Machine Learning-Guided Computational Screening of New Candidate Reactions with High Bioorthogonal Click Potential.
arxiv(2023)
摘要
Bioorthogonal click chemistry has become an indispensable part of the biochemist's toolbox. Despite the wide variety of applications that have been developed in recent years, only a limited number of bioorthogonal click reactions have been discovered so far, most of them based on (substituted) azides. In this work, we present a computational workflow to discover new candidate reactions with promising kinetic and thermodynamic properties for bioorthogonal click applications. Sampling only around 0.05 % of an overall search space of over 10,000,000 dipolar cycloadditions, we develop a machine learning model able to predict DFT-computed activation and reaction energies within ∼2-3 kcal/mol across the entire space. Applying this model to screen the full search space through iterative rounds of learning, we identify a broad pool of candidate reactions with rich structural diversity, which can be used as a starting point or source of inspiration for future experimental development of both azide-based and non-azide-based bioorthogonal click reactions.
更多查看译文
关键词
bioorthogonal click chemistry,deep learning,density functional theory calculations,machine learning,reactivity
AI 理解论文
溯源树
样例
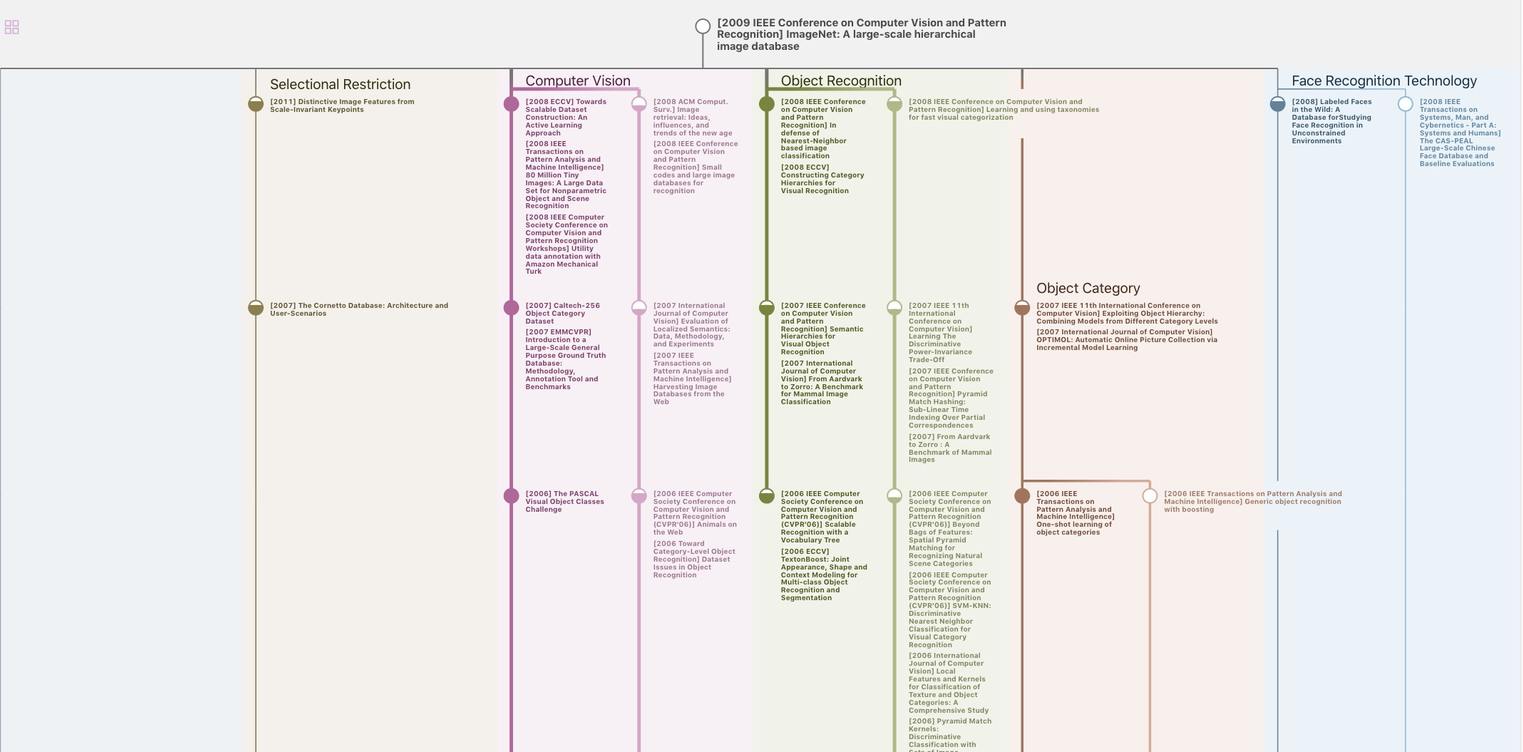
生成溯源树,研究论文发展脉络
Chat Paper
正在生成论文摘要