Multi-Conditional Optimization of a High-Specific-Speed Axial Flow Pump Impeller Based on Machine Learning
MACHINES(2022)
摘要
In order to widen the range of high-efficiency area of a high-specific-speed axial flow pump and to improve the operating efficiency under non-design conditions, the parameters of the axial flow pump blades were optimized. An optimization system based on computational fluid dynamics (CFD), optimized Latin hypercube sampling (OLHS), machine learning (ML), and multi-island genetic algorithm (MIGA) was established. The prediction effects of three machine learning models based on Bayesian optimization, support vector machine regression (SVR), Gaussian process regression (GPR), and fully connected neural network (FNN) on the performance of the axial flow pump were compared. The results show that the GPR model has the highest prediction accuracy for the impeller head and weighted efficiency. Compared to the original impeller, the optimized impeller is forward skewed and backward swept, and the weighted efficiency of the impeller increases by 1.31 percentage points. The efficiency of the pump section at 0.8Q(d), 1.0Q(d), and 1.2Q(d) increases by about 1.1, 1.4, and 1.6 percentage points, respectively, which meets the optimization requirements. After optimization, the internal flow field of the impeller is more stable; the entropy production in the impeller reduces; the spanwise distribution of the total pressure coefficient and the axial velocity coefficient at the impeller outlet are more uniform; and the flow separation near the hub at the blade trailing edge is restrained. This research can provide a reference for the efficient operation of pumping stations and the optimal design of axial flow pumps under multiple working conditions.
更多查看译文
关键词
axial flow pump,multi-objective optimization,machine learning,skew and sweep
AI 理解论文
溯源树
样例
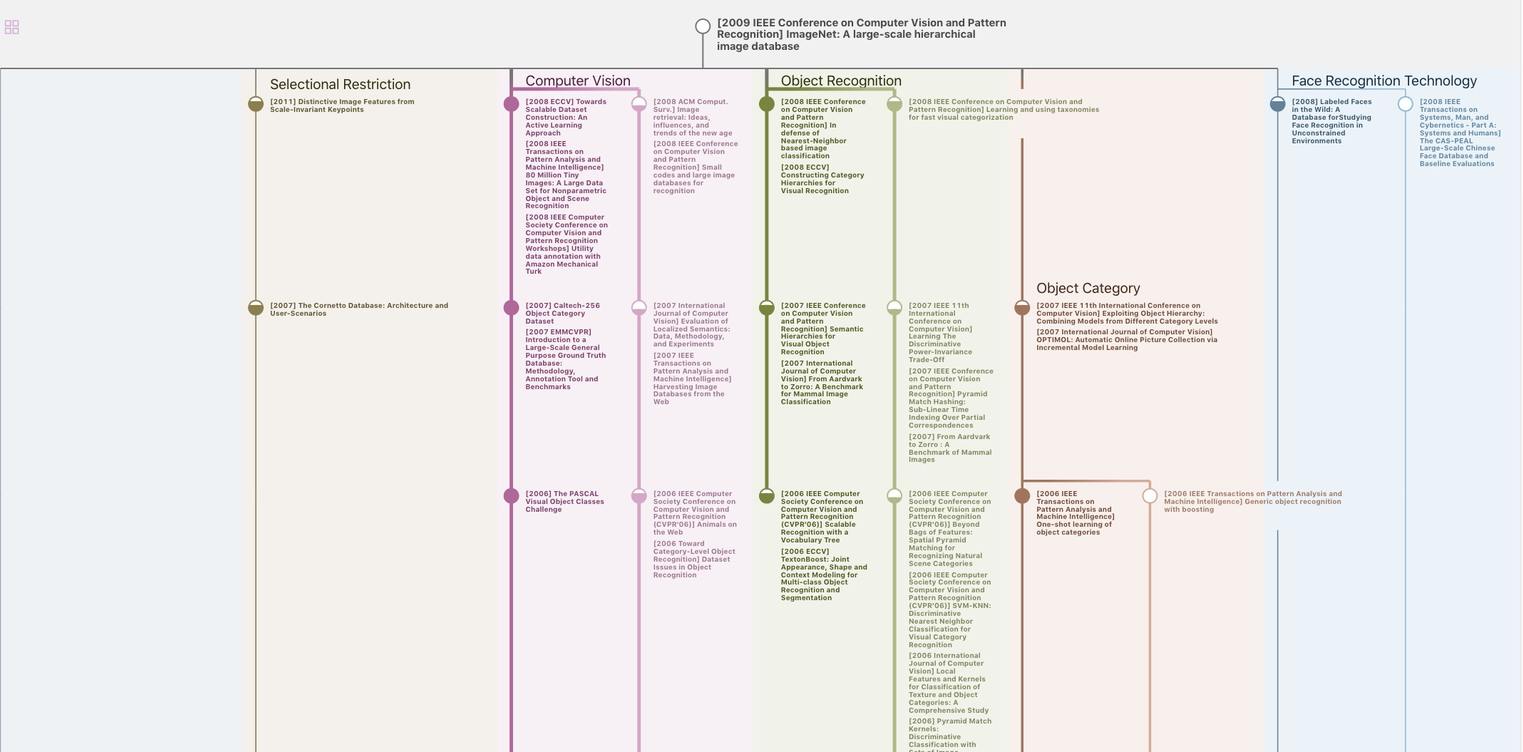
生成溯源树,研究论文发展脉络
Chat Paper
正在生成论文摘要