Using Image Analysis and Regression Modeling to Develop a Diagnostic Tool for Peanut Foliar Symptoms
AGRONOMY-BASEL(2022)
摘要
Peanut foliar diseases and disorders can be difficult to rapidly diagnose with little experience because some abiotic and biotic symptoms present similar symptoms. Developing algorithms for automated identification of peanut foliar diseases and disorders could potentially provide a quick, affordable, and easy method for diagnosing peanut symptoms. To examine this, images of peanut leaves were captured from various angles, distances, and lighting conditions using various cameras. Color space data from all images was subsequently extracted and subjected to logistic regression. Separate algorithms were developed for each symptom to include healthy, hopperburn, late leaf spot, Provost injury, tomato spotted wilt, paraquat injury, or surfactant injury. The majority of these symptoms are not included within currently available disease identification mobile apps. All of the algorithms developed for peanut foliar diagnostics were >= 86% accurate. These diagnostic algorithms have the potential to be a valuable tool for growers if made available via a web-accessible platform, which is the next step of this work.
更多查看译文
关键词
Arachis hypogaea,late leaf spot,tomato spotted wilt,hopperburn,pesticide injury
AI 理解论文
溯源树
样例
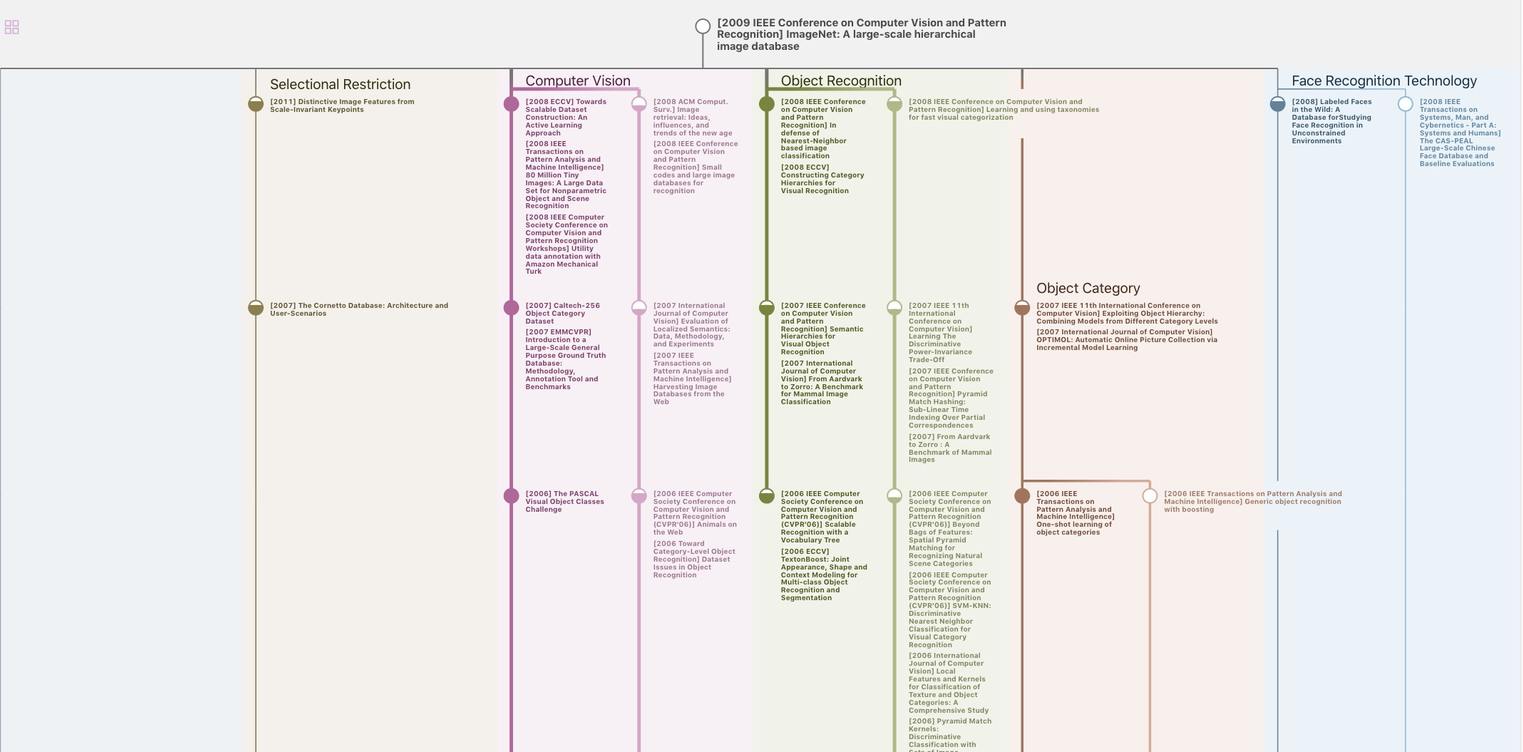
生成溯源树,研究论文发展脉络
Chat Paper
正在生成论文摘要