Detecting Cheating in Large-Scale Assessment: The Transfer of Detectors to New Tests
EDUCATIONAL AND PSYCHOLOGICAL MEASUREMENT(2023)
摘要
Recent approaches to the detection of cheaters in tests employ detectors from the field of machine learning. Detectors based on supervised learning algorithms achieve high accuracy but require labeled data sets with identified cheaters for training. Labeled data sets are usually not available at an early stage of the assessment period. In this article, we discuss the approach of adapting a detector that was trained previously with a labeled training data set to a new unlabeled data set. The training and the new data set may contain data from different tests. The adaptation of detectors to new data or tasks is denominated as transfer learning in the field of machine learning. We first discuss the conditions under which a detector of cheating can be transferred. We then investigate whether the conditions are met in a real data set. We finally evaluate the benefits of transferring a detector of cheating. We find that a transferred detector has higher accuracy than an unsupervised detector of cheating. A naive transfer that consists of a simple reuse of the detector increases the accuracy considerably. A transfer via a self-labeling (SETRED) algorithm increases the accuracy slightly more than the naive transfer. The findings suggest that the detection of cheating might be improved by using existing detectors of cheating at an early stage of an assessment period.
更多查看译文
关键词
cheating,data mining,transfer learning
AI 理解论文
溯源树
样例
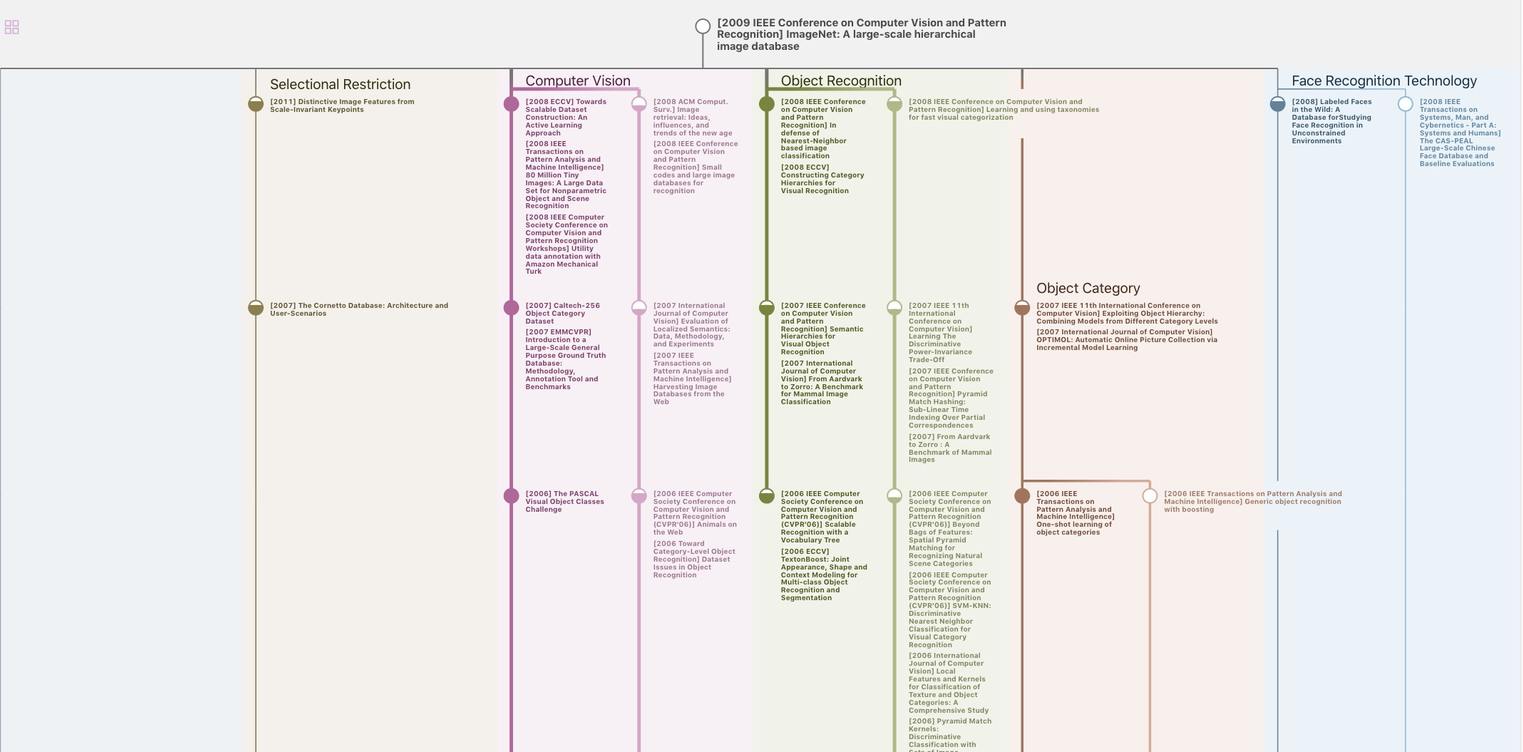
生成溯源树,研究论文发展脉络
Chat Paper
正在生成论文摘要