Reconstruction of long-term sea-level data gaps of tide gauge records using a neural network operator
FRONTIERS IN MARINE SCIENCE(2022)
摘要
The coastal sea level is an important factor in understanding and clarifying the physical processes in coastal seas. However, missing values and outliers of the sea level that occur for various reasons often disrupt the continuity of its time series. General-purpose time-series analysis and prediction methods are not tolerant of missing values, which is why researchers have attempted to fill these gaps. The disadvantage of conventional time-series reconstruction techniques is the low accuracy when missed sea-level records are longer than the timescales of coastal processes. To solve this problem, we used an artificial neural network, which is a novel tool for creating multivariate and nonlinear regression equations. The trained neural network weight set was designed to enable long-term reconstruction of sea level by acting as a one-step prediction operator. In addition, a data assimilation technique was developed and adapted to ensure seamless continuity between predicted and observed sea-level records. The application of our newly developed method to 3-day gaps of seal level records at 16 tide gauge stations around the Korean peninsula confirms that it can successfully reconstruct missing values with root-mean-squared errors of 0.5-1.1 cm on average.
更多查看译文
关键词
data reconstruction, data gap-filling, neural network, long short-term memory (LSTM), coastal sea level
AI 理解论文
溯源树
样例
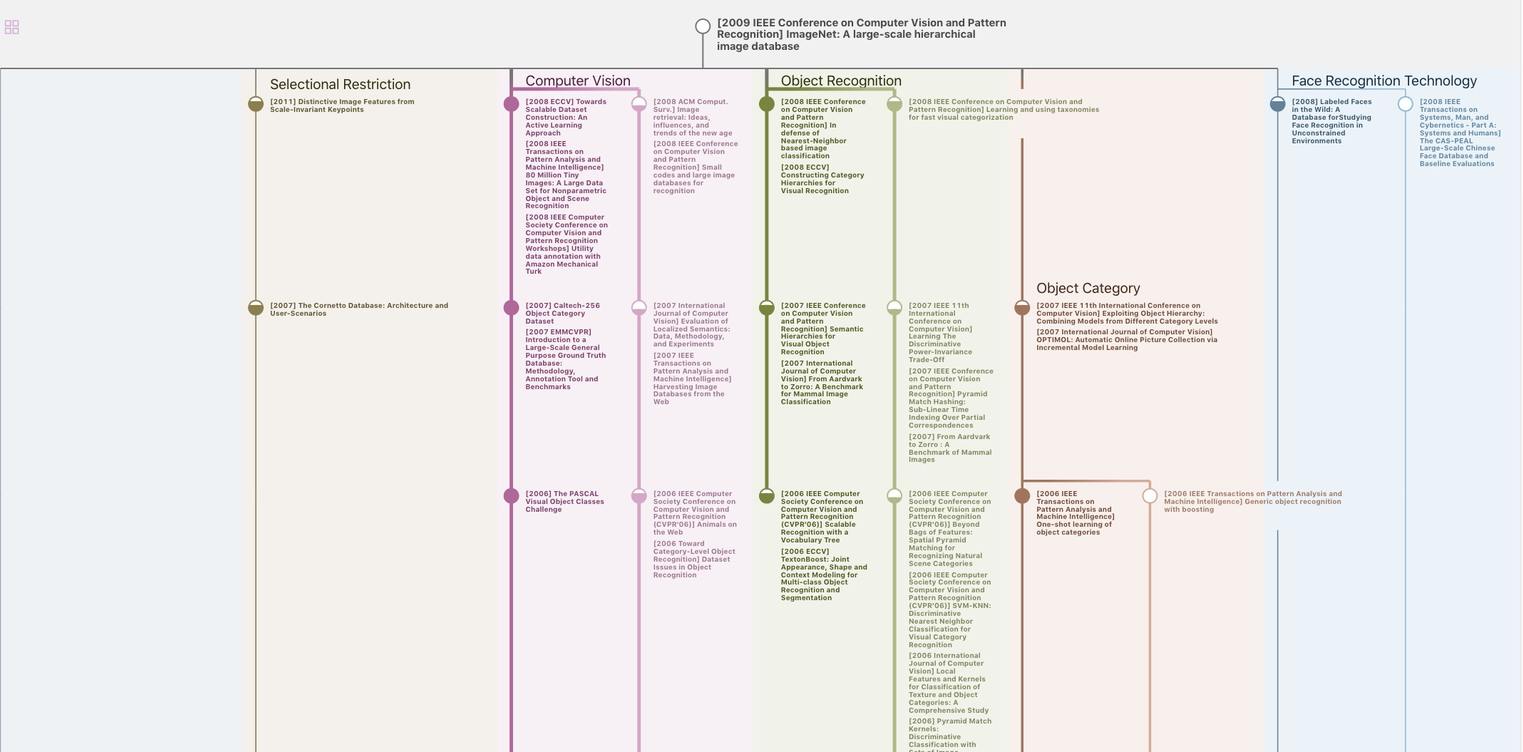
生成溯源树,研究论文发展脉络
Chat Paper
正在生成论文摘要