Bayesian Data Fusion With Shared Priors
IEEE TRANSACTIONS ON SIGNAL PROCESSING(2024)
摘要
The integration of data and knowledge from several sources is known as data fusion. When data is only available in a distributed fashion or when different sensors are used to infer a quantity of interest, data fusion becomes essential. In Bayesian settings, a priori information of the unknown quantities is available and, possibly, present among the different distributed estimators. When the local estimates are fused, the prior knowledge used to construct several local posteriors might be overused unless the fusion node accounts for this and corrects it. In this paper, we analyze the effects of shared priors in Bayesian data fusion contexts. Depending on different common fusion rules, our analysis helps to understand the performance behavior as a function of the number of collaborative agents and as a consequence of different types of priors. The analysis is performed by using two divergences which are common in Bayesian inference, and the generality of the results allows to analyze very generic distributions. These theoretical results are corroborated through experiments in a variety of estimation and classification problems, including linear and nonlinear models, and federated learning schemes.
更多查看译文
关键词
Bayes methods,Data integration,Distributed databases,Federated learning,Signal processing,Estimation,Distance learning,Data fusion,Bayesian inference,distributed machine learning,federated learning
AI 理解论文
溯源树
样例
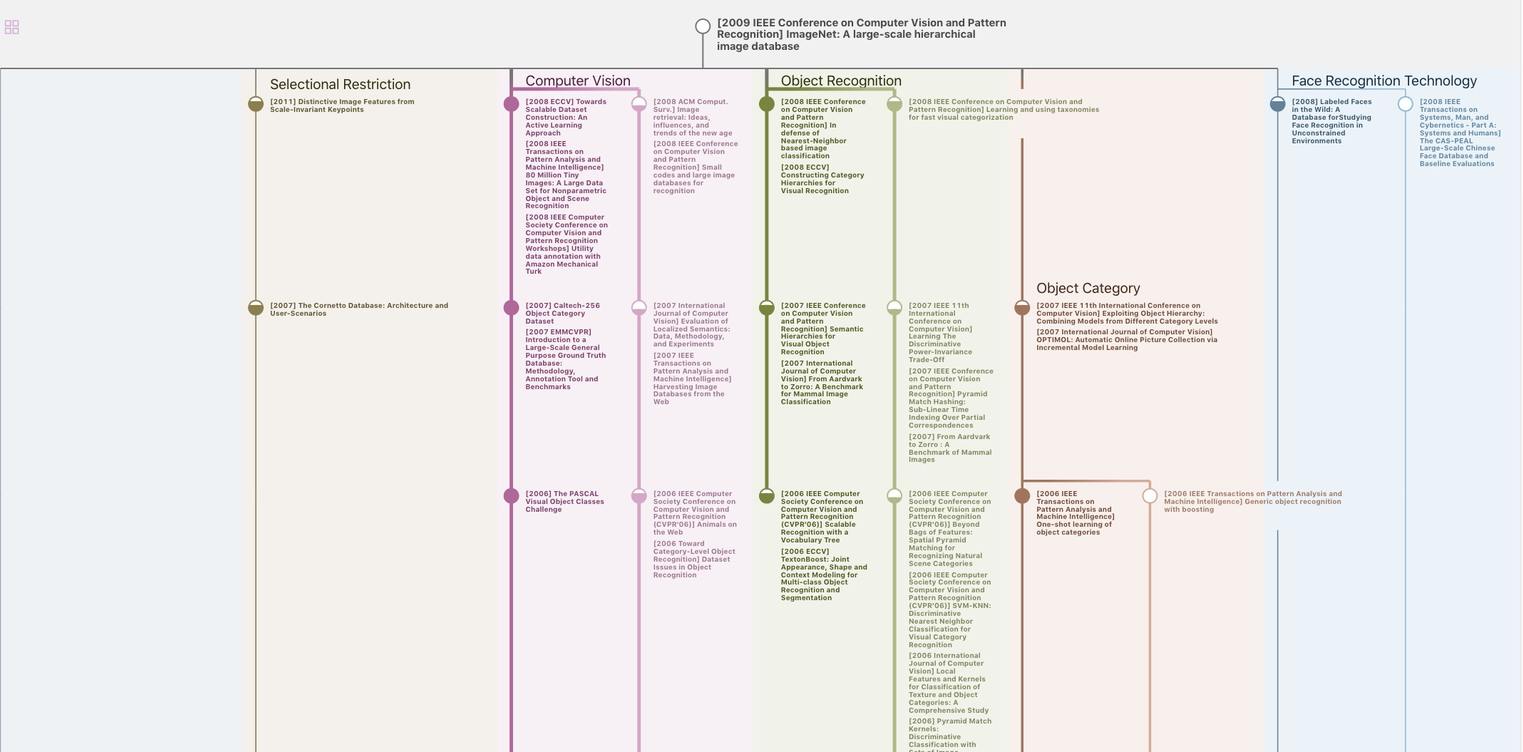
生成溯源树,研究论文发展脉络
Chat Paper
正在生成论文摘要