Deep learning-based reduced-order methods for fast transient dynamics
arxiv(2022)
摘要
In recent years, large-scale numerical simulations played an essential role
in estimating the effects of explosion events in urban environments, for the
purpose of ensuring the security and safety of cities. Such simulations are
computationally expensive and, often, the time taken for one single computation
is large and does not permit parametric studies. The aim of this work is
therefore to facilitate real-time and multi-query calculations by employing a
non-intrusive Reduced Order Method (ROM). We propose a deep learning-based (DL)
ROM scheme able to deal with fast transient dynamics. In the case of blast
waves, the parametrised PDEs are time-dependent and non-linear. For such
problems, the Proper Orthogonal Decomposition (POD), which relies on a linear
superposition of modes, cannot approximate the solutions efficiently. The
piecewise POD-DL scheme developed here is a local ROM based on time-domain
partitioning and a first dimensionality reduction obtained through the POD.
Autoencoders are used as a second and non-linear dimensionality reduction. The
latent space obtained is then reconstructed from the time and parameter space
through deep forward neural networks. The proposed scheme is applied to an
example consisting of a blast wave propagating in air and impacting on the
outside of a building. The efficiency of the deep learning-based ROM in
approximating the time-dependent pressure field is shown.
更多查看译文
AI 理解论文
溯源树
样例
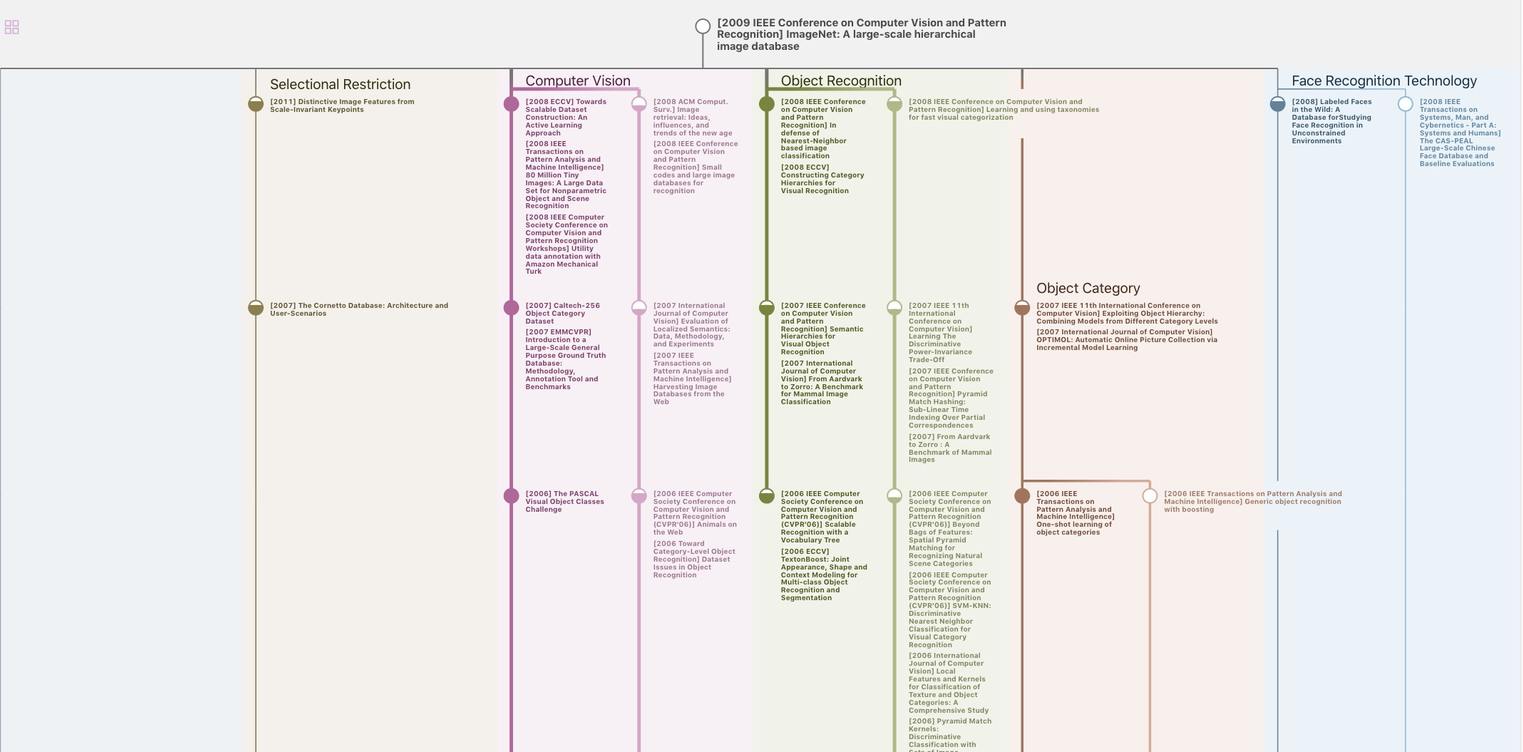
生成溯源树,研究论文发展脉络
Chat Paper
正在生成论文摘要