CAD System for Parkinson's Disease with Penalization of Non-significant or High-Variability Input Data Sources
ARTIFICIAL INTELLIGENCE IN NEUROSCIENCE: AFFECTIVE ANALYSIS AND HEALTH APPLICATIONS, PT I(2022)
摘要
In the last decade, the progressive development of new machine learning schemas in combination with novel biomarkers have led us to more accurate models to diagnose and predict the evolution of neurological disorders like Parkinson's Disease (PD). Though some of these previous work have attempted to combine multiple input data sources, many studies are critical of their lack of robustness when combining several input sources that with a high variability and/or not statistically significant. In order to minimize this problem, we have develop a Computer-Aided-Diagnosis (CAD) system for PD able to combine multiple input data sources underestimating those data types with poor classification rates and high variability. This model has been evaluated using FP-CIT SPECT and MRI images from healthy control subjects and patients with Parkinson's Disease. As shown by our results, the cross-validation model proposed here does not only preserves the performance of our CAD system (93.8% of balanced accuracy) but also minimizes its variability even despite the input data sources poorly statistically significant.
更多查看译文
关键词
Machine learning, Ensemble learning, Neuroimaging, Parkinson's disease, Multimodal analysis, Computer-aided-diagnosis
AI 理解论文
溯源树
样例
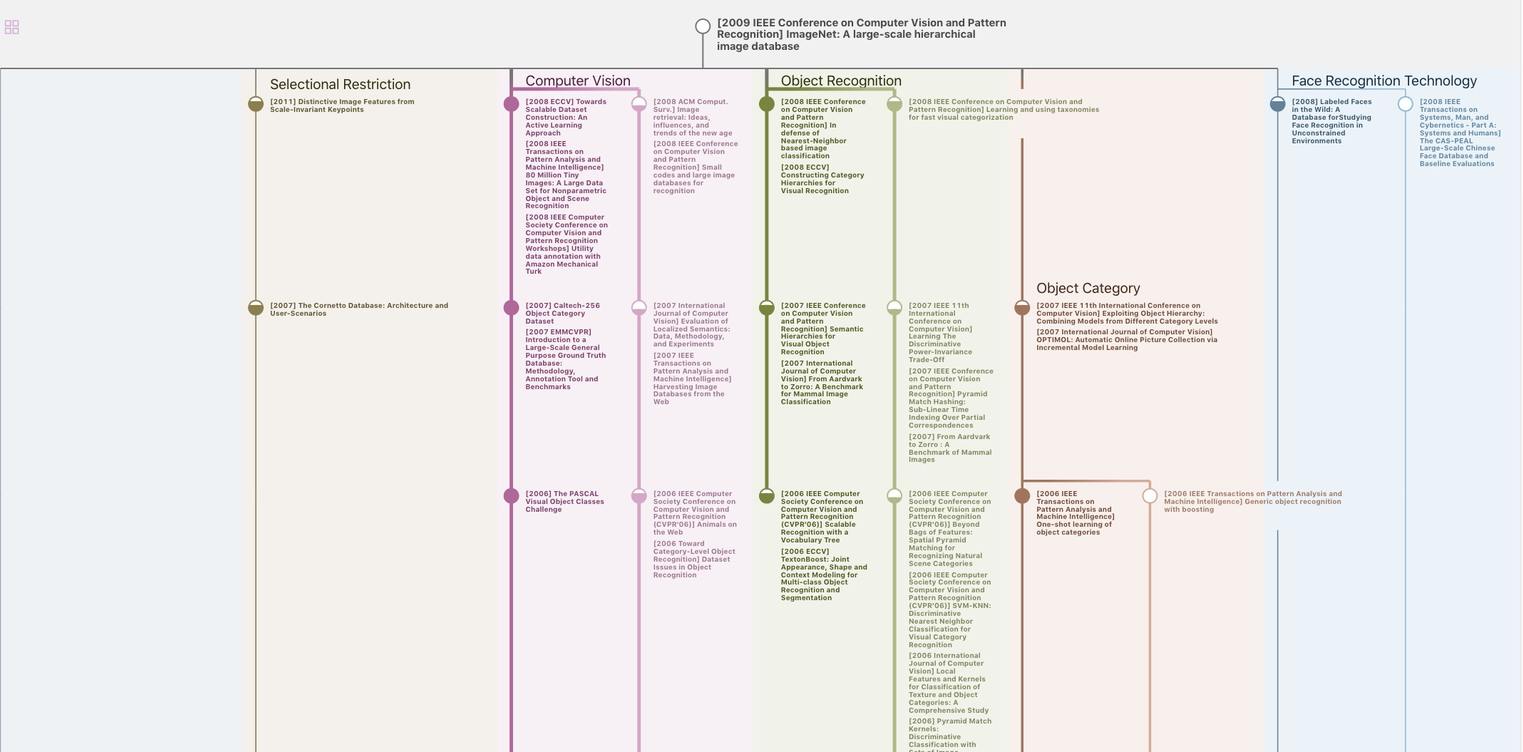
生成溯源树,研究论文发展脉络
Chat Paper
正在生成论文摘要