VP-KLNet: efficient 6D object pose estimation with an enhanced vector-field prediction network and a keypoint localization network
JOURNAL OF ELECTRONIC IMAGING(2022)
摘要
6D object pose estimation is essential for many applications with high demands in accuracy and speed. Compared with end-to-end approaches, pixel-wise voting network (PVNet), a vector-field based two-stage approach, has shown the superiority in accuracy but the inferiority in speed because of the time-consuming RANSAC-based voting strategy. To resolve this problem, we propose an efficient deep architecture that consists of an enhanced vector-field prediction network (VPNet) and a keypoint localization network (KLNet) and call it VP-KLNet. Specifically, the KLNet replaces PVNet's time-consuming voting scheme by directly regressing 2D keypoints from the vector fields, which significantly improves the inference speed. Furthermore, to capture multiscale contextual information, we embed the pyramid pooling module between the encoder and decoder in VPNet to obtain more accurate object segmentation and vector-field prediction, with negligible speed loss. Experiments demonstrate that our method has more than 50% improved in the running speed to the baseline method PVNet and achieves comparable accuracy with the state-of-the-art methods on the LINEMOD and occlusion LINEMOD datasets. (c) 2022 SPIE and IS&T
更多查看译文
关键词
6D object pose estimation, vector fields, keypoint localization, semantic segmentation, pyramid pooling module
AI 理解论文
溯源树
样例
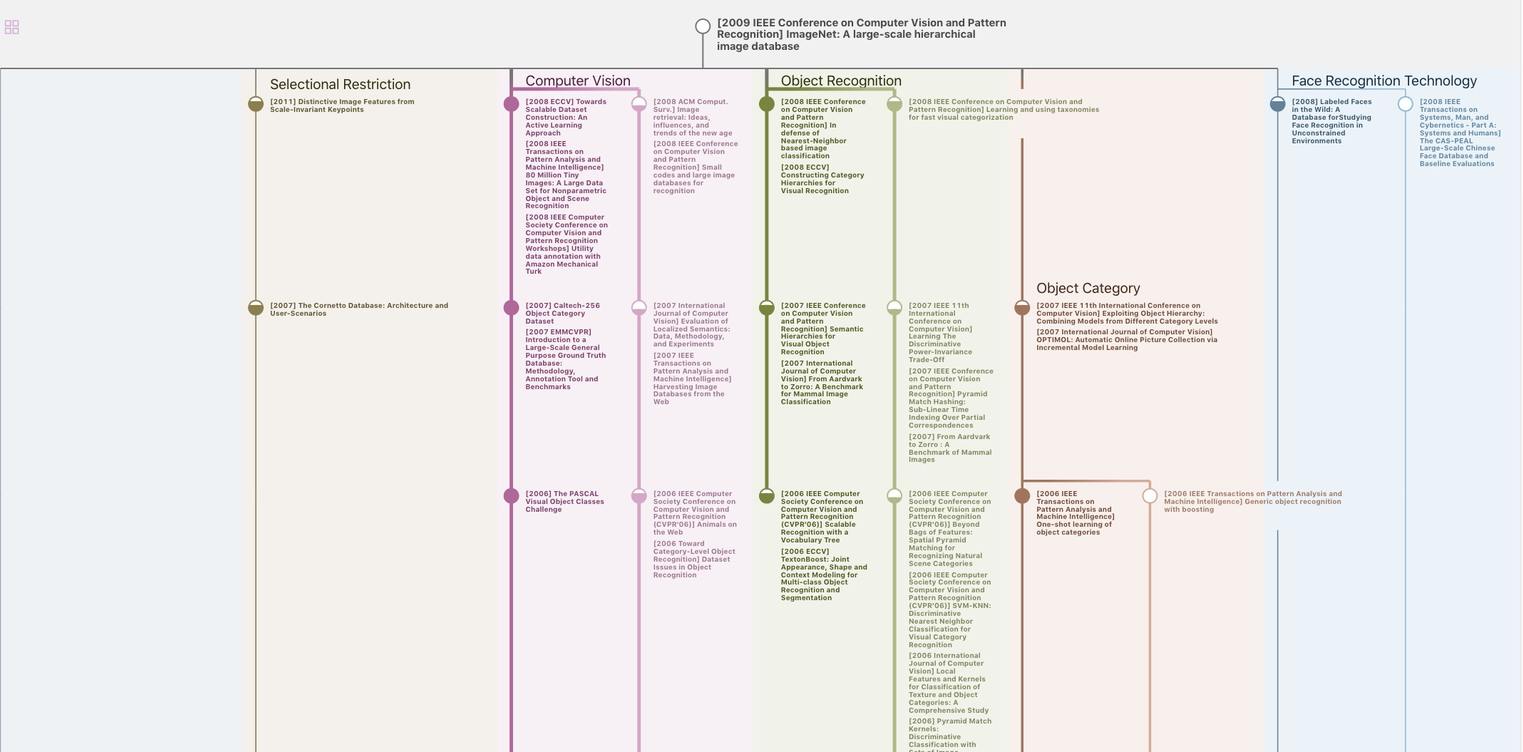
生成溯源树,研究论文发展脉络
Chat Paper
正在生成论文摘要