A comparative assessment of machine learning methods in extracting place functionality from textual content
TRANSACTIONS IN GIS(2022)
摘要
Places are usually ambiguous and context dependent. Place functionality as a context in place descriptions is one of the prominent and distinguishing features of place. Today, due to the rapid growth of the Internet and social networks, users usually share place-based information. Among the types of information, user-generated textual content is not usually shared in a specific structure. This article aims to extract place functionality using analysis of user-generated textual contents shared by users. For this purpose, we try to extract and predict the place functionality through the whole user reviews as well as only the action verbs expressed in the reviews. In this research, series of comparative experiments was conducted to highlight the influence of action verbs on the prediction of place functionality and to evaluate the predictability across different natural language processing (NLP) methods. We compare the efficiency of three NLP methods in selecting and extracting features, as well as different machine learning text classifier methods to predict place functionality. Evaluation results show that using the whole text of the review can extract place functionality better than using only the action verbs. Also, the bag-of-words feature extraction method performs better than the two embedding methods. Among text classification algorithms, although the multinomial naive Bayes method is the fastest method, the support vector machine method is more accurate than all other methods, while the execution time of the algorithm is longer. In addition, among the various predicted functionalities, the algorithm's efficiency is higher in Food Places. While the Vacation Rentals are less accurate regarding the high functional similarity to Hotels. In addition, the sub-categories of Food Places and Shops are predicted with the average accuracy of 89.48 and 84.41% for Food Places with lemmatized words and action verbs, respectively, and the average accuracy of 95.89 and 79.84% for Shops with lemmatized words and action verbs, respectively.
更多查看译文
关键词
place functionality,machine learning methods,machine learning,content
AI 理解论文
溯源树
样例
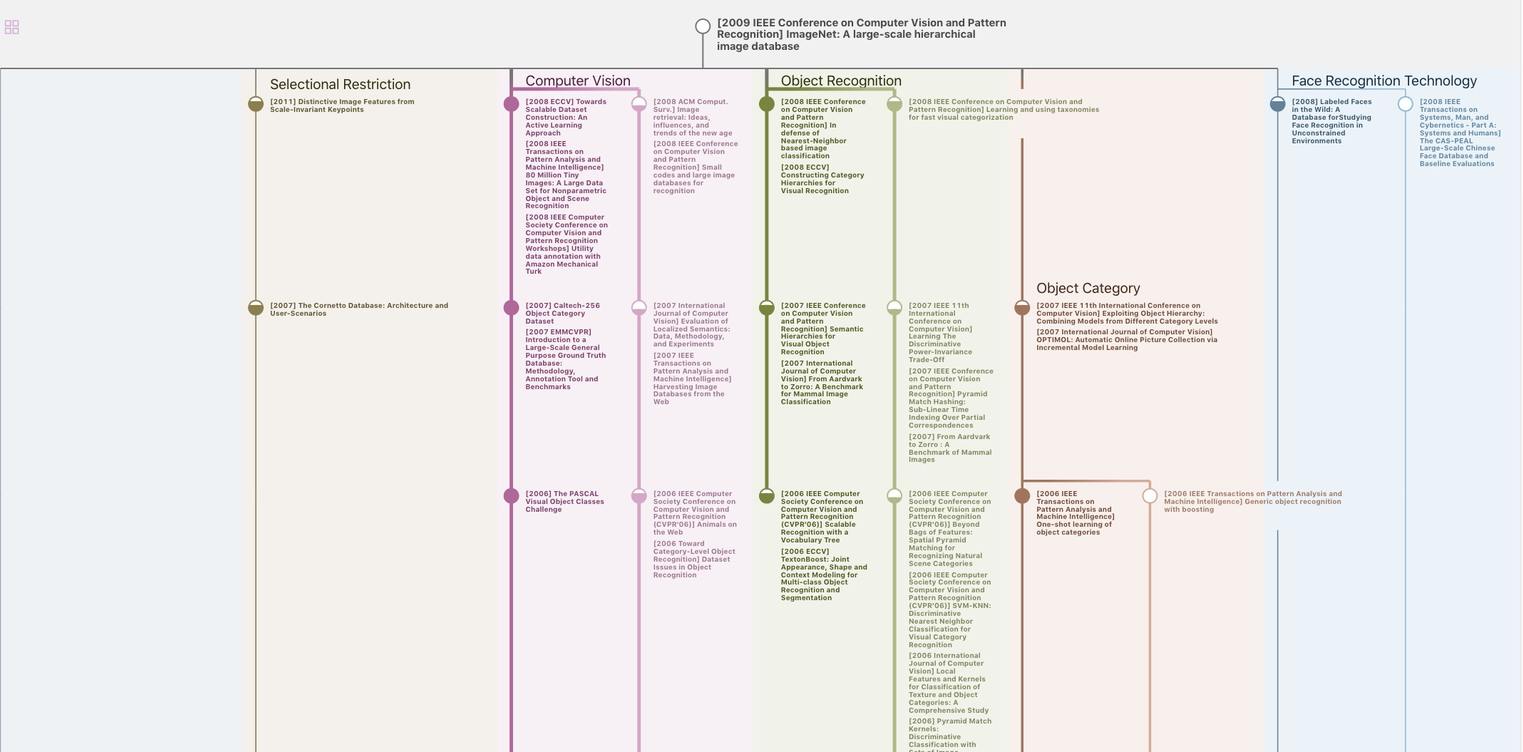
生成溯源树,研究论文发展脉络
Chat Paper
正在生成论文摘要