Pushing the Accuracy of Thai Food Image Classification with Transfer Learning
ENGINEERING JOURNAL-THAILAND(2022)
摘要
Food image classification is a challenging problem, the solution of which can be of great benefit to many real-world applications such as nutrition and allergy estimation. Most of the previous studies proposed to use variations of convolutional neural networks to tackle the problem. However, due to the limited number of annotated food image datasets, there is still some room for improvement, especially in terms of accuracy and speed. Generally speaking, neural networks trained to solve image classification problems on a small dataset benefit from utilizing the weights of the networks that have been pre-trained on a large image classification dataset such as ImageNet. In this paper, we compare the trade-offs between training networks from scratch, deploying pre-trained networks as feature extractors, and fine-tuning the networks for Thai food image classification. By utilizing Transfer Learning with EfficientNetV1, we were able to achieve higher accuracy for Thai Food Image Classification on the largest publicly available Thai food image dataset, THFOOD-50. In particular, our proposed method improves upon the accuracy of the previous state-of-the-art method from 84.06% to 91.49% while maintaining the speed for the prediction at 103 ms and 1205 ms for GPU and CPU, respectively.
更多查看译文
关键词
Thai food image classification, food image classification, deep learning, neural networks, transfer learning
AI 理解论文
溯源树
样例
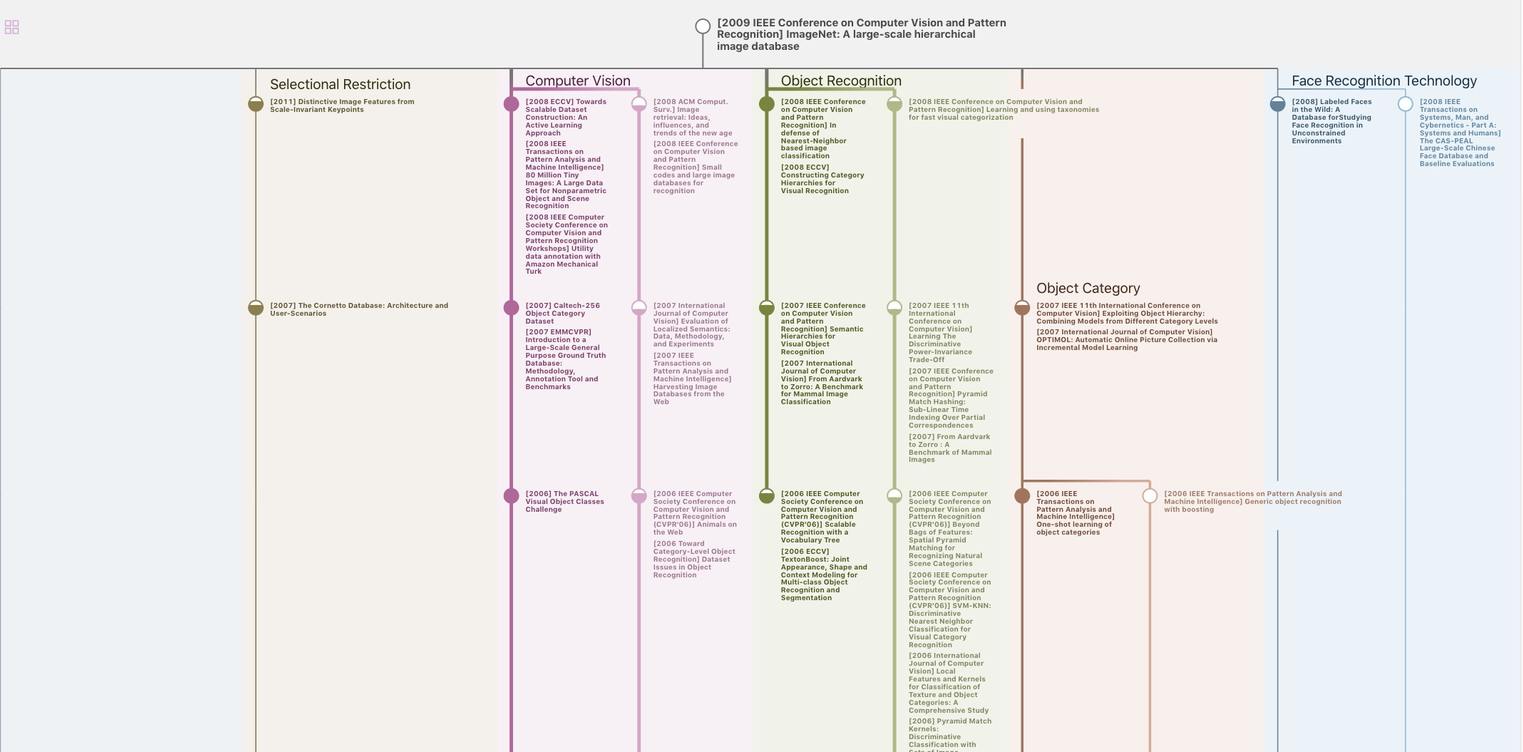
生成溯源树,研究论文发展脉络
Chat Paper
正在生成论文摘要