Automated Gating and Interpretation of Clinical Flow Cytometry Data: A Computational Approach using Artificial Intelligence and Deep Learning
AMERICAN JOURNAL OF CLINICAL PATHOLOGY(2022)
摘要
Abstract Flow cytometry (FCM) allows pathologists to accurately immunophenotype hematopoietic cells by detecting the expression of surface proteins with fluorochrome-conjugated antibodies. Accordingly, FCM plays a pivotal role in the diagnostic workup of many hematologic malignancies. However, post-analytic processing and the interpretation of FCM data are primarily manual processes that impact the consistency and limit the throughput of the method. The post-analytic processing workflow is colloquially referred to as ‘gating’ and involves the identification and characterization of immune cell populations by hand through successions of biaxial plots. The gated FCM data is then used by the pathologist to make a clinical interpretation and to then determine potential diagnoses from the patterns and cell frequencies seen in bivariate plots of the data. Since gating and interpretation are manually performed, post-analytic analyses of FCM data are not only laborious from a workflow perspective but remain subjective and prone to variability based on the experience and skill of both the medical laboratory scientist and pathologist. The objective of this study was to develop a computational pipeline that leverages machine learning-based (ML) solutions to automate gating and clinical interpretation of FCM data to increase the throughput and improve the repeatability of FCM analysis. Raw FCS files from clinical samples being evaluated for the presence of T-cell lymphoproliferative disease were exported from the on-instrument database. Automated gating was performed using open-source, supervised-ML packages for flow cytometry data (flowCore and flowDensity; R). These packages implement all processing steps that would typically be done manually (e.g. applying compensation, quality control (QC), and gating). Plots of interest were then generated from the gated data and classified as normal or abnormal using the clinical interpretations that were applied during the normal clinical workflow. These binary labels were used to train an ML-based classifier (VGG-19; Python). To evaluate pipeline performance, we collected FCS files from 1,188 samples that were analyzed by our flow cytometry lab. The automated gating pipeline was used to gate for CD4+CD3+ cells and to create bivariate plots for CD7/CD26 expression. 1150 (96.8%) passed QC and were all gated correctly by visual inspection. Of the 38 (3.2%) samples that failed QC, 13 (1.1%) were lymphopenic and were gated correctly by visual inspection, and the remaining 25 (2.1%) were gated manually. Using the CD7/CD26 plots, the classifier demonstrated promising predictive performance and achieved a precision of 0.85 and recall of 0.83 (weighted average across classes). Our findings represent a novel effort to automate both the gating and interpretation of FCM data using artificial intelligence. These results suggest that ML-based tools have potential utility in aiding the processing and interpretation of FCM data and can augment the efficiency and consistency of workflows in the clinical flow cytometry laboratory.
更多查看译文
关键词
clinical flow cytometry data,flow cytometry,deep learning,artificial intelligence
AI 理解论文
溯源树
样例
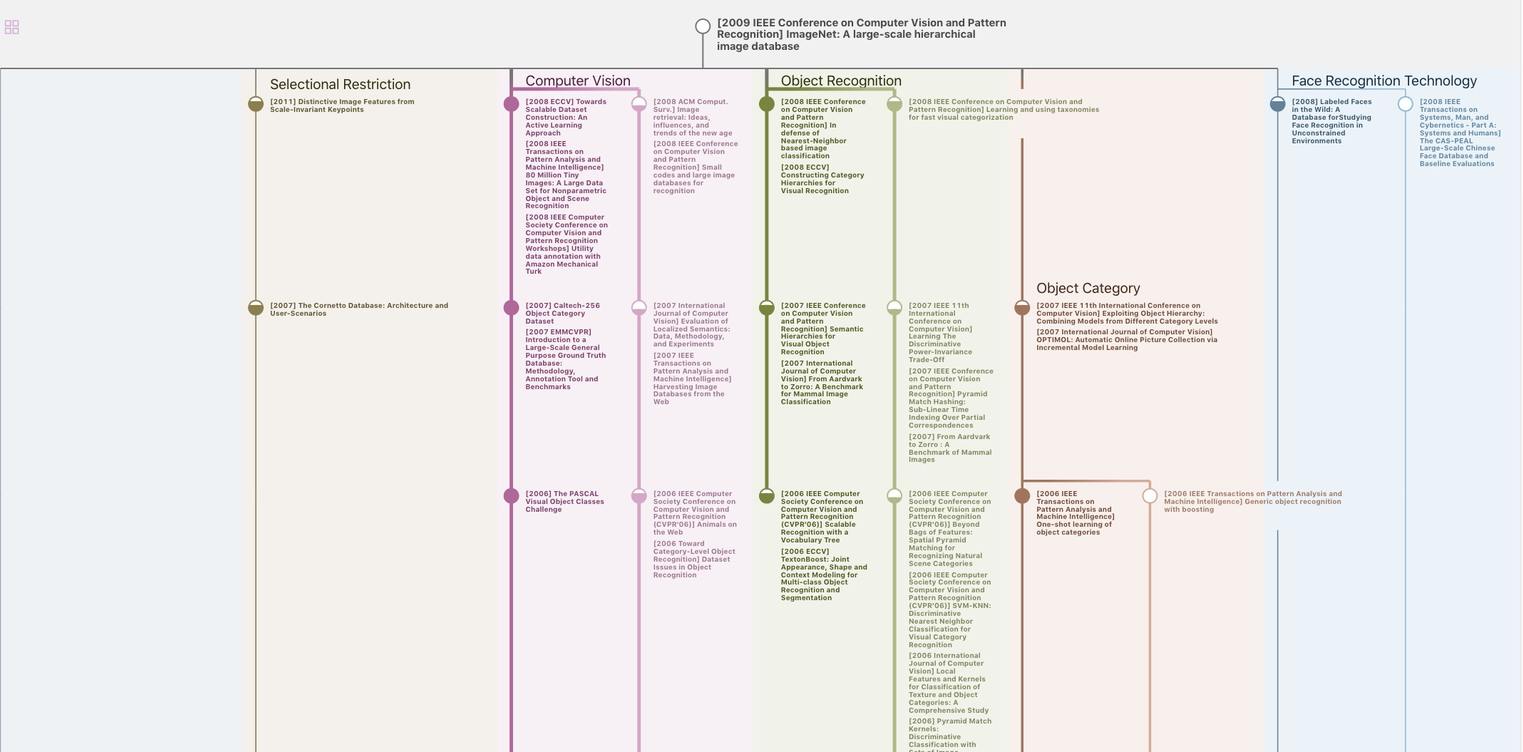
生成溯源树,研究论文发展脉络
Chat Paper
正在生成论文摘要