ADEV: Sound Automatic Differentiation of Expected Values of Probabilistic Programs.
Proc. ACM Program. Lang.(2023)
摘要
Optimizing the expected values of probabilistic processes is a central problem in computer science and its applications, arising in fields ranging from artificial intelligence to operations research to statistical computing. Unfortunately, automatic differentiation techniques developed for deterministic programs do not in general compute the correct gradients needed for widely used solutions based on gradient-based optimization. In this paper, we present ADEV, an extension to forward-mode AD that correctly differentiates the expectations of probabilistic processes represented as programs that make random choices. Our algorithm is a source-to-source program transformation on an expressive, higher-order language for probabilistic computation, with both discrete and continuous probability distributions. The result of our transformation is a new probabilistic program, whose expected return value is the derivative of the original program's expectation. This output program can be run to generate unbiased Monte Carlo estimates of the desired gradient, which can then be used within the inner loop of stochastic gradient descent. We prove ADEV correct using logical relations over the denotations of the source and target probabilistic programs. Because it modularly extends forward-mode AD, our algorithm lends itself to a concise implementation strategy, which we exploit to develop a prototype in just a few dozen lines of Haskell (https://github.com/probcomp/adev).
更多查看译文
关键词
probabilistic programming,automatic differentiation,denotational semantics,logical relations,functional programming,correctness,machine learning theory
AI 理解论文
溯源树
样例
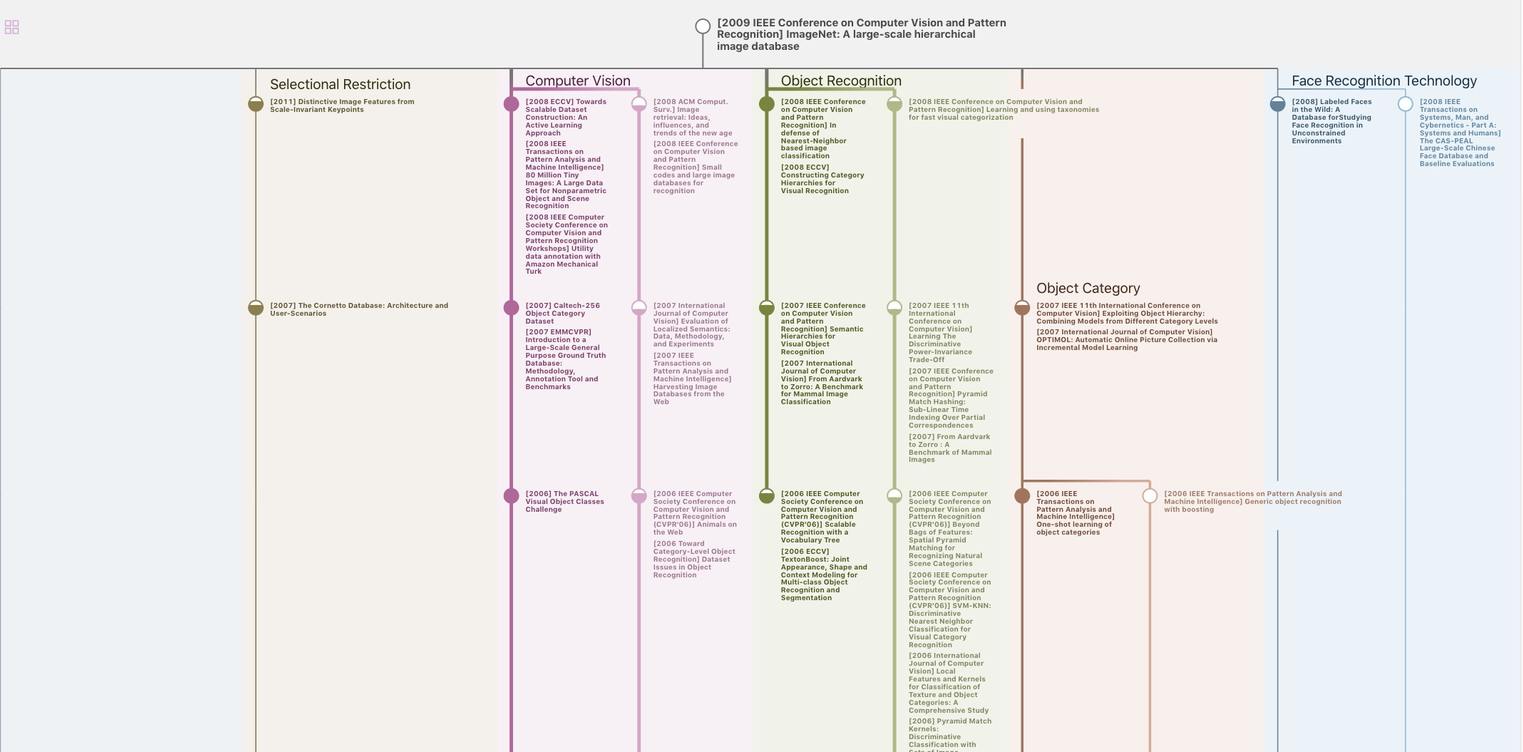
生成溯源树,研究论文发展脉络
Chat Paper
正在生成论文摘要