Performance Investigation of Principal Component Analysis for Intrusion Detection System Using Different Support Vector Machine Kernels
ELECTRONICS(2022)
摘要
The growing number of security threats has prompted the use of a variety of security techniques. The most common security tools for identifying and tracking intruders across diverse network domains are intrusion detection systems. Machine Learning classifiers have begun to be used in the detection of threats, thus increasing the intrusion detection systems' performance. In this paper, the investigation model for an intrusion detection systems model based on the Principal Component Analysis feature selection technique and a different Support Vector Machine kernels classifier is present. The impact of various kernel functions used in Support Vector Machines, namely linear, polynomial, Gaussian radial basis function, and Sigmoid, is investigated. The performance of the investigation model is measured in terms of detection accuracy, True Positive, True Negative, Precision, Sensitivity, and F-measure to choose an appropriate kernel function for the Support Vector Machine. The investigation model was examined and evaluated using the KDD Cup'99 and UNSW-NB15 datasets. The obtained results prove that the Gaussian radial basis function kernel is superior to the linear, polynomial, and sigmoid kernels in both used datasets. Obtained accuracy, Sensitivity, and, F-measure of the Gaussian radial basis function kernel for KDD CUP'99 were 99.11%, 98.97%, and 99.03%. for UNSW-NB15 datasets were 93.94%, 93.23%, and 94.44%.
更多查看译文
关键词
intrusion detection system, support vector machine, network security, KDD Cup'99 datasets, UNSW-NB15 datasets, principal component analysis
AI 理解论文
溯源树
样例
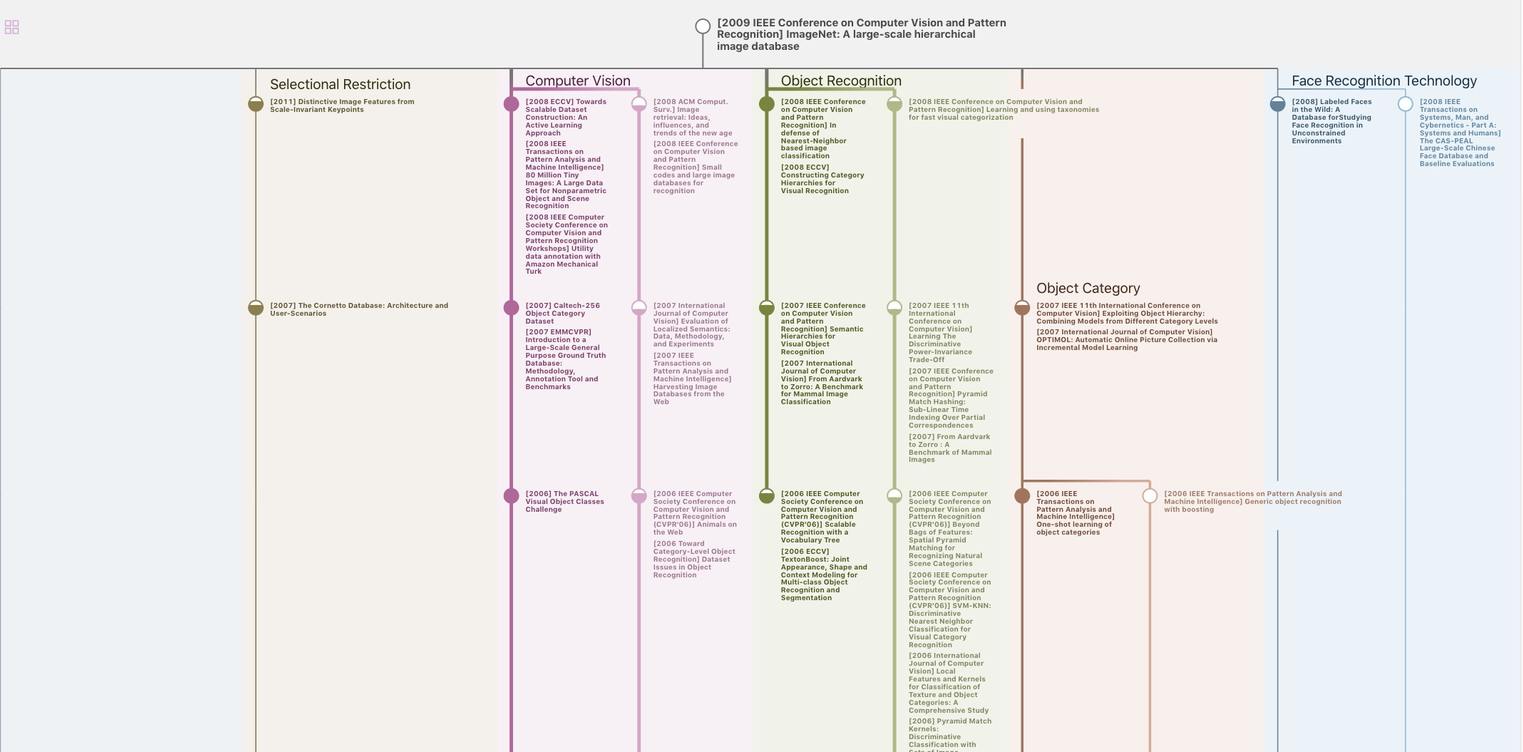
生成溯源树,研究论文发展脉络
Chat Paper
正在生成论文摘要