ALRt: An Active Learning Framework for Irregularly Sampled Temporal Data
CoRR(2022)
摘要
Sepsis is a deadly condition affecting many patients in the hospital. Recent studies have shown that patients diagnosed with sepsis have significant mortality and morbidity, resulting from the body's dysfunctional host response to infection. Clinicians often rely on the use of Sequential Organ Failure Assessment (SOFA), Systemic Inflammatory Response Syndrome (SIRS), and the Modified Early Warning Score (MEWS) to identify early signs of clinical deterioration requiring further work-up and treatment. However, many of these tools are manually computed and were not designed for automated computation. There have been different methods used for developing sepsis onset models, but many of these models must be trained on a sufficient number of patient observations in order to form accurate sepsis predictions. Additionally, the accurate annotation of patients with sepsis is a major ongoing challenge. In this paper, we propose the use of Active Learning Recurrent Neural Networks (ALRts) for short temporal horizons to improve the prediction of irregularly sampled temporal events such as sepsis. We show that an active learning RNN model trained on limited data can form robust sepsis predictions comparable to models using the entire training dataset.
更多查看译文
关键词
sampled temporal data,active learning,active learning framework
AI 理解论文
溯源树
样例
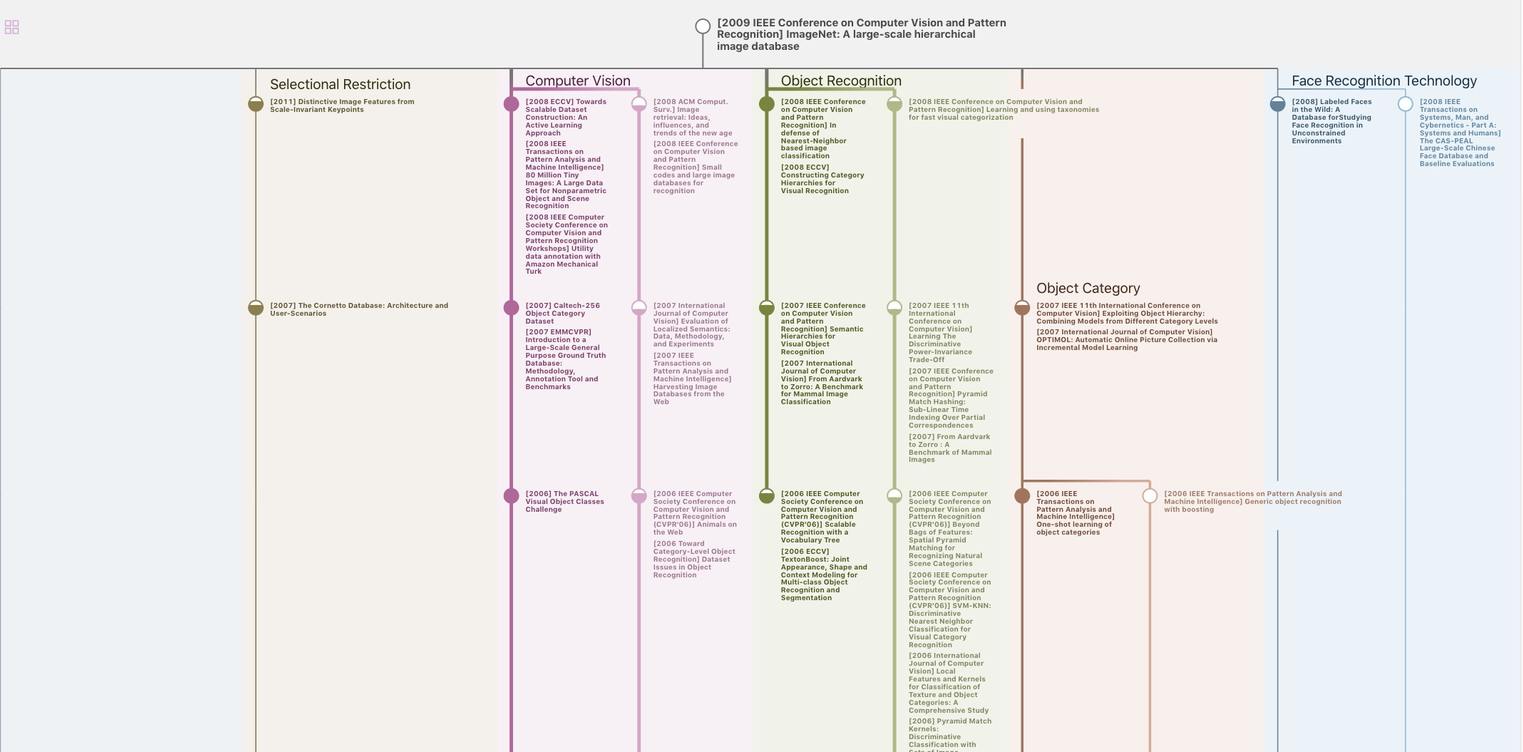
生成溯源树,研究论文发展脉络
Chat Paper
正在生成论文摘要