Predicting Hospital Admissions to Reduce Crowding in the Emergency Departments
Applied sciences(2022)
摘要
Having an increasing number of patients in the emergency department constitutes an obstacle to the admissions process and hinders the emergency department (ED)’s ability to deal with the continuously arriving demand for new admissions. In addition, forecasting is an important aid in many areas of hospital management, including elective surgery scheduling, bed management, and staff resourcing. Therefore, this paper aims to develop a precise prediction model for admissions in the Integral Healthcare System for Public Use in Catalonia. These models assist in reducing overcrowding in emergency rooms and improve the quality of care offered to patients. Data from 60 EDs were analyzed to determine the likelihood of hospital admission based on information readily available at the time of arrival in the ED. The first part of the study targeted the obtention of models with high accuracy and area under the curve (AUC), while the second part targeted the obtention of models with a sensitivity higher than 0.975 and analyzed the possible benefits that could come from the application of such models. From the 3,189,204 ED visits included in the study, 11.02% ended in admission to the hospital. The gradient boosting machine method was used to predict a binary outcome of either admission or discharge.
更多查看译文
关键词
digital health,machine learning,gradient boosting
AI 理解论文
溯源树
样例
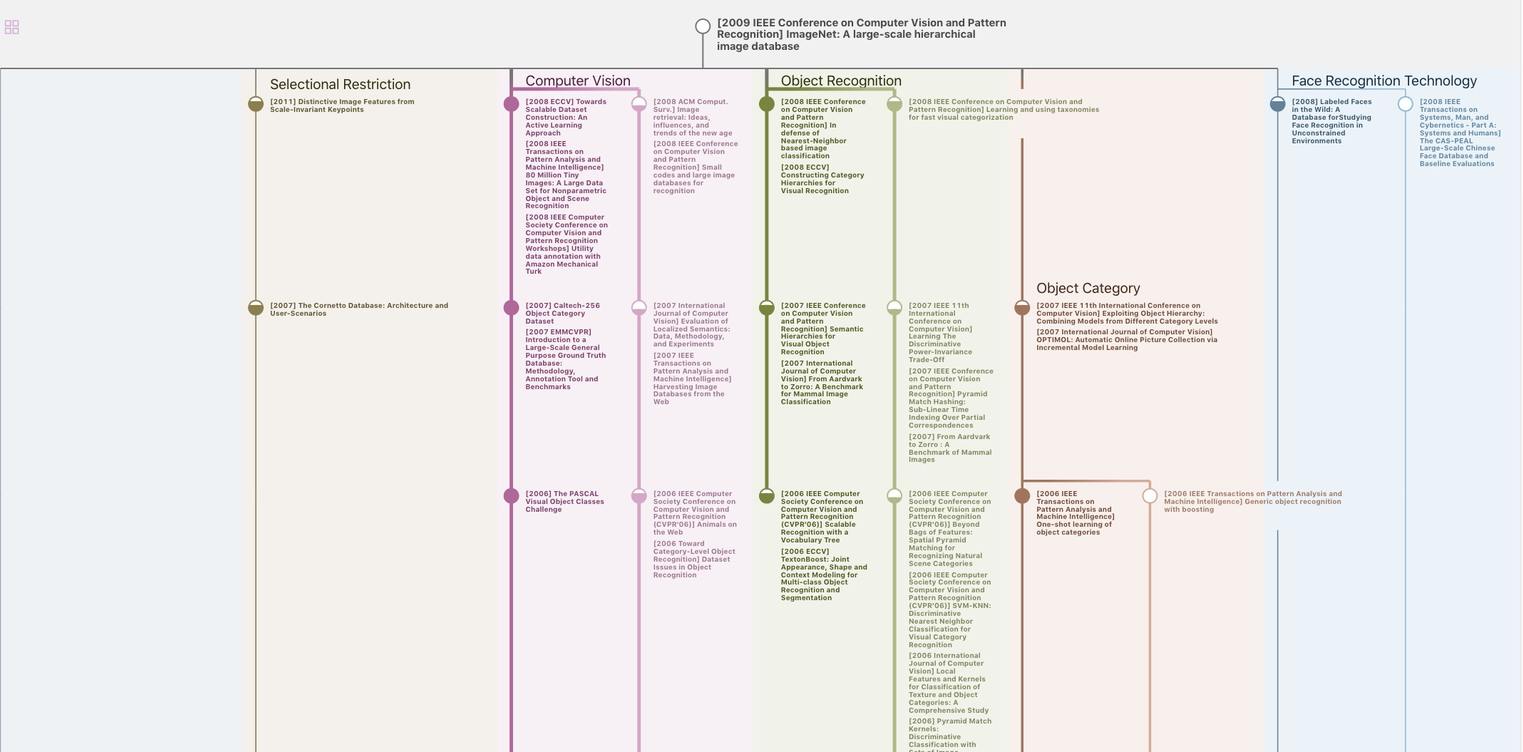
生成溯源树,研究论文发展脉络
Chat Paper
正在生成论文摘要