Applying Deep Learning Cancer Subtyping Algorithms Trained on Physical Slides to Multiphoton Imaging of Unembedded Samples
AMERICAN JOURNAL OF CLINICAL PATHOLOGY(2022)
摘要
Abstract Introduction/Objective Deep learning algorithms on digital images of physical tissue slides have shown potential improvements in accuracy and precision of diagnostic interpretation of neoplastic histology. Clustering-constrained- attention multiple-instance learning (CLAM) is one such method that identifies diagnostic sub-regions to accurately classify whole slides. Often, algorithm performance degrades when deployed on datasets that differ from the original set and it is subject to physical slide preparation variability. New multiphoton imaging modalities have potential workflow and quality advantages over physical slides, producing images analogous to whole slide imaging (WSI) without cutting artifacts, but performance of existing algorithms trained on digitized physical slides and applied to multiphoton images remains completely unknown. Given this, we aimed to test the performance of CLAM algorithms for subtyping renal cell carcinoma (RCC) and lung cancer (LC) applied to pseudo-colored multiphoton WSI. Methods/Case Report Clinical RCC and LC surgical resection samples were processed and imaged by Clearing Histology with MulitPhoton microscopy (CHiMP, Applikate Technologies, Fairfield, CT), producing digital images of un- cut, un-embeded tissue to generate H&E-like optical slices. Multiphoton images were downscaled to 0.5 um/px to match algorithm target resolution. CLAM models for subtyping RCC (chromophobe, clear cell, papillary) and LC (squamous & adenocarcinoma) previously trained using TCGA and CPTAC whole slide images of physical slides were applied directly to CHiMP multiphoton images without adjustment. Reference cancer subtype classifications were provided from physical and digital slides. Results (if a Case Study enter NA) For the subtypes included during training, multiphoton WSIs of RCC and LC were accurately subtyped by the CLAM models without stain normalization nor network fine tuning producing high prediction levels. Subtypes not included during the training (namely oncocytoma for RCC) resulted in low scoring model predictions (below 0.85), indicating specificity of identification. Multiple slide levels improved interpretation of several difficult cases for CLAM predictions. Conclusion This preliminary data suggests that CLAM models trained on standard H&E WSIs for RCC and LC subtyping are applicable to pseudo-H&E multiphoton WSIs without domain adaptations. This implies that diagnostic histologic features have been learned by these CLAM models and are efficiently recognized in digital histology images produced via CHiMP.
更多查看译文
AI 理解论文
溯源树
样例
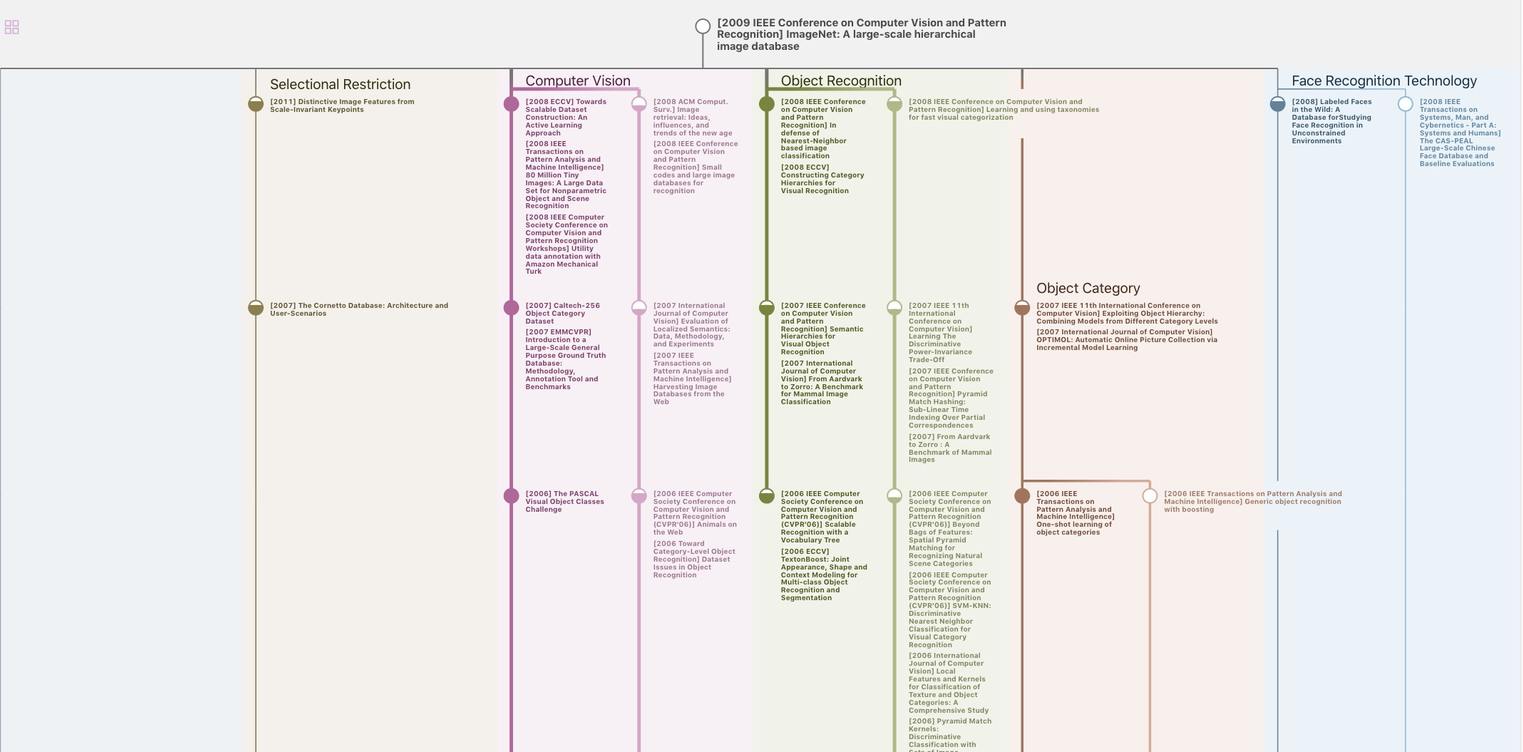
生成溯源树,研究论文发展脉络
Chat Paper
正在生成论文摘要