Fault Diagnosis of Exhaust Gas Treatment System Combining Physical Insights and Neural Networks
IFAC PAPERSONLINE(2022)
摘要
Fault diagnosis is important for automotive systems, e.g., to reduce emissions and improve system reliability. Developing diagnosis systems is complicated by model inaccuracies and limited training data from relevant operating conditions, especially for new products and models. One solution is the use of hybrid fault diagnosis techniques combining model-based and data-driven methods. In this work, data-driven residual generation for fault detection and isolation is investigated for a system injecting urea into the aftertreatment system of a heavy-duty truck. A set of recurrent neural network-based residual generators is designed using a structural model of the system. The performance of this approach is compared to a baseline model-based approach using data collected from a heavy-duty truck during different fault scenarions with promising results. Copyright (c) 2022 The Authors. This is an open access article under the CC BY-NC-ND license (https://creativecommons.org/licenses/by-nc-nd/4.0/)
更多查看译文
关键词
Methods based on neural networks for FDI, Structural analysis and residual evaluation methods, AI methods for FDI, Modeling, supervision, control and diagnosis of automotive systems, Filtering and change detection
AI 理解论文
溯源树
样例
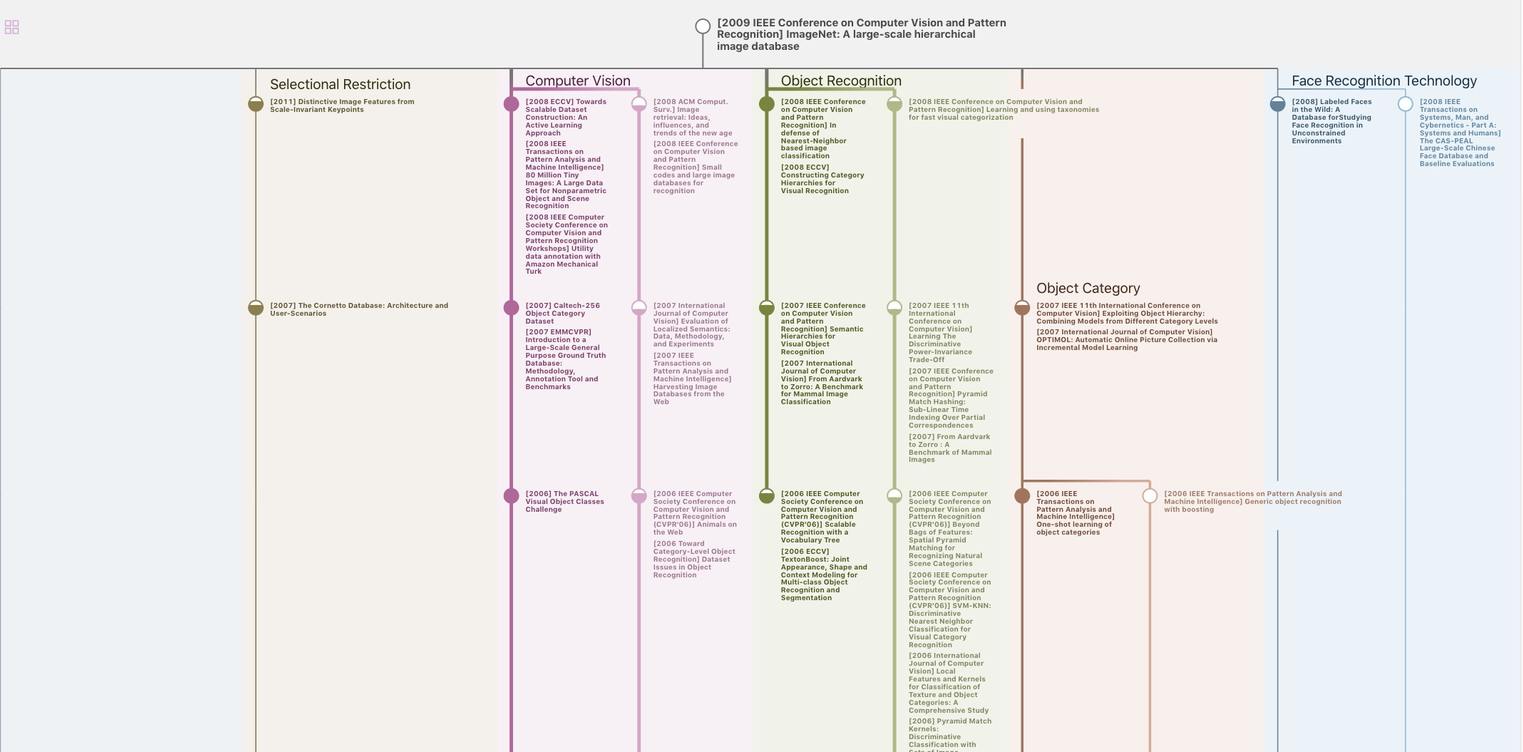
生成溯源树,研究论文发展脉络
Chat Paper
正在生成论文摘要