Enriched entity representation of knowledge graph for text generation
COMPLEX & INTELLIGENT SYSTEMS(2022)
摘要
Text generation is a key tool in natural language applications. Generating texts which could express rich ideas through several sentences needs a structured representation of their content. Many works utilize graph-based methods for graph-to-text generation, like knowledge-graph-to-text generation. However, generating texts from knowledge graph still faces problems, such as repetitions and the entity information is not fully utilized in the generated text. In this paper, we focus on knowledge-graph-to-text generation, and develop a multi-level entity fusion representation (MEFR) model to address the above problems, aiming to generate high-quality text from knowledge graph. Our model introduces a fusion mechanism, which is capable of aggregating node representations from word level and phrase level to obtain rich entity representations of the knowledge graph. Then, Graph Transformer is adopted to encode the graph and outputs contextualized node representations. Besides, we develop a vanilla beam search-based comparison mechanism during decoding procedure, which further considers similarity to reduce repetitive information of the generated text. Experimental results show that the proposed MEFR model could effectively improve generation performance, and outperform other baselines on AGENDA and WebNLG datasets. The results also demonstrate the importance to further explore information contained in knowledge graph.
更多查看译文
关键词
Text generation,Knowledge graph,Multi-level entity fusion representation model,Comparison mechanism
AI 理解论文
溯源树
样例
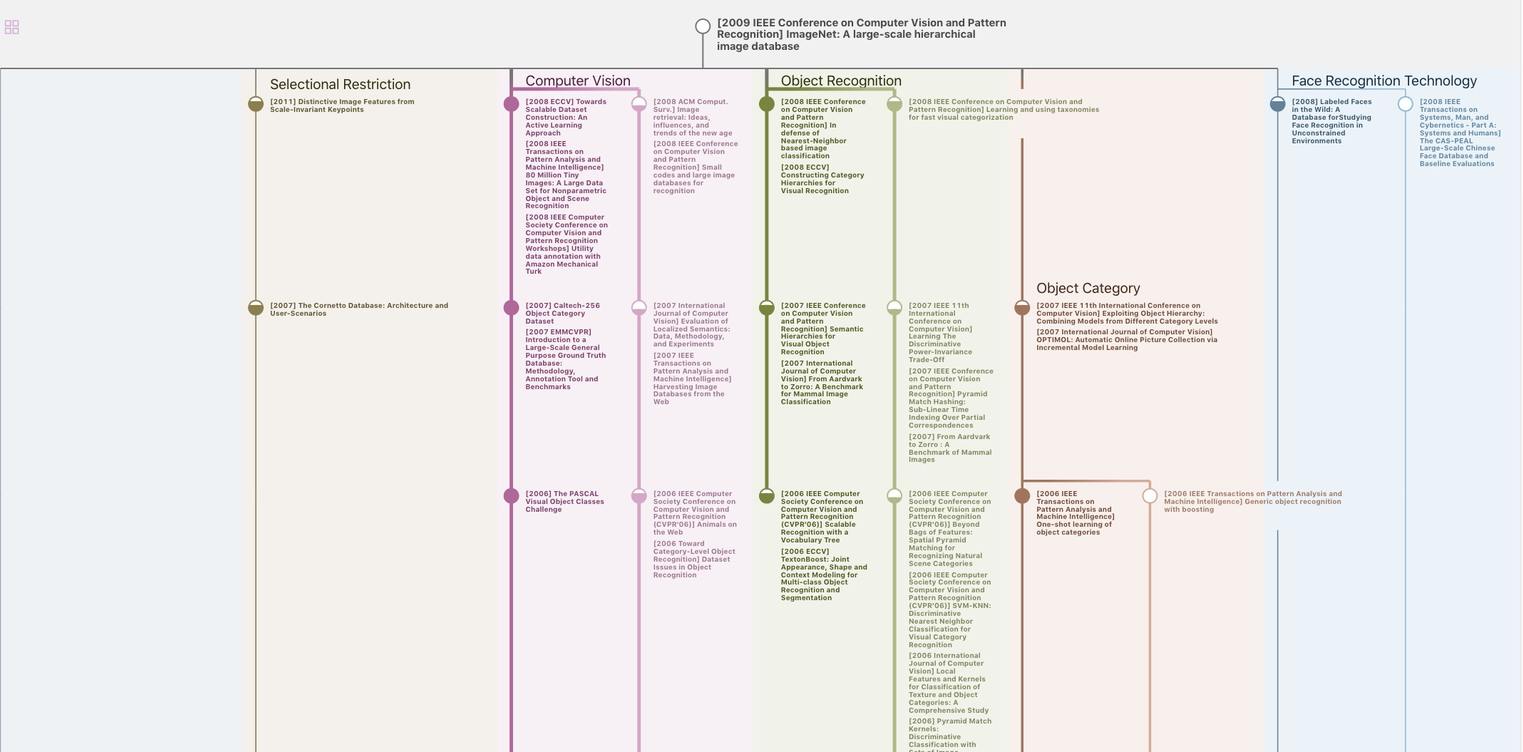
生成溯源树,研究论文发展脉络
Chat Paper
正在生成论文摘要