Special Issue on Sparsity-inducing Methods in Control Theory
INTERNATIONAL JOURNAL OF ROBUST AND NONLINEAR CONTROL(2023)
摘要
Modern control theory is challenged by an increasing complexity of the systems to be controlled and meanwhile by the increasing level of demanded performance. In effect, the control performance specification comes with various other design requirements related to energy consumption, communication network topology, computational efficiency, fault-tolerance, robustness/resilience and diverse hard constraints with respect to size, cost, compliance to norms, etc. It can be observed that a significant number of performance specifications admit a mathematical formalization which involves implicitly or explicitly some structural sparsity requirements (e.g., design of network topology with minimum communication links, estimation in the presence of sporadic data losses, impulsive noise-tolerant control, identification of switched systems, etc). Furthermore, sparsity constraints can arise as a mere methodological device induced by the intrinsic nature of the control engineering problem, as well as from sparse uncertainties which can take the form of parametric inaccuracies in the system model or sparse disturbances affecting the measurements and the control signals. For example, a large-scale control system, such as a chemical plant or a power grid, could be subject to a myriad of failures, but it is very rare that a large number of faults occur simultaneously. Meanwhile, sparsity constraints can be leveraged to model critical situations where the sensory and control channels are under an integrity attack from a resource-constrained adversary, who can only break the encryption on a small subset of the signals. In all these situations, the structural sparsity, whether induced by intermittent errors, spatially distributed faults or adversary attacks, can usually be represented by additive sparse or low-rank matrices. From a methodological viewpoint, sparsity-inducing optimization is a powerful paradigm for developing control and estimation schemes that are robust to the aforementioned sparse uncertainties. Sparse optimization refers to a mathematical optimization framework in which the to-be-optimized objective function or the associated constraints involve an sparsity measure on the data such as the rank of a matrix, the “zero-norm” of a vector or the cardinality of a set. Although most of the sparse optimization problems are combinatorial in nature, recent advancements, such as ℓ 1 $$ {\ell}_1 $$ relaxation and basis pursuit, have greatly reduced the computational complexity of sparse optimization for many practical problems. For example, compressed sensing has leveraged sparsity to bring a remarkable methodological shift in the way of acquiring, representing and processing information-bearing signals. Even though sparse optimization has been initially investigated in the communities of signal processing, information theory and machine learning, it has now been successfully applied to many other fields including the field of control systems design. For example, sparsity-inducing optimization has proven to be a powerful principle for developing resilient estimation and control algorithms that can withstand random system failures and malicious attacks. The recent literature shows that the application scope of sparsity-inducing methods in control theory covers diverse topics such as secure state estimation for cyber-physical systems, hybrid system identification and control, diagnosis, analysis and estimation of impulsive systems, control/estimation of switched systems, maximum hands-off control, sensor scheduling, control allocation, network connectivity design/estimation, etc. This special issue gathers a sample of new developments in the theory and practice of estimation and control methods which rely on sparsity-promoting optimization. It collects a representative body of innovative contributions in diverse aspects of this methodology, hence expecting to give a complete picture of the current trend and state of the art. The Special Issue contains 12 papers which cover various topics such as control design, state estimation, system identification and data allocation. Most of the contributions of this Special Issue present recent methodological developments in control design techniques involving an implicit or explicit sparsification constraint. The sparse constraint is imposed either on the desired control signal1-3 or on the structure of the to-be-designed controller.4, 5 Both continuous-time and discrete-time systems are considered. More specifically, Ref.1 provides an excellent survey on sparse control design methods for continuous-time systems. Ref.2 considers the problem of designing a group-sparse control called maximum turn-off control for multi-inputs discrete-time systems. The authors propose a solution based on the resolution of a constrained convex optimization on a finite time-horizon. Ref.3 considers the sparse control design problem for a class of nonlinear systems. The method consists in optimizing a sparsity-inducing objective function under stability constraints. Ref.4 utilizes block-sparse optimization as a tool for the design of distributed controllers.5 designs decentralized controllers for linear time-varying systems under structural-sparsity constraints. In a similar setting, Ref.6 proposes a state estimator along with a decentralized control. Refs7 and8 use the event-triggered principle as a way to achieve sparsity in the desired control signal for linear systems and multi-agent systems respectively. Another important problem considered in this Special Issue is that of state estimation in the face of impulsive noise or sparse adversarial attacks. In this context, Ref.9 surveys the application of sparsity-inducing methods in robust regression, hybrid system identification and secure state estimation. Ref.10 considers specifically the secure state estimation problem and derives an efficient estimator in the presence of sparse integrity attacks. In11 the authors consider the problem of optimizing the data rate allocation for dynamic sensor fusion under resource-constrained communication networks. The so-obtained solution turns out to be sparse. Finally, the work presented in Ref.12 proposes a multiple importance unscented Kalman filtering with soft spatio-temporal constraint for multi-passive-sensor target tracking. We would like to thank all the authors and the anonymous reviewers who contributed to this Special Issue. We are also grateful to the Editor-in-Chief and Editorial Staff for their support to this Special Issue.
更多查看译文
关键词
control theory
AI 理解论文
溯源树
样例
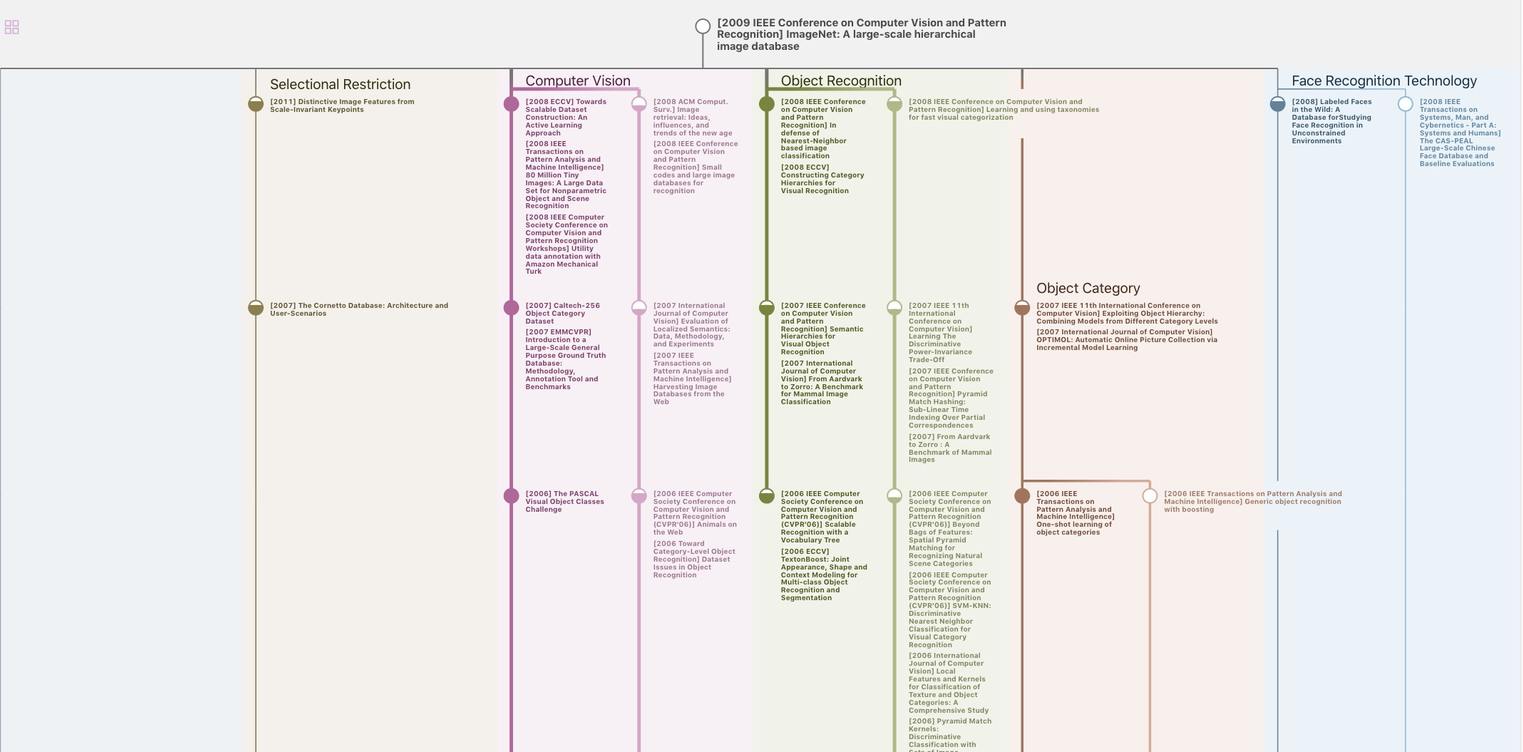
生成溯源树,研究论文发展脉络
Chat Paper
正在生成论文摘要