Concurrent estimation of montelukast and loratadine mixture in spiked human plasma utilizing high-performance thin-layer chromatography with ultraviolet detection
JPC-JOURNAL OF PLANAR CHROMATOGRAPHY-MODERN TLC(2022)
摘要
A novel, fast, and cost-effective high-performance thin-layer chromatography (HPTLC) with reflectance/absorbance densitometry was developed and validated for the simultaneous estimation of montelukast (MONT) and loratadine (LOR) in bulk and spiked human plasma. Separation was attained on a pre-coated silica gel HPTLC plate with chloroform–ethyl acetate (8:2, V/V ) as the mobile phase. Quantification was performed at 280 nm in range concentrations of 48–300 ng/band for MONT and 90–600 ng/band for LOR. This system gave compact bands with high resolution for MONT at a retardation factor ( R F ) of 0.17 ± 0.002 and 0.39 ± 0.001 for LOR. The developed methodology was validated on the basis of the International Council for Harmonization (ICH) guidelines. The lower detectable amounts were 9.47 and 22.16 ng/band for MONT and LOR, respectively, and the limits of quantification were 28.7 and 67.2 ng/band for MONT and LOR, respectively. The suggested HPTLC methodology is sensitive, selective, accurate, and precise. Therefore, the method could efficiently be utilized for the detection and estimation of MONT and LOR simultaneously in pure powders and human plasma samples.
更多查看译文
关键词
Thin-layer chromatography-densitometry, Absorbance, Montelukast, Loratadine, Plasma
AI 理解论文
溯源树
样例
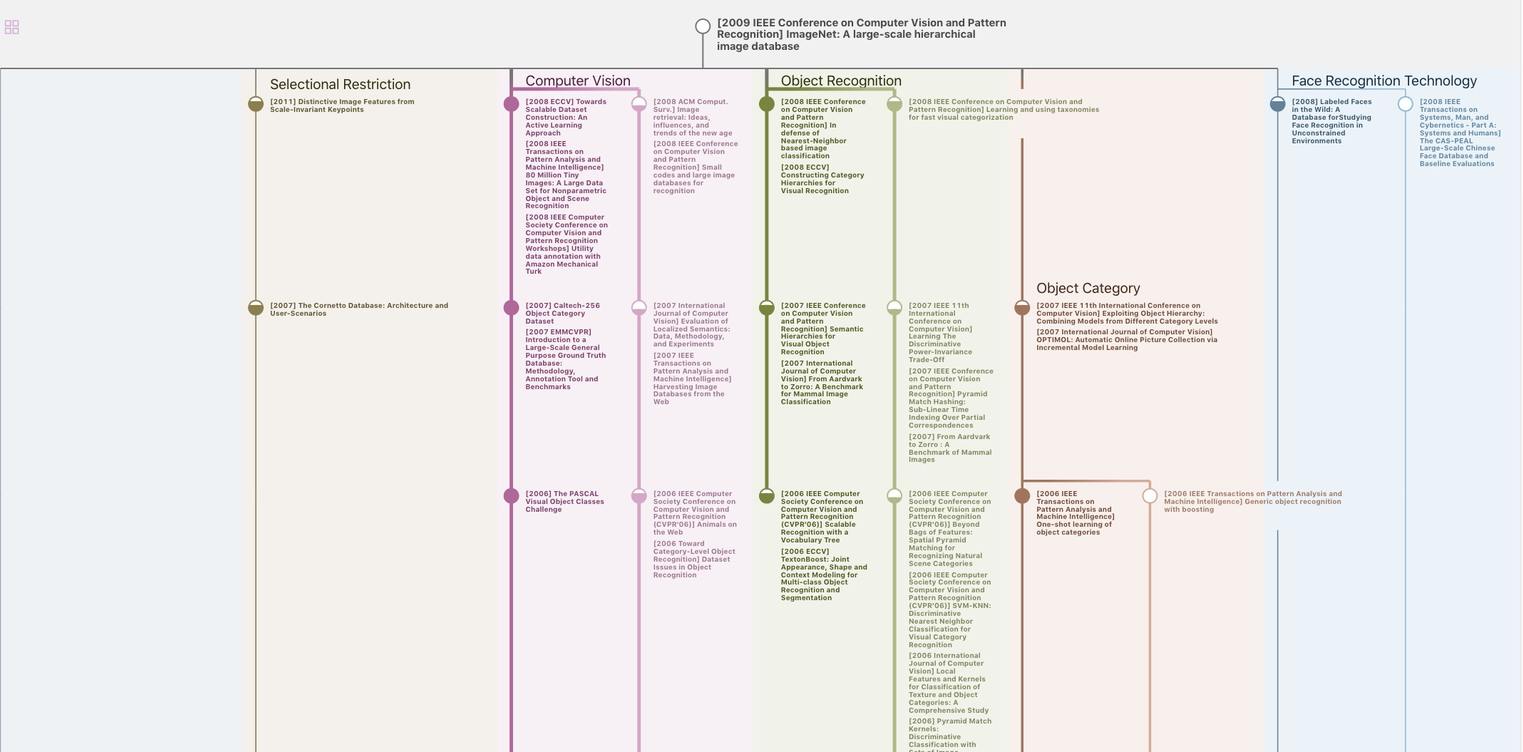
生成溯源树,研究论文发展脉络
Chat Paper
正在生成论文摘要