Data-mining based assembly of promising metal-organic frameworks on Xe/Kr separation
SEPARATION AND PURIFICATION TECHNOLOGY(2023)
摘要
The adsorptive separation of xenon (Xe) and krypton (Kr) becomes increasingly important for the treatment of used nuclear fuel (UNF), and thus the novel high-performing metal-organic frameworks (MOFs) on Xe/Kr adsorption separation are urgently needed. In this work, the 200 MOFs formally used for ethane/ethylene separation were adapted to construct structure-adsorption property relationships (SAPR) for Xe/Kr mixture (20/ 80 v/v) at 298 K and 1 bar, to screen for MOFs with large Xe/Kr selectivities and Xe uptakes in the CoRE MOF, G-MOFs and hMOFs databases with more than 320,000 structures. Then based on the screened 1499 MOFs, the important metal nodes and organic linkers of MOFs (genes) governing the Xe uptake were identified by data -mining of feature engineering, which were assembled crossly into three novel promising MOFs according to material genomics strategy. After considering of the regenerabilities, it is found that Xe uptake (4.2857 mmol/g) and Xe/Kr selectivity (19.70) of the assembled Al2O6-fum_B-hmof8_No1 are larger than those of the most of experimentally synthesized frameworks including Al-Fum-Me, overcoming the "trade-off" problem between adsorption selectivity and capacity. From the multiscale calculations at GCMC and DFT levels, it is found that the large 1D pore size that can accommodate a double-atom chain and large electrostatic potential gradient (EPG) should be responsible for the high Xe uptake and Xe/Kr selectivity of Al2O6-fum_B-hmof8_No1, respectively. Note that the present work first report double-atom chain of rare gas adsorbed in MOF materials. The present data mining and cross assembly strategies are expected to assist the discovery of novel high-performing MOF ab-sorbents for the separation of Xe-Kr even light hydrocarbon in the future.
更多查看译文
关键词
Xe, Kr separation, MOFs, Feature engineering, Cross assembly
AI 理解论文
溯源树
样例
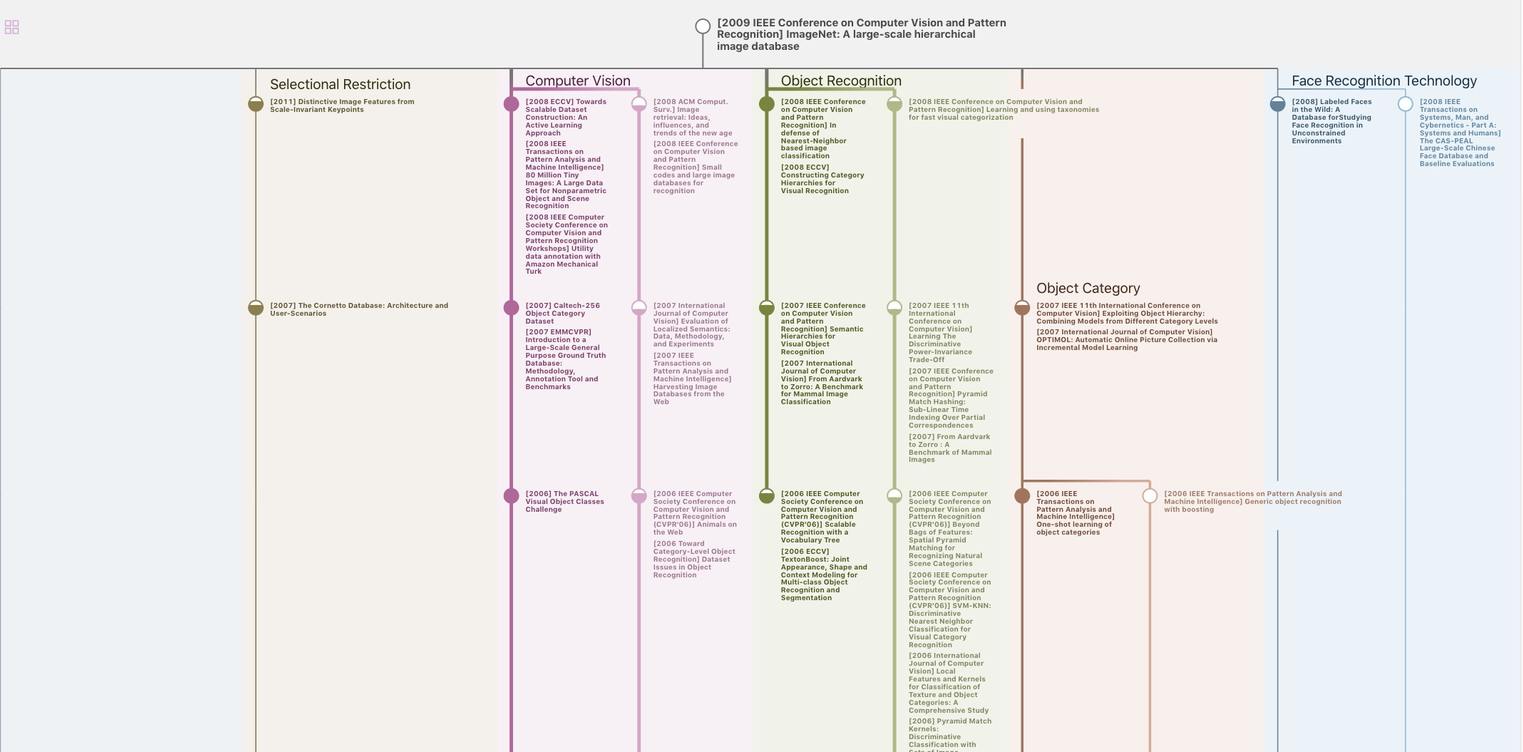
生成溯源树,研究论文发展脉络
Chat Paper
正在生成论文摘要