Deep learning based on carotid transverse B-mode scan videos for the diagnosis of carotid plaque: a prospective multicenter study
European radiology(2022)
摘要
Objectives Accurate detection of carotid plaque using ultrasound (US) is essential for preventing stroke. However, the diagnostic performance of junior radiologists (with approximately 1 year of experience in carotid US evaluation) is relatively poor. We thus aim to develop a deep learning (DL) model based on US videos to improve junior radiologists’ performance in plaque detection. Methods This multicenter prospective study was conducted at five hospitals. CaroNet-Dynamic automatically detected carotid plaque from carotid transverse US videos allowing clinical detection. Model performance was evaluated using expert annotations (with more than 10 years of experience in carotid US evaluation) as the ground truth. Model robustness was investigated on different plaque characteristics and US scanning systems. Furthermore, its clinical applicability was evaluated by comparing the junior radiologists’ diagnoses with and without DL-model assistance. Results A total of 1647 videos from 825 patients were evaluated. The DL model yielded high performance with sensitivities of 87.03% and 94.17%, specificities of 82.07% and 74.04%, and areas under the receiver operating characteristic curve of 0.845 and 0.841 on the internal and multicenter external test sets, respectively. Moreover, no significant difference in performance was noted among different plaque characteristics and scanning systems. Using the DL model, the performance of the junior radiologists improved significantly, especially in terms of sensitivity (largest increase from 46.3 to 94.44%). Conclusions The DL model based on US videos corresponding to real examinations showed robust performance for plaque detection and significantly improved the diagnostic performance of junior radiologists. Key Points • The deep learning model based on US videos conforming to real examinations showed robust performance for plaque detection. • Computer-aided diagnosis can significantly improve the diagnostic performance of junior radiologists in clinical practice.
更多查看译文
关键词
Atherosclerosis,Carotid arteries,Computer-assisted image interpretation,Deep learning,Ultrasonography
AI 理解论文
溯源树
样例
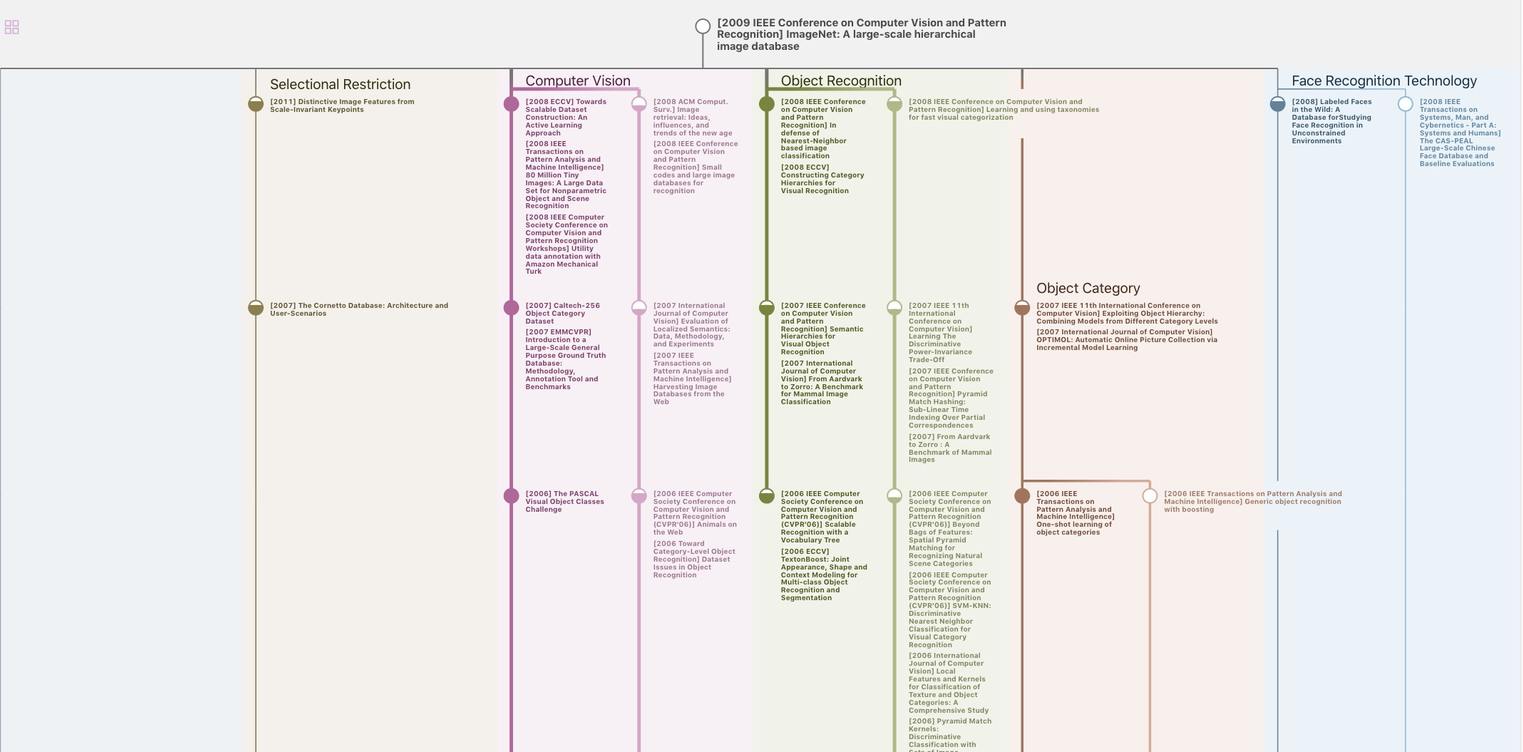
生成溯源树,研究论文发展脉络
Chat Paper
正在生成论文摘要