Category-Level Adversaries for Outdoor LiDAR Point Clouds Cross-Domain Semantic Segmentation
IEEE Transactions on Intelligent Transportation Systems(2023)
摘要
Unsupervised domain adaptation (UDA) is a low-cost way to deal with the lack of annotations in a new domain. For outdoor point clouds in urban transportation scenes, the mismatch of sampling patterns and the transferability difference between classes make cross-domain segmentation extremely difficult. To overcome these challenges, we propose a category-level adversarial framework. Firstly, we propose a multi-scale domain conditioned block that facilitates to extract the critical low-level domain-dependent knowledge and reduce the domain gap caused by distinct LiDAR sampling patterns. Secondly, we make full use of multiple representation forms (i.e., point-based sets and voxel-based cells) and utilize the prediction consistency between the two forms to measure how well each point is semantically aligned. The model then focuses on the poorly-aligned points without affecting the well-aligned points. Experimental results on three autonomous driving point cloud datasets show that the proposed method outperforms existing methods by a large margin, especially on the low-beam to high-beam cross-domain segmentation task.
更多查看译文
关键词
Unsupervised domain adaptation,point cloud,adversarial learning,domain conditioned feature extraction,semantic segmentation
AI 理解论文
溯源树
样例
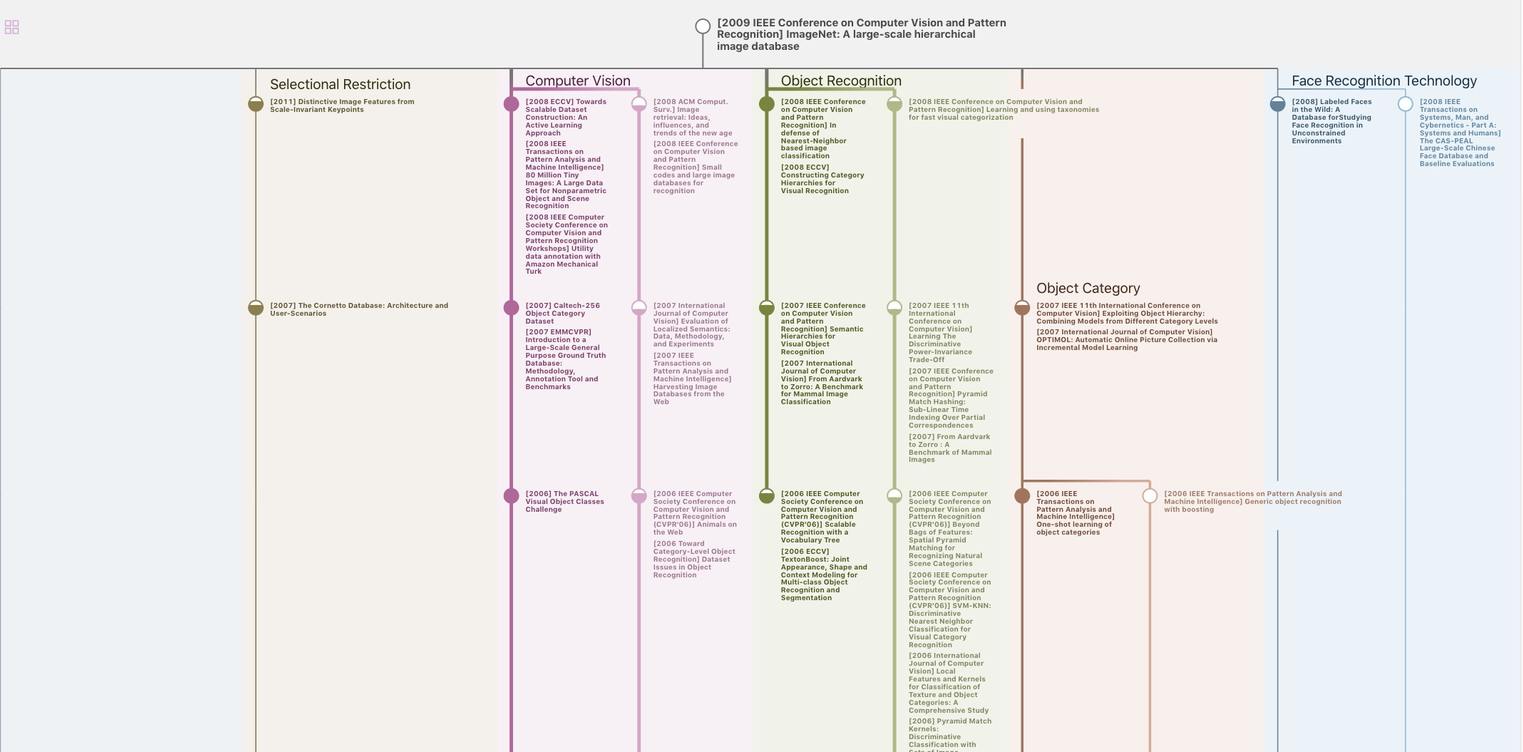
生成溯源树,研究论文发展脉络
Chat Paper
正在生成论文摘要