A deep transfer learning -based protocol accelerates full quantum mechanics calculation of protein
Briefings in bioinformatics(2023)
摘要
Effective full quantum mechanics (FQM) calculation of protein remains a grand challenge and of great interest in computational biology with substantial applications in drug discovery, protein dynamic simulation and protein folding. However, the huge computational complexity of the existing QM methods impends their applications in large systems. Here, we design a transfer-learning -based deep learning (TDL) protocol for effective FQM calculations (TDL-FQM) on proteins. By incorporating a transfer-learning algorithm into deep neural network (DNN), the TDL-FQM protocol is capable of performing calculations at any given accuracy using models trained from small datasets with high -precision and knowledge learned from large amount of low-level calculations. The high-level double -hybrid DFT functional and high-level quality of basis set is used in this work as a case study to evaluate the performance of TDL-FQM, where the selected 15 proteins are predicted to have a mean absolute error of 0.01 kcal/mol/atom for potential energy and an average root mean square error of 1.47 kcal/mol/A for atomic forces. The proposed TDL-FQM approach accelerates the FQM calculation more than thirty thousand times faster in average and presents more significant benefits in efficiency as the size of protein increases. The ability to learn knowledge from one task to solve related problems demonstrates that the proposed TDL-FQM overcomes the limitation of standard DNN and has a strong power to predict proteins with high precision, which solves the challenge of high precision prediction in large chemical and biological systems.
更多查看译文
关键词
full-quantum mechanics (FQM),deep transfer learning,many-body fragmentation,protein calculation
AI 理解论文
溯源树
样例
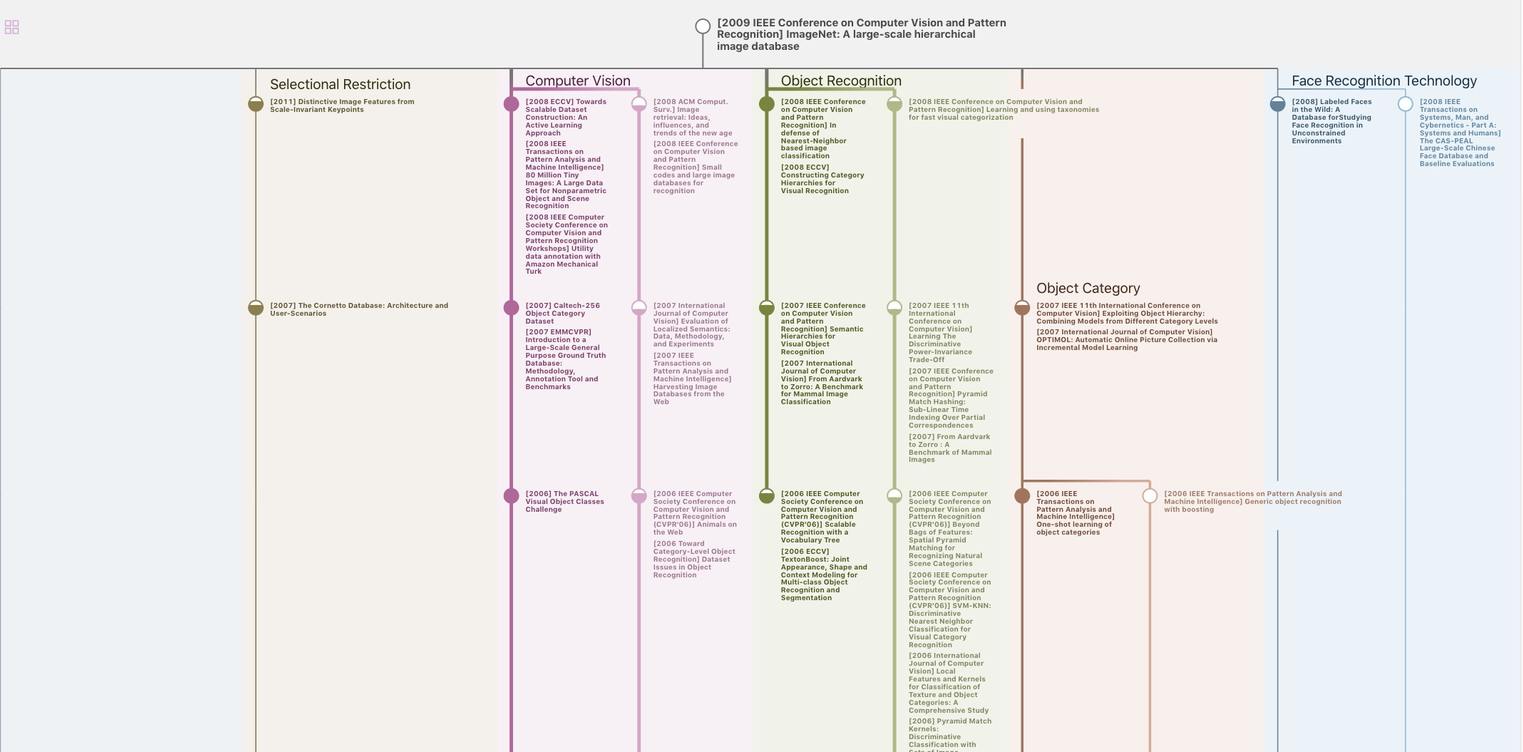
生成溯源树,研究论文发展脉络
Chat Paper
正在生成论文摘要