Machine Learning Wavefront Sensing for the James Webb Space Telescope
SPACE TELESCOPES AND INSTRUMENTATION 2022: OPTICAL, INFRARED, AND MILLIMETER WAVE(2022)
摘要
The fine alignment of the James Webb Space Telescope (JWST)'s 18 primary mirror segments relies on image-based wavefront sensing. Previously a convolutional neural network (CNN) produced phase estimates with around 0.37 waves RMS error, which were adequate as starting guesses for phase retrieval to accurately predict global Zernike coefficients. In contrast, our study uses a CNN to sense segment piston errors between the 18 segments. Our trained CNN model could retrieve segment piston phase errors of +/- 0.5 waves from a single defocused point-spread function to within 0.02 waves RMS, without the need of additional phase retrieval algorithms.
更多查看译文
关键词
JWST, wavefront sensing, convolutional neural network, machine learning
AI 理解论文
溯源树
样例
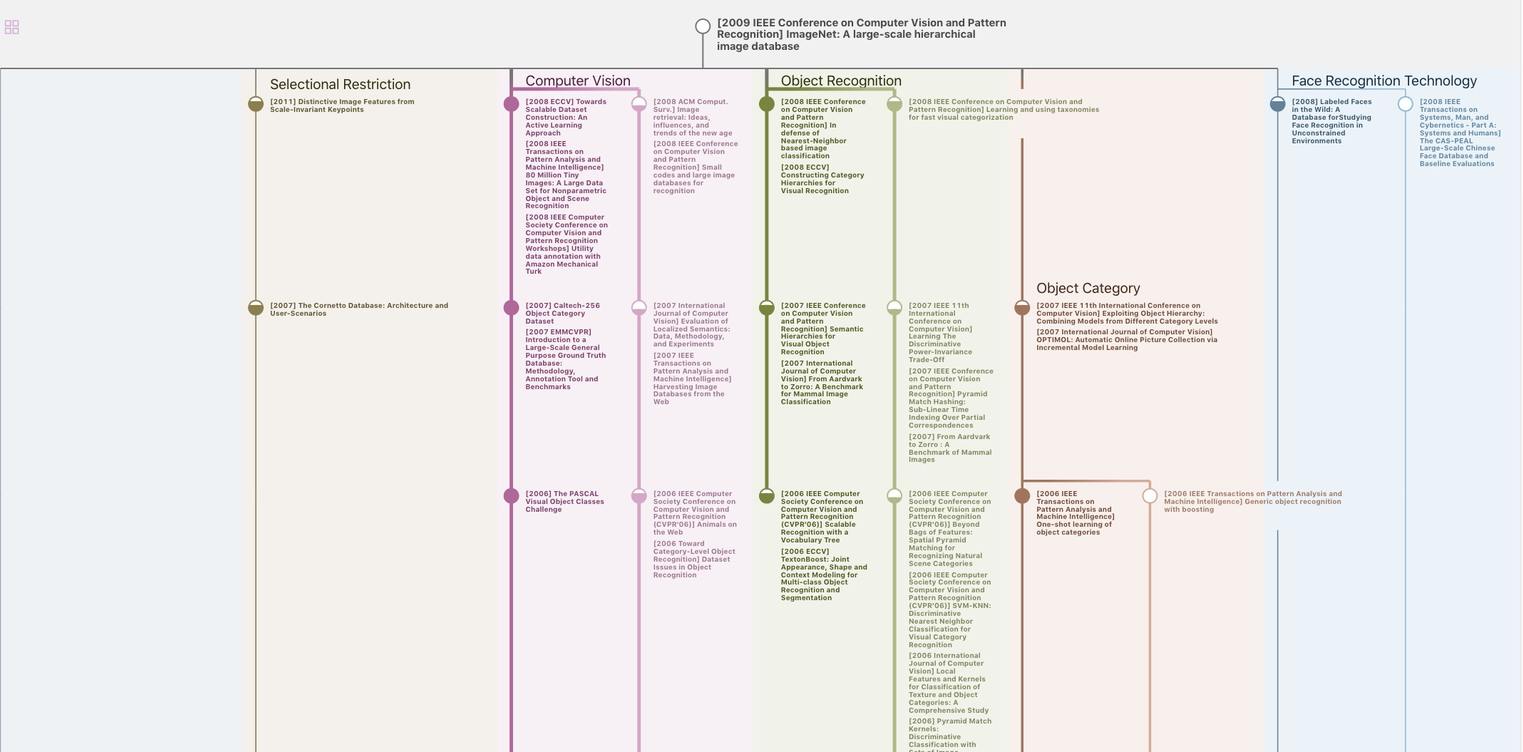
生成溯源树,研究论文发展脉络
Chat Paper
正在生成论文摘要