Network structure indexes to forecast epidemic spreading in real-world complex networks
FRONTIERS IN PHYSICS(2022)
摘要
Complex networks are the preferential framework to model spreading dynamics in several real-world complex systems. Complex networks can describe the contacts between infectious individuals, responsible for disease spreading in real-world systems. Understanding how the network structure affects an epidemic outbreak is therefore of great importance to evaluate the vulnerability of a network and optimize disease control. Here we argue that the best network structure indexes (NSIs) to predict the disease spreading extent in real-world networks are based on the notion of network node distance rather than on network connectivity as commonly believed. We numerically simulated, via a type-SIR model, epidemic outbreaks spreading on 50 real-world networks. We then tested which NSIs, among 40, could a priori better predict the disease fate. We found that the "average normalized node closeness " and the "average node distance " are the best predictors of the initial spreading pace, whereas indexes of "topological complexity " of the network, are the best predictors of both the value of the epidemic peak and the final extent of the spreading. Furthermore, most of the commonly used NSIs are not reliable predictors of the disease spreading extent in real-world networks.
更多查看译文
关键词
complex networks,network spreading,network epidemics,network structural characteristics,SIR (susceptible infected recovered) model
AI 理解论文
溯源树
样例
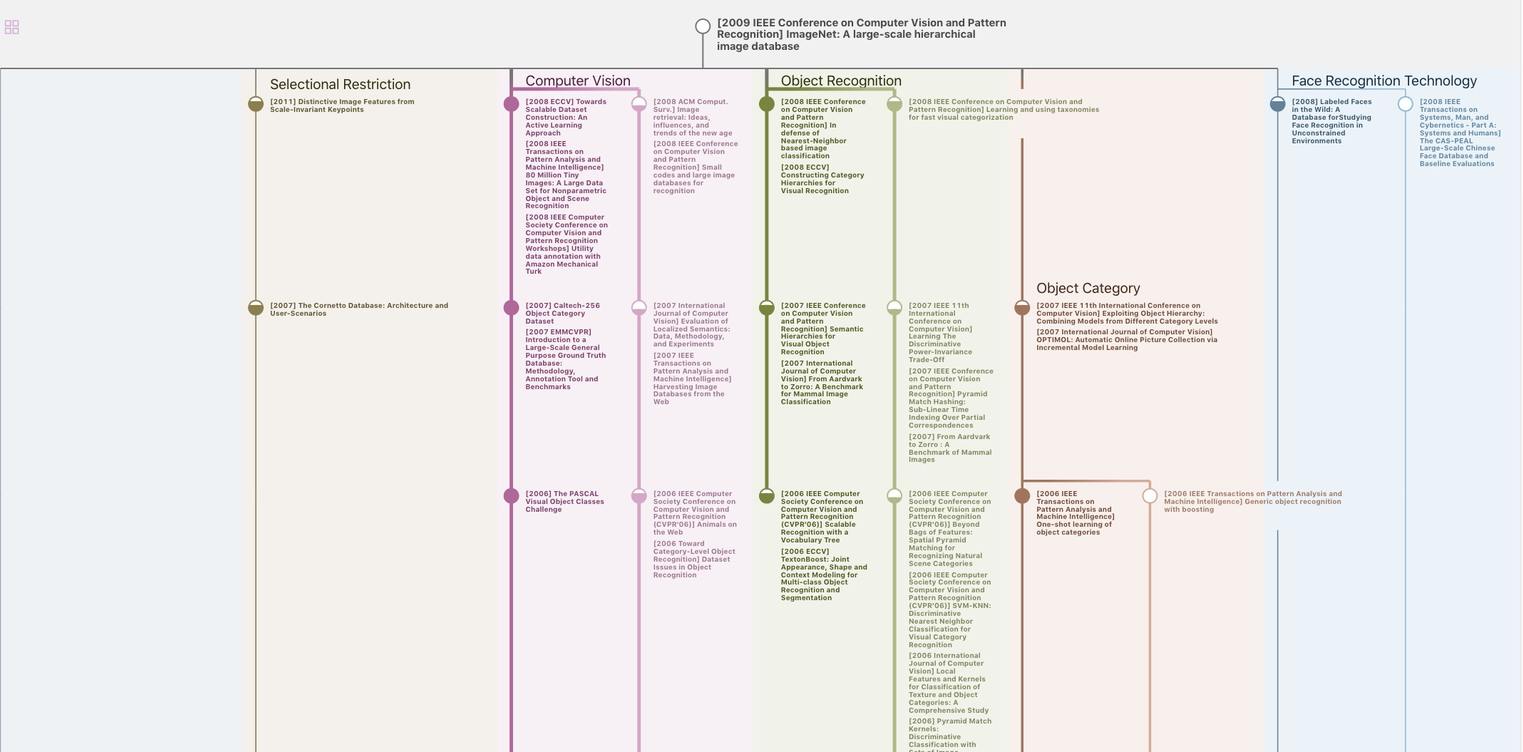
生成溯源树,研究论文发展脉络
Chat Paper
正在生成论文摘要