Classifier Performance Explanations for Trustworthy AI Systems
arxiv(2022)
摘要
Explainability of a classification model is crucial when deployed in real-world decision support systems. Explanations make predictions actionable to the user and should inform about the capabilities and limitations of the system. Existing explanation methods, however, typically only provide explanations for individual predictions. Information about conditions under which the classifier is able to support the decision maker is not available, while for instance information about when the system is not able to differentiate classes can be very helpful. In the development phase it can support the search for new features or combining models, and in the operational phase it supports decision makers in deciding e.g. not to use the system. This paper presents a method to explain the qualities of a trained base classifier, called PERFormance EXplainer (PERFEX). Our method consists of a meta tree learning algorithm that is able to predict and explain under which conditions the base classifier has a high or low error or any other classification performance metric. We evaluate PERFEX using several classifiers and datasets, including a case study with urban mobility data. It turns out that PERFEX typically has high meta prediction performance even if the base classifier is hardly able to differentiate classes, while giving compact performance explanations.
更多查看译文
关键词
trustworthy ai,classifier performance explanations,perfex
AI 理解论文
溯源树
样例
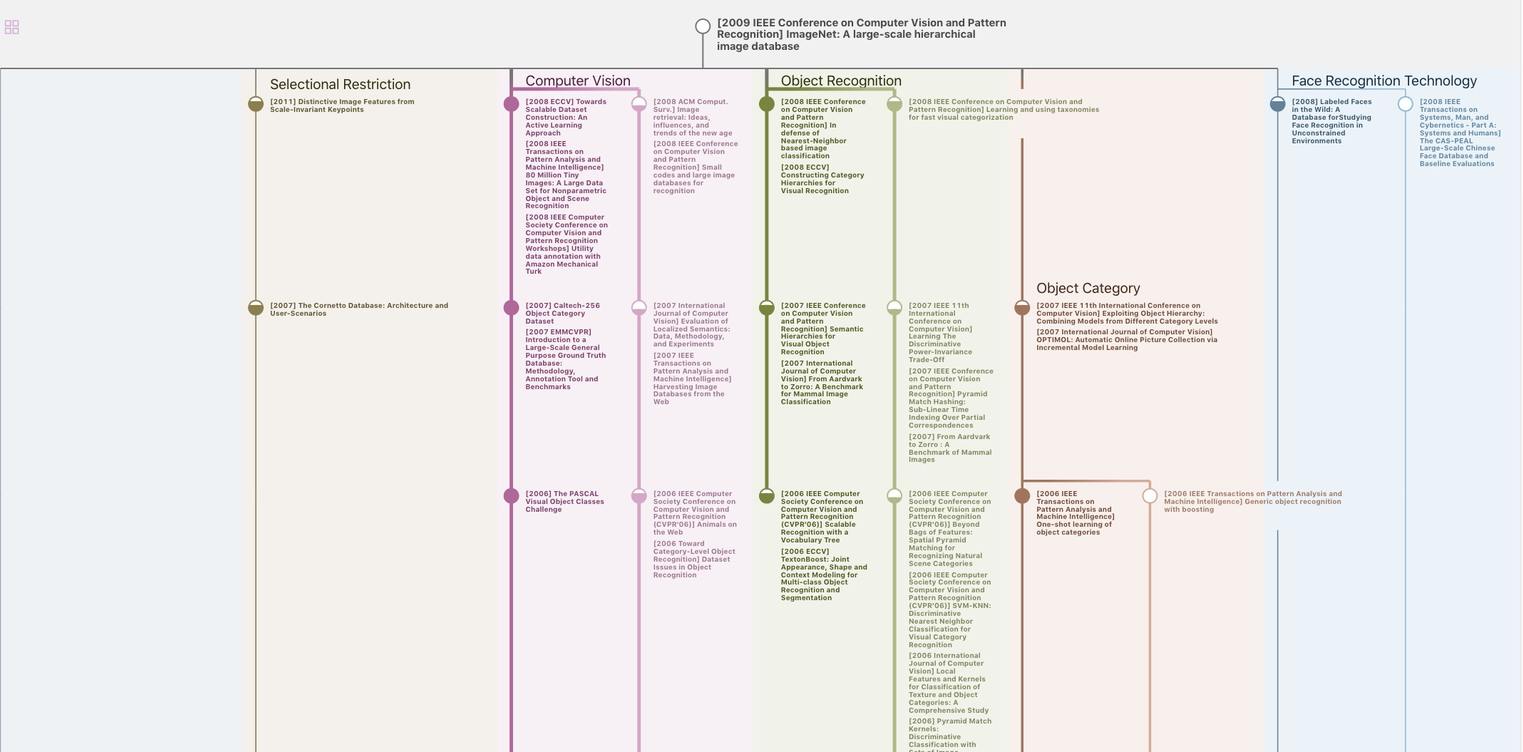
生成溯源树,研究论文发展脉络
Chat Paper
正在生成论文摘要