Visual analytics of potential dropout behavior patterns in online learning based on counterfactual explanation
Journal of Visualization(2022)
摘要
Online learning is gradually becoming a popular way of learning due to the high flexibility in time and space. Reducing the high dropout rate is important to promote the further development of smart education. However, learners’ learning is a dynamic temporal process, which is influenced by multiple factors synergistically. How to identify the key influencing factors of dropout in an interpretable way is still a challenging problem. In this paper, we propose a pattern identification method of dropout behavior, including the prediction of the dropout probability and the mining of potential impact factors, to gain a comprehensive insight into the dropout behavior hidden in the data. A CNN-LSTM model for dropout prediction is constructed, which can automatically extract features and learn the temporal dependence of dropout behavior. By introducing the counterfactual explanation, the dropout impacts of different learning behavior can be revealed quantitatively. Moreover, we design and develop an interactive visual analytics system, DropoutVis, for exploring learning behavior, extracting the various dropout patterns and providing a basis for formulating strategies. The effectiveness and usefulness of DropoutVis have been demonstrated through case studies with a real dataset. Graphical abstract
更多查看译文
关键词
Visual analytics,Dropout pattern,Counterfactual explanation
AI 理解论文
溯源树
样例
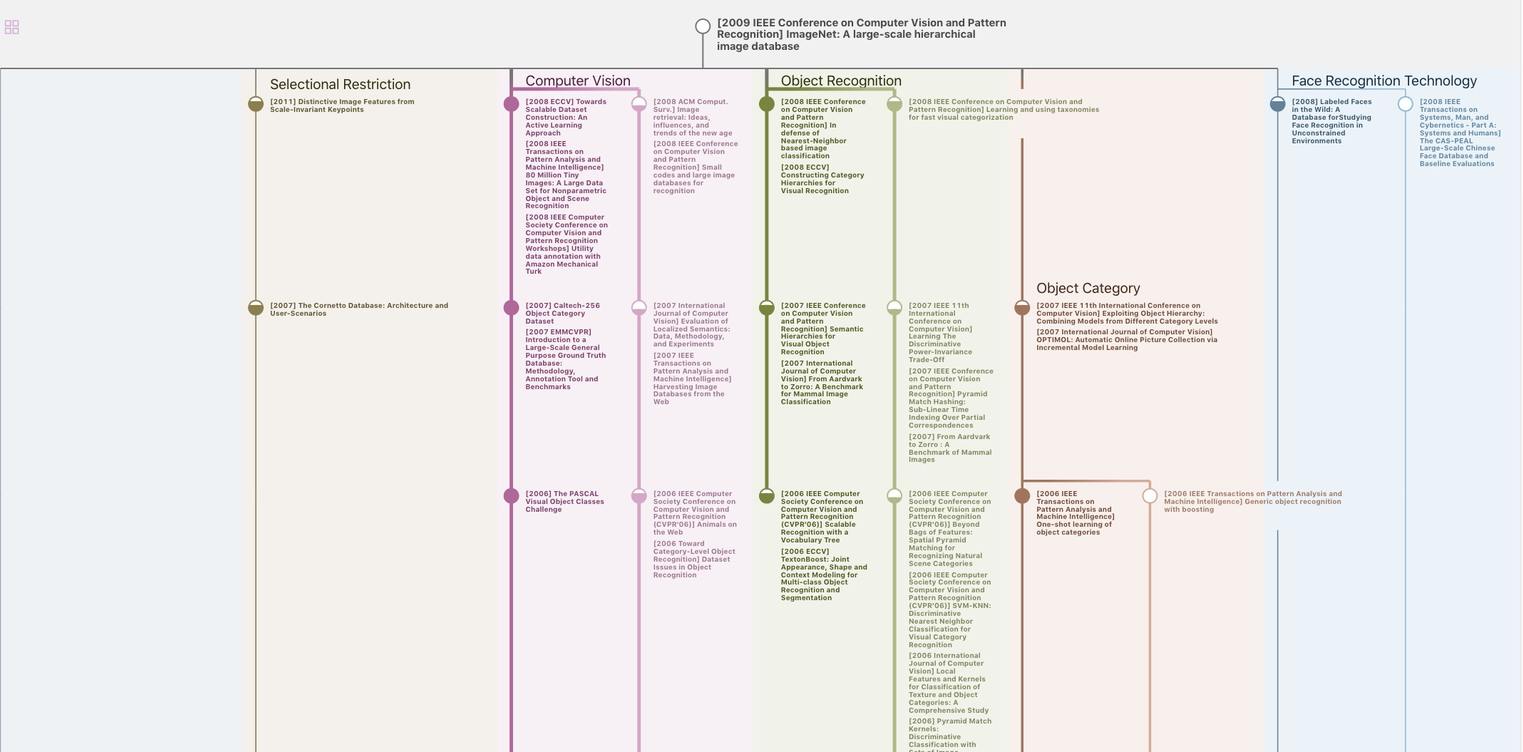
生成溯源树,研究论文发展脉络
Chat Paper
正在生成论文摘要