Event-guided Multi-patch Network with Self-supervision for Non-uniform Motion Deblurring
INTERNATIONAL JOURNAL OF COMPUTER VISION(2022)
摘要
Contemporary deep learning multi-scale deblurring models suffer from many issues: (I) They perform poorly on non-uniformly blurred images/videos; (II) Simply increasing the model depth with finer-scale levels cannot improve deblurring; (III) Individual RGB frames contain a limited motion information for deblurring; (IV) Previous models have a limited robustness to spatial transformations and noise. Below, we propose several mechanisms based on the multi-patch network to address the above issues: (I) We present a novel self-supervised event-guided deep hierarchical Multi-patch Network (MPN) to deal with blurry images and videos via fine-to-coarse hierarchical localized representations; (II) We propose a novel stacked pipeline, StackMPN, to improve the deblurring performance under the increased network depth; (III) We propose an event-guided architecture to exploit motion cues contained in videos to tackle complex blur in videos; (IV) We propose a novel self-supervised step to expose the model to random transformations (rotations, scale changes), and make it robust to Gaussian noises. Our MPN achieves the state of the art on the GoPro and VideoDeblur datasets with a 40 × faster runtime compared to current multi-scale methods. With 30 ms to process an image at 1280 × 720 resolution, it is the first real-time deep motion deblurring model for 720p images at 30 fps. For StackMPN, we obtain significant improvements over 1.2 dB on the GoPro dataset by increasing the network depth. Utilizing the event information and self-supervision further boost results to 33.83 dB.
更多查看译文
关键词
Motion deblurring, Deep learning, Multi-patch, Event camera, Self-supervision
AI 理解论文
溯源树
样例
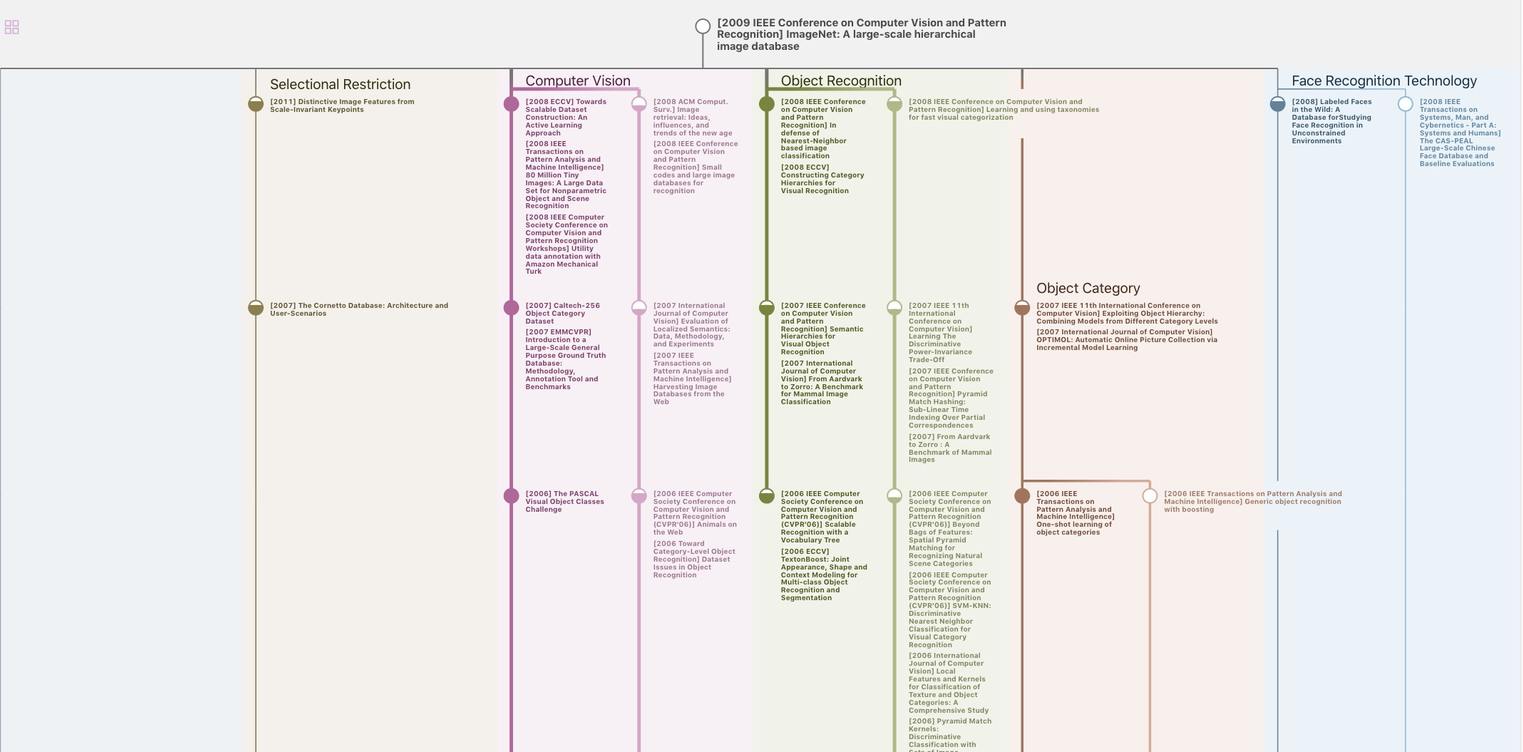
生成溯源树,研究论文发展脉络
Chat Paper
正在生成论文摘要