Unsupervised Feature Learning in Activity Recognition using Convolutional Denoising Autoencoders with Squeeze and Excitation Networks
2022 5th International Conference on Information and Communications Technology (ICOIACT)(2022)
摘要
Sensor modalities have recently been used to acquire activity recognition data, especially wearable sensors, due to their relative advantages over other modalities. Datasets obtained using wearable sensors are often in time-series format; therefore, there is a need to segment and extract only salient features from the raw sensor data. Generally, the overall performance of activity recognition relies heavily on the quality of these extracted features. For this reason, various feature extraction approaches have been proposed in the literature, ranging from manual to automatic feature extraction methods. However, most of the existing feature extraction methods are time-consuming and supervised. Since wearable sensor data is quite tedious and time-consuming to label, there is a need to propose unsupervised feature extraction methods capable of extracting features from fully unlabelled wearable sensor datasets. Several autoencoder models have been proposed for unsupervised feature learning using fully connected and convolutional neural networks. However, these methods cannot effectively learn discriminative features since they treat all features as equally important. To tackle this, this research introduced a feature weighting mechanism based on squeeze and excitation networks in a convolutional denoising autoencoder to act as channel-wise attention to reweigh feature channels accordingly. This allowed more discriminative activity features to be extracted from fully unlabelled activity data. The proposed model was benchmarked on two publicly available datasets, including a dataset which consists of transitional activities (WISDM and HAPT). Results showed that the proposed method exploited more discriminative features while learning inherent features of unlabelled activity recognition data and outperformed the existing methods with improved recognition performance on the two benchmark datasets.
更多查看译文
关键词
features,convolutional,autoencoder,learning,activity,recognition
AI 理解论文
溯源树
样例
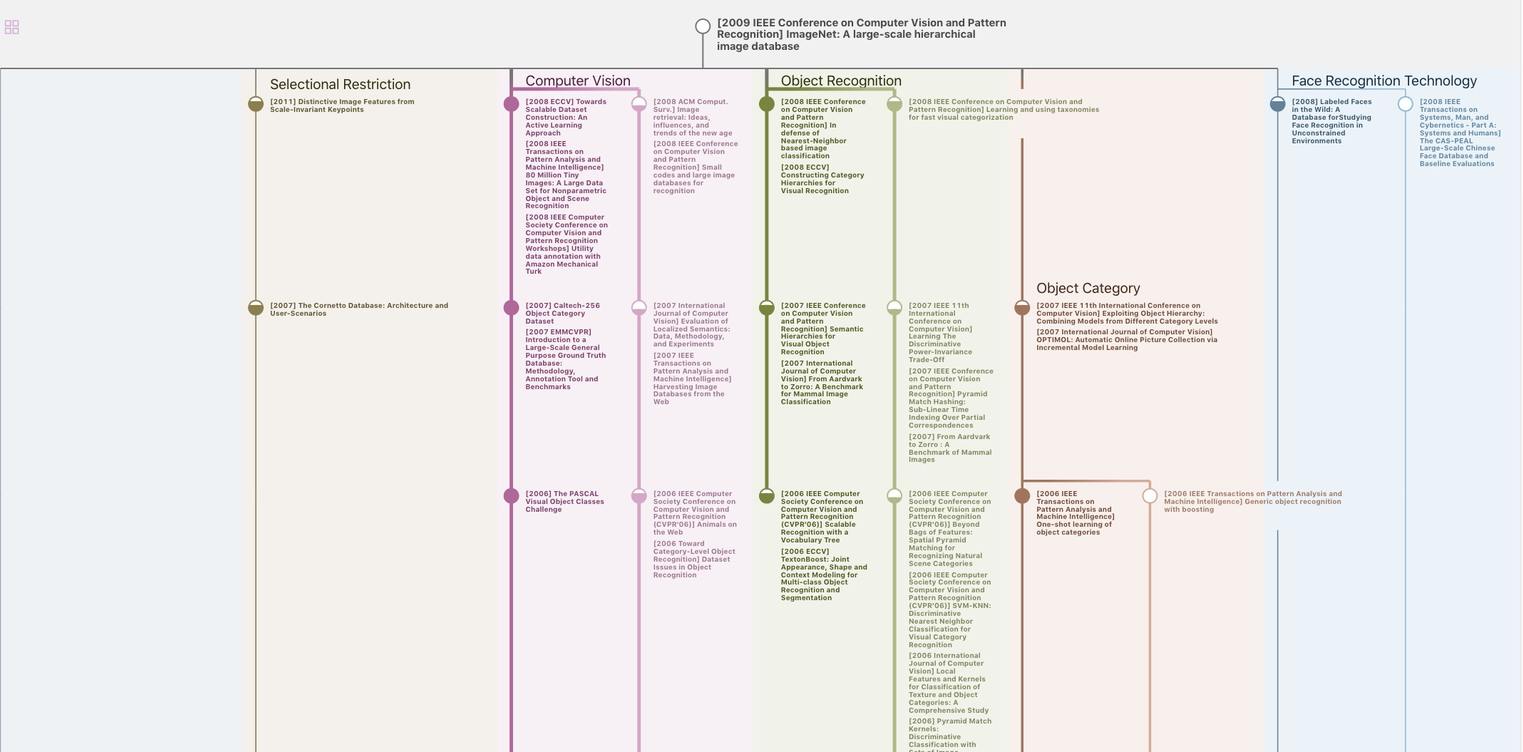
生成溯源树,研究论文发展脉络
Chat Paper
正在生成论文摘要