Design of Approximate Explicit Model Predictive Controller Using Parametric Optimization
Journal of mechanical design(2022)
摘要
This paper introduces a new technique, called state-parameterized nonlinear programming control (sp-NLPC), for designing a feedback controller that can stabilize intrinsically unstable nonlinear dynamical systems using parametric optimization. Stability-preserving constraints are included in the optimization problem solved offline by the predictive parameterized Pareto genetic algorithm (P3GA), a constrained nonlinear parametric optimization algorithm. The optimal control policy is approximated from P3GA output using radial basis function (RBF) metamodeling. The sp-NLPC technique requires fewer assumptions and is more data-efficient than alternative methods. Two nonlinear systems (single and double inverted pendulums on a cart) are used as benchmarking problems. Performance and computational efficiency are compared to several competing control design techniques. Results show that sp-NLPC outperforms and is more efficient than competing methods. A qualitative investigation on phase plane analysis for the controlled systems is presented to ensure stability. The approximating state-dependent solution for the control input lends itself to applications of control design for control co-design (CCD). Such extensions are discussed as part of future work.
更多查看译文
关键词
design optimization,explicit model predictive control,parametric optimization
AI 理解论文
溯源树
样例
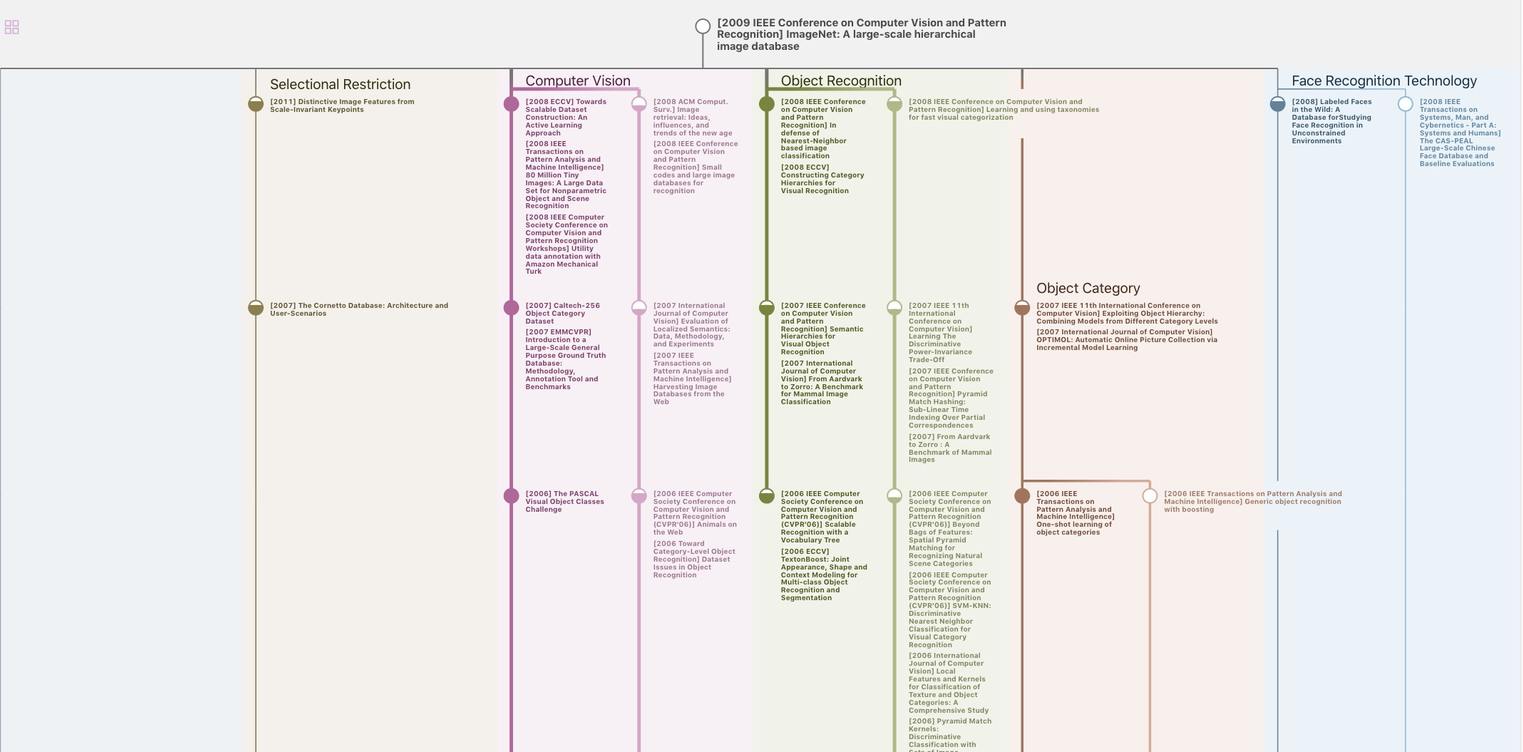
生成溯源树,研究论文发展脉络
Chat Paper
正在生成论文摘要