What Is Common to Transportation and Health in Machine Learning Education? The Domain Neglect Bias
IEEE TRANSACTIONS ON EDUCATION(2023)
摘要
Contribution: This article presents evidence that electrical engineering, computer science, and data science students, participating in introduction to machine learning (ML) courses, fail to interpret the performance of ML algorithms correctly, since they fail to consider the application domain. This phenomenon is referred to as the domain neglect bias and is discussed in this article from the cognitive psychology perspective. Background: ML algorithms are common components in electrical engineering, computer science, and data science curricula. Learning ML is challenging not only due to its complex mathematical and algorithmic aspects, but also due to: 1) the complexity of using these algorithms in the context of real-life situations correctly and 2) the comprehension of related social and ethical issues. Research Question: To what extent do students fail to correctly interpret the performance of ML algorithms due to the domain neglect bias and how can this bias be explained? Methodology: A questionnaire containing two questions was designed, in which the participants were asked to compare the performance of ML algorithms in the contexts of different application domains. The questions were designed to trigger the domain neglect bias which in turn, allowed quantification of its prevalence. Findings: One third of the students failed to recognize the outperforming ML algorithm due to the domain neglect bias. Educators should note this bias and develop new pedagogical methods for teaching ML performance in order to mitigate the bias.
更多查看译文
关键词
Machine learning algorithms, Data science, Prediction algorithms, Education, Machine learning, Computer science, Data models, Artificial intelligence, cognitive psychology, cognitive bias, domain neglect, machine learning
AI 理解论文
溯源树
样例
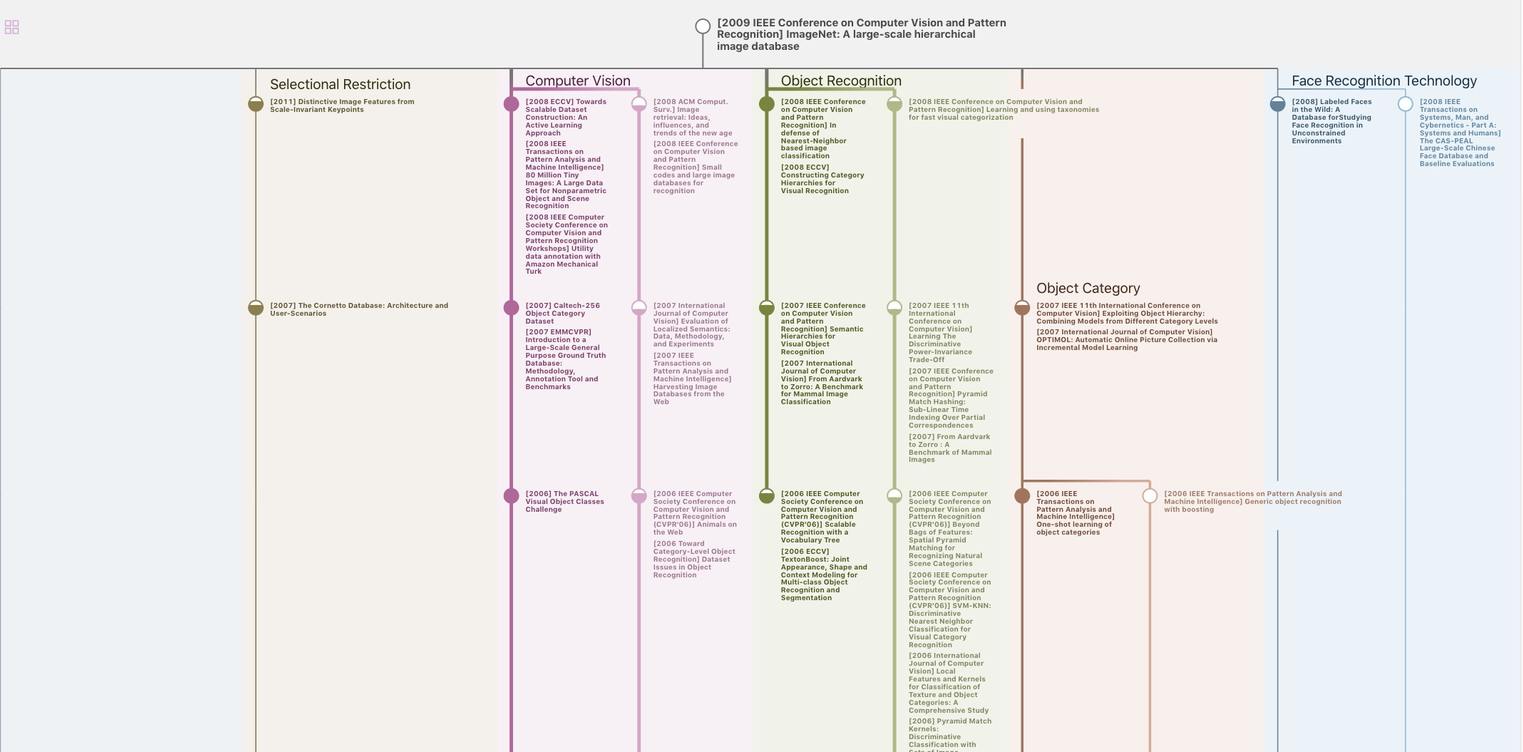
生成溯源树,研究论文发展脉络
Chat Paper
正在生成论文摘要