Data synthesis with dual-stage sample grouping for electromyography signals
EXPERT SYSTEMS WITH APPLICATIONS(2023)
摘要
The correlation-based data synthesis (CDS) model can improve the accuracy of machine learning models via dataset enrichment. However, the performance of the classical CDS model is unsatisfactory when applied to electromyography (EMG) signals because the signal is stochastic and nonstationary. To overcome this problem, this study proposes a new CDS model integrated with dual-stage sample grouping: intra-class clustering followed by intra-cluster random selection. This sample grouping method not only enables the CDS model to create signals similar to the original EMG signal but also increases the diversity of the synthesized dataset. The synthesized sample quality was verified using the sample probability distribution, the Jensen-Shannon divergence, and t -distributed stochastic neighbor embedding plots. Furthermore, the classification accuracy of various machine learning models increased when the synthesized dataset was used for training. Specifically, accuracy improve-ments of 6.29%, 5.09%, 9.26%, and 3.69% were observed for the multi-layer perceptron, support vector machine (SVM) with linear kernel, SVM with radial basis function kernel, and k-nearest neighbor models, respectively. As the EMG signals considerably vary over subjects, the classifiers need to be optimized for individual subjects. The proposed model is useful to create personalized classifiers with a small number of original samples.
更多查看译文
关键词
EMG, Data synthesis, Correlation, Nonstationary signal, Clustering, Random selection
AI 理解论文
溯源树
样例
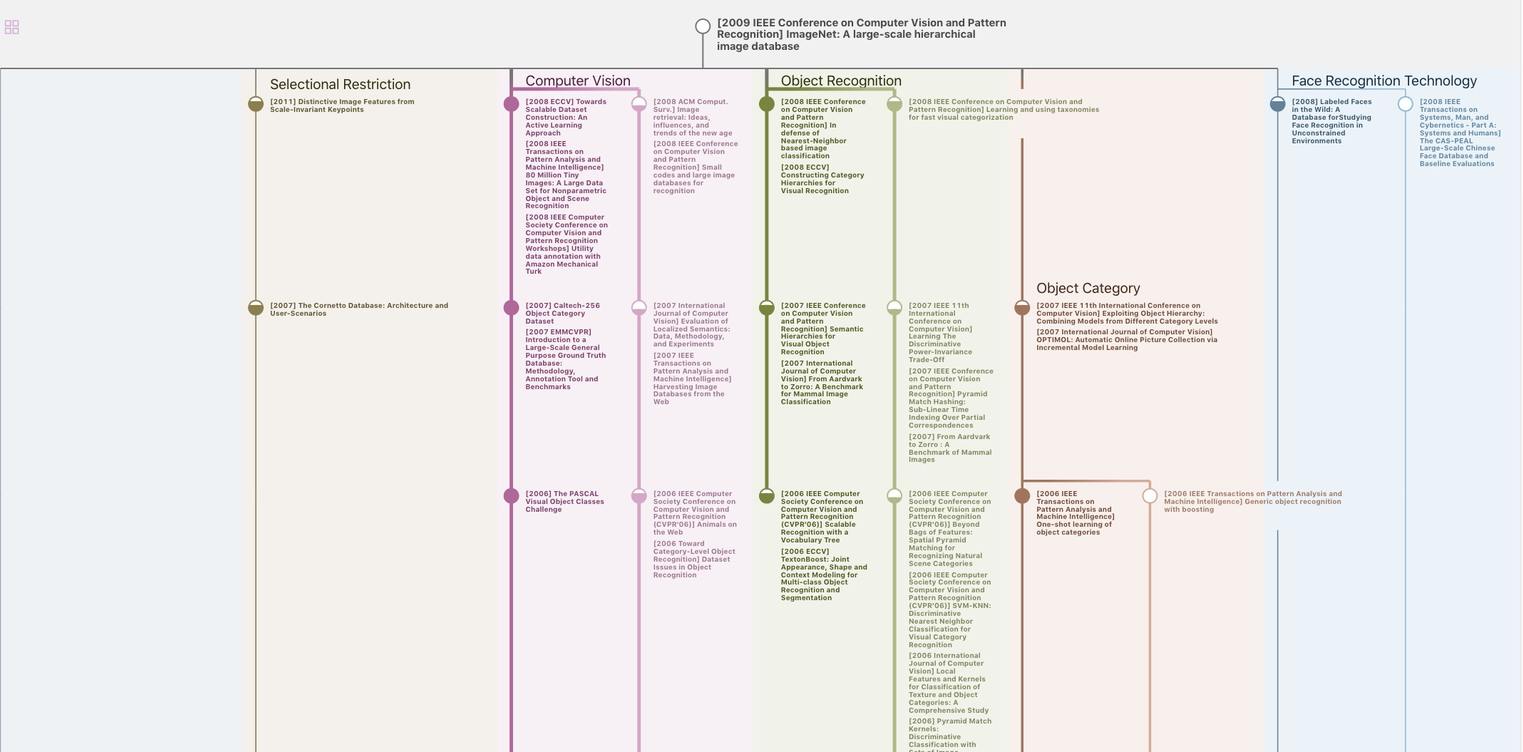
生成溯源树,研究论文发展脉络
Chat Paper
正在生成论文摘要