Grape leaf disease detection based on attention mechanisms
INTERNATIONAL JOURNAL OF AGRICULTURAL AND BIOLOGICAL ENGINEERING(2022)
摘要
Prevention and control of grape diseases is the key measure to ensure grape yield. In order to improve the precision of grape leaf disease detection, in this study, Squeeze-and-Excitation Networks (SE), Efficient Channel Attention (ECA), and Convolutional Block Attention Module (CBAM) attention mechanisms were introduced into Faster Region-based Convolutional Neural Networks (R-CNN), YOLOx, and single shot multibox detector (SSD), to enhance important features and weaken unrelated features and ensure the real-time performance of the model in improving its detection precision. The study showed that Faster R-CNN, YOLOx, and SSD models based on different attention mechanisms effectively enhanced the detection precision and operation speed of the models by slightly enhancing parameters. Optimal models among the three types of models were selected for comparison, and results showed that Faster R-CNN+SE had lower detection precision, YOLOx+ECA required the least parameters with the highest detection precision, and SSD+SE showed optimal real-time performance with relatively high detection precision. This study solved the problem of difficulty in grape leaf disease detection and provided a reference for the analysis of grape diseases and symptoms in automated agricultural production.
更多查看译文
关键词
disease detection, Faster R-CNN, YOLOx, SSD, attention mechanism
AI 理解论文
溯源树
样例
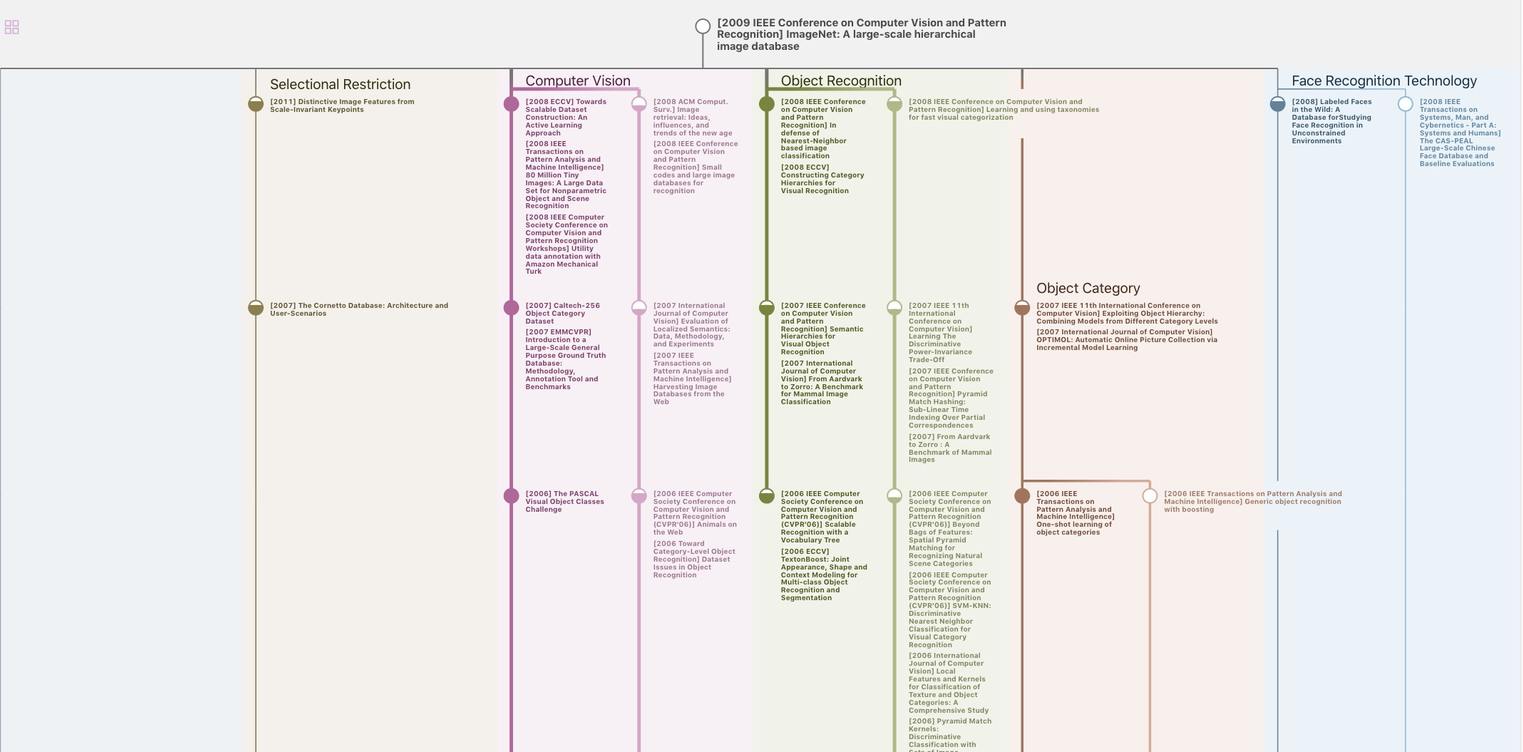
生成溯源树,研究论文发展脉络
Chat Paper
正在生成论文摘要