Federated Clustering for Recognizing Driving Styles from Private Trajectories
ENGINEERING APPLICATIONS OF ARTIFICIAL INTELLIGENCE(2023)
摘要
Driving style recognition of real-world drivers is beneficial for various reasons, such as safe and economic driving, auto-insurance and designing autonomous systems. A common way to achieve this goal is to group drivers using clustering methods according to their trajectory data. However, the conventional model training process is centralized, where all drivers’ private trajectories are collected and shared, which has resulted in privacy concerns. Considering that the driving data are sourced from various vehicles and have a decentralized distribution, we introduce a federated clustering approach for privacy-preserving driving style recognition. The method preserves the trajectory data in edge devices, such as connected vehicles or roadside units, and allows only the exchange of model parameters and not raw, sensitive data under the coordination of a central server to meet the privacy protection requirements. To address the clustering heterogeneous challenge posed by the imbalanced distribution of trajectory data in this setting, we combine local Bayesian Gaussian mixture and global weighted K-means to output high-quality global initialization centers. Then, novel local training and global aggregation strategies are proposed to ensure the convergence of training and improve the performance of the final global model. Through comparison experiments on benchmark and real-world datasets, we conclude that this method outperforms existing methods.
更多查看译文
关键词
Driving style,Privacy protection,Federated learning,Federated clustering,Bayesian Gaussian Mixture
AI 理解论文
溯源树
样例
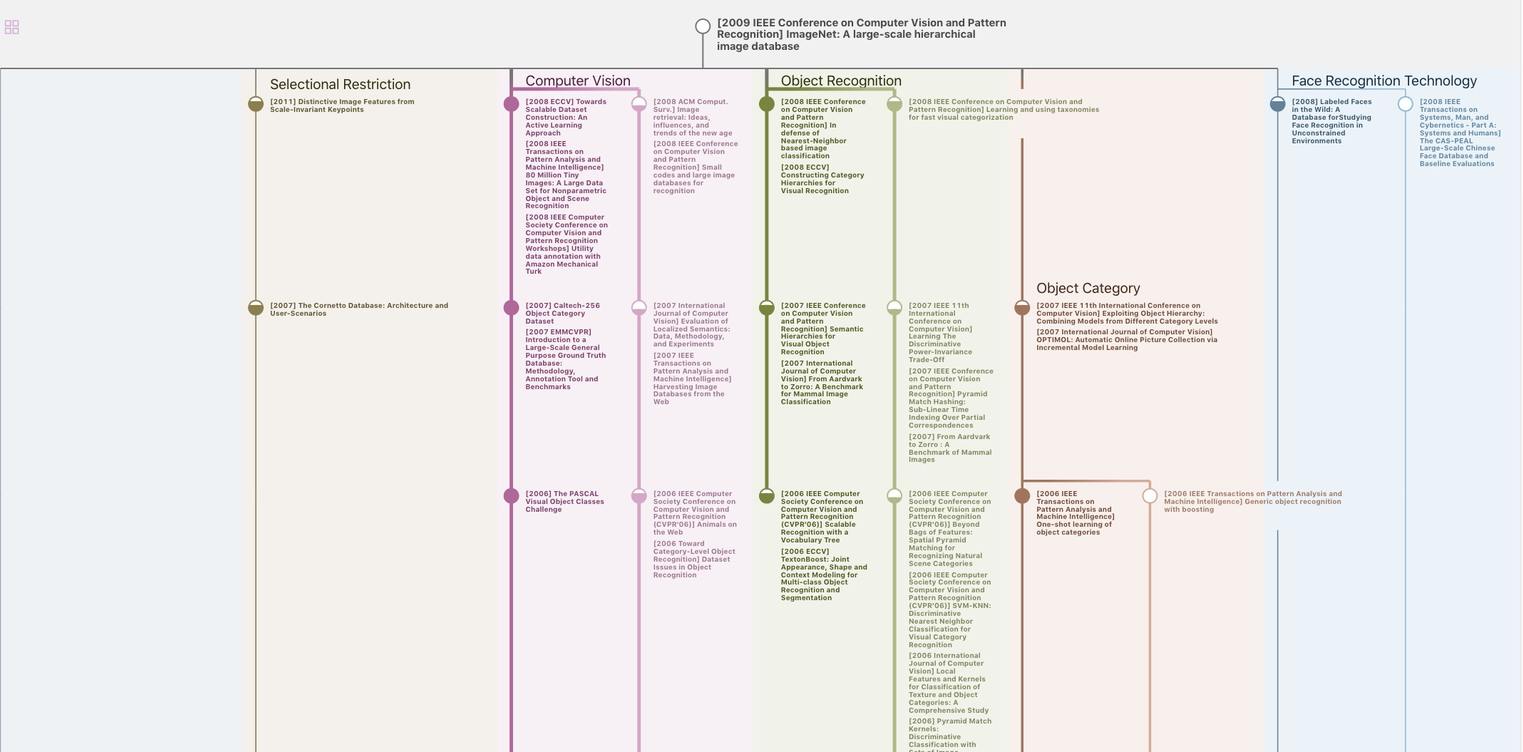
生成溯源树,研究论文发展脉络
Chat Paper
正在生成论文摘要