Enhancing Sentiment Analysis via Random Majority Under-Sampling with Reduced Time Complexity for Classifying Tweet Reviews
ELECTRONICS(2022)
摘要
Twitter has become a unique platform for social interaction from people all around the world, leading to an extensive amount of knowledge that can be used for various reasons. People share and spread their own ideologies and point of views on unique topics leading to the production of a lot of content. Sentiment analysis is of extreme importance to various businesses as it can directly impact their important decisions. Several challenges related to the research subject of sentiment analysis includes issues such as imbalanced dataset, lexical uniqueness, and processing time complexity. Most machine learning models are sequential: they need a considerable amount of time to complete execution. Therefore, we propose a model sentiment analysis specifically designed for imbalanced datasets that can reduce the time complexity of the task by using various text sequenced preprocessing techniques combined with random majority under-sampling. Our proposed model provides competitive results to other models while simultaneously reducing the time complexity for sentiment analysis. The results obtained after the experimentation corroborate that our model provides great results producing the accuracy of 86.5% and F1 score of 0.874 through XGB.
更多查看译文
关键词
sentiment analysis-SA, sentiment classification-SC, resampling, random minority oversampling, random majority under-sampling, machine learning-ML
AI 理解论文
溯源树
样例
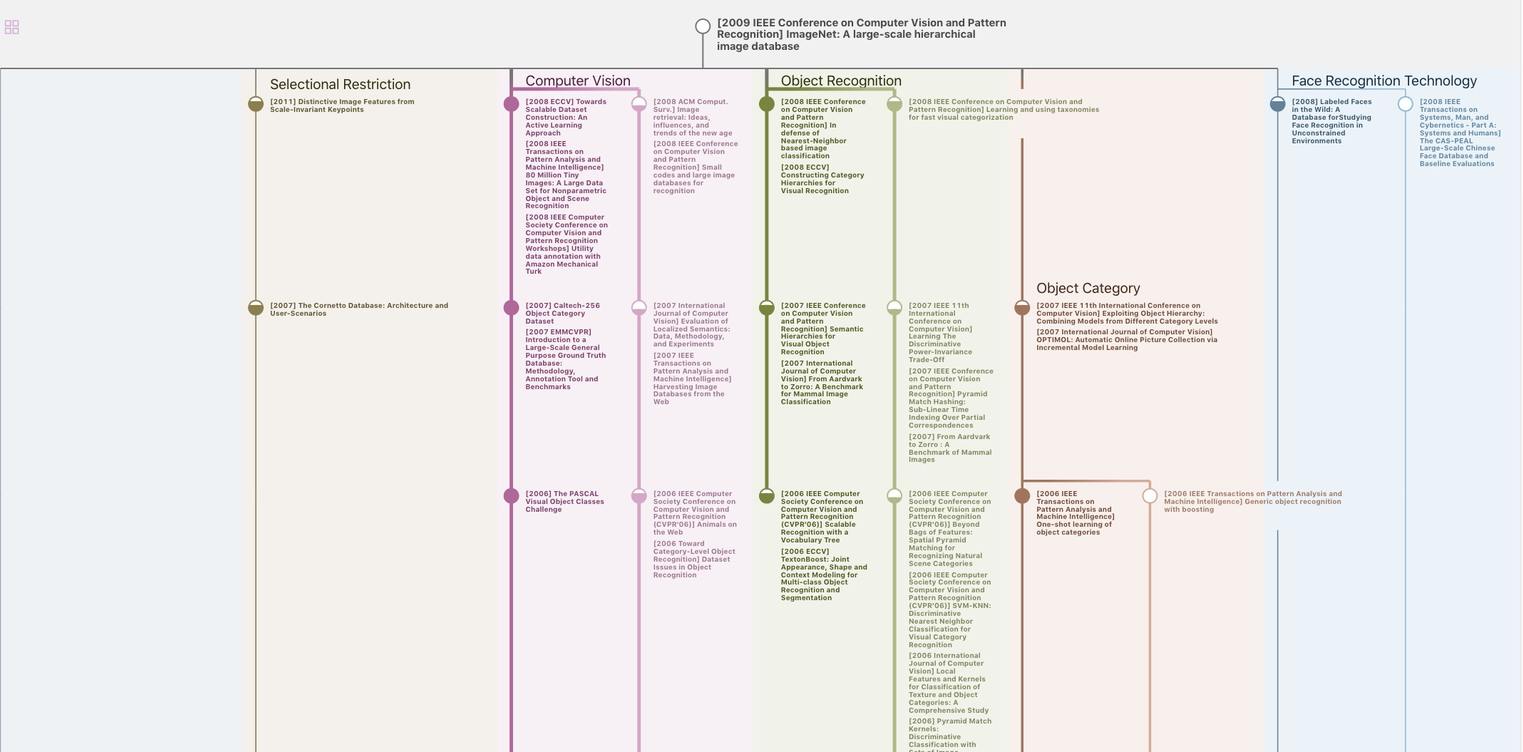
生成溯源树,研究论文发展脉络
Chat Paper
正在生成论文摘要