Improving the Performance of Sleep Apnea Screening System using Wavelets and Bottleneck Feature Fusion
2022 International Conference on Distributed Computing, VLSI, Electrical Circuits and Robotics ( DISCOVER)(2022)
摘要
S1eep apnea is the one of the most prevalent sleep disorder caused due to obstruction in breathing. Sleep apnea detection is usually done using polysomnography (PSG) which is not available for rural health care. The main objective of this work is to develop an affordable sleep apnea screening system using electrocardiography (ECG) signals as input.The baseline system was built using statistical features extracted from the time domain, frequency domain, and wavelet decomposed signals as input to a support vector machine (SVM) backend classifier. The baseline showed an accuracy of 86%, specificity of 83%, and sensitivity of 88%. Further, a Convolutional neural network (CNN) model is also implemented to check the performance of the system on wavelet decomposed signals. The best CNN model gave an accuracy of 86.6%, a sensitivity of 84.01%, and a specificity of 84.1%.To enhance the performance further, bottleneck features were extracted from the bottleneck layer of a CNN and the features thus derived are combined for feature fusion. The bottleneck layer compresses the model aiding in the extraction of lower dimensionality information. The bottleneck features from the best-performing models are fused together. The performance of the fused bottleneck features was found to show an accuracy of 87.6%, sensitivity of 86.4%, and specificity of 86.49%.
更多查看译文
关键词
Sleep apnea,PSG,ECG,Wavelet decomposition,SVM,CNN,Bottleneck feature fusion
AI 理解论文
溯源树
样例
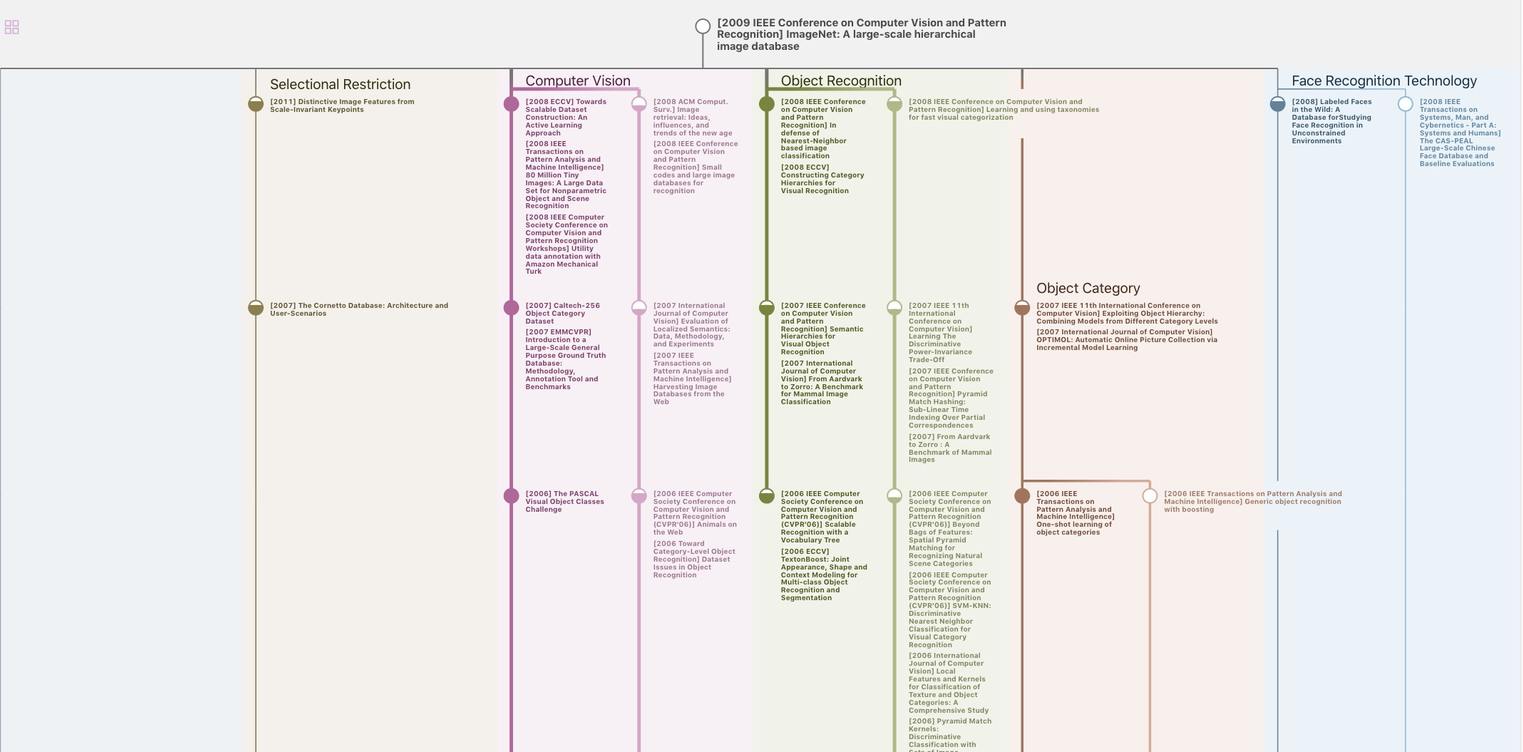
生成溯源树,研究论文发展脉络
Chat Paper
正在生成论文摘要