Development of a Robust Data-Driven Soft Sensor for Multivariate Industrial Processes with Non-Gaussian Noise and Outliers
MATHEMATICS(2022)
摘要
Industrial processes are often nonlinear and multivariate and suffer from non-Gaussian noise and outliers in the process data, which cause significant challenges in data-driven modelling. To address these issues, a robust soft-sensing algorithm that integrates Huber's M-estimation and adaptive regularisations with multilayer perceptron (MLP) is proposed in this paper. The proposed algorithm, called RAdLASSO-MLP, starts with an initially well-trained MLP for nonlinear data-driven modelling. Subsequently, the residuals of the proposed model are robustified with Huber's M-estimation to improve the resistance to non-Gaussian noise and outliers. Moreover, a double L1-regularisation mechanism is introduced to minimise redundancies in the input and hidden layers of MLP. In addition, the maximal information coefficient (MIC) index is investigated and used to design the adaptive operator for the L1-regularisation of the input neurons to improve biased estimations with L1-regularisation. Including shrinkage parameters and Huber's M-estimation parameter, the hyperparameters are determined via grid search and cross-validation. To evaluate the proposed algorithm, simulations were conducted with both an artificial dataset and an industrial dataset from a practical gasoline treatment process. The results indicate that the proposed algorithm is superior in terms of predictive accuracy and robustness to the classic MLP and the regularised soft-sensing approaches LASSO-MLP and dLASSO-MLP.
更多查看译文
关键词
date-driven modelling, soft sensor, multilayer perceptron, LASSO, maximal information coefficient, robust estimation
AI 理解论文
溯源树
样例
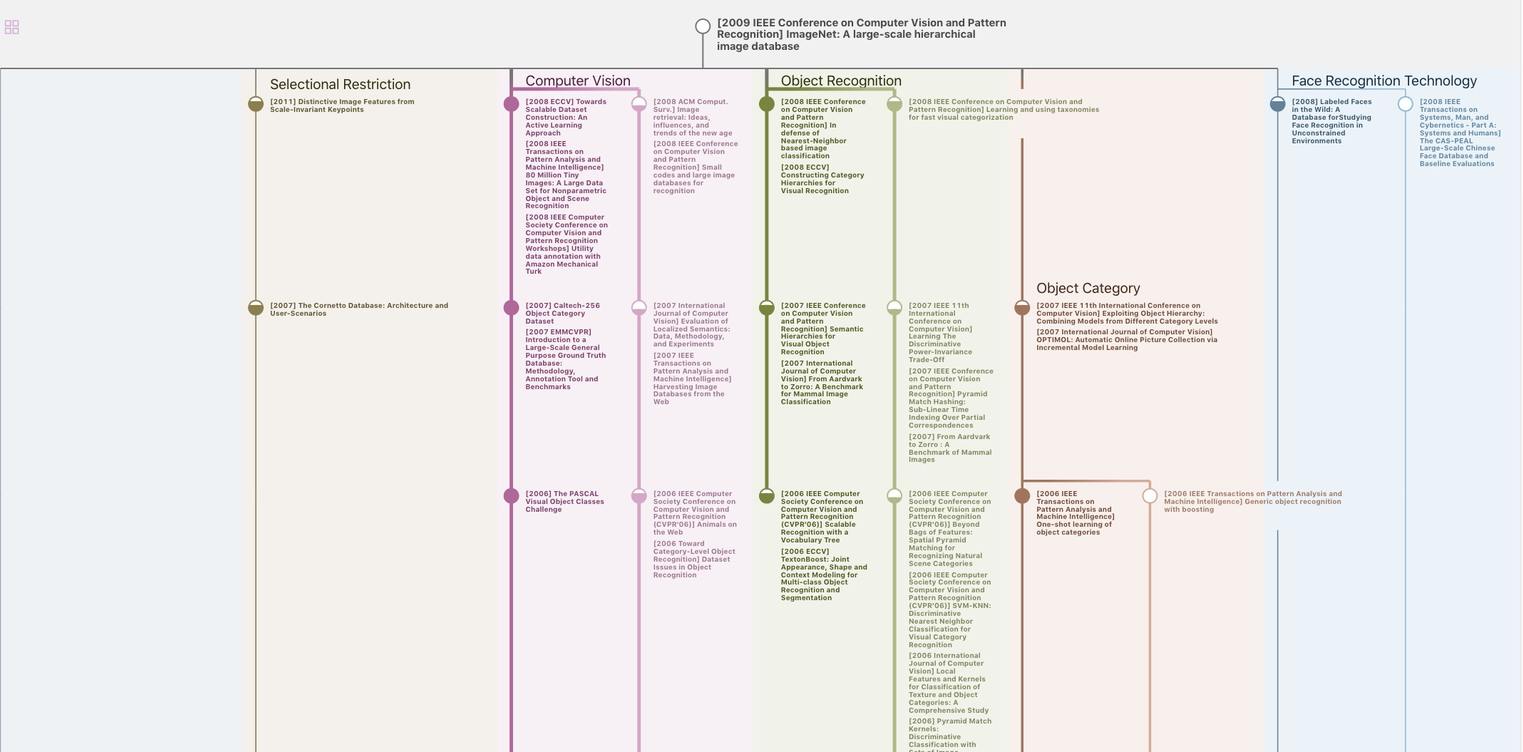
生成溯源树,研究论文发展脉络
Chat Paper
正在生成论文摘要