Structure-Oriented Transformer for retinal diseases grading from OCT images
Computers in Biology and Medicine(2022)
摘要
Retinal diseases are the leading causes of vision temporary or permanent loss. Precise retinal disease grading is a prerequisite for early intervention or specific therapeutic schedules. Existing works based on Convolutional Neural Networks (CNN) focus on typical locality structures and cannot capture long-range dependencies. But retinal disease grading relies more on the relationship between the local lesion and the whole retina, which is consistent with the self-attention mechanism. Therefore, the paper proposes a novel Structure-Oriented Transformer (SoT) framework to further construct the relationship between lesions and retina on clinical datasets. To reduce the dependence on the amount of data, we design structure guidance as a model-oriented filter to emphasize the whole retina structure and guide relation construction. Then, we adopt the pre-trained vision transformer that efficiently models all feature patches’ relationships via transfer learning. Besides, to make the best of all output tokens, a Token vote classifier is proposed to obtain the final grading results. We conduct extensive experiments on one clinical neovascular Age-related Macular Degeneration (nAMD) dataset. The experiments demonstrate the effectiveness of SoT components and improve the ability of relation construction between lesion and retina, which outperforms the state-of-the-art methods for nAMD grading. Furthermore, we evaluate our SoT on one publicly available retinal diseases dataset, which proves our algorithm has classification superiority and good generality.
更多查看译文
关键词
Optical Coherence Tomography,Retinal diseases grading,Vision transformer,Self-attention
AI 理解论文
溯源树
样例
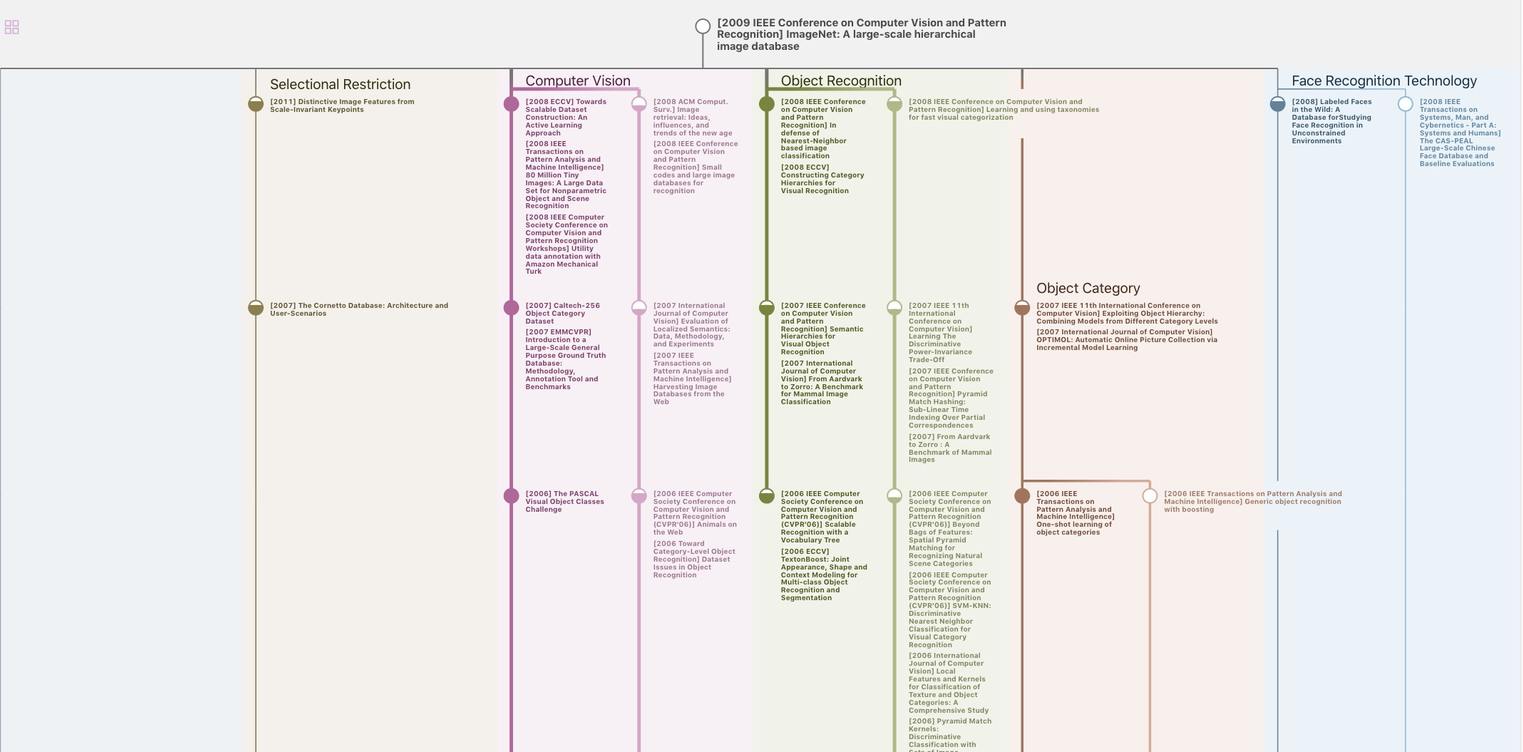
生成溯源树,研究论文发展脉络
Chat Paper
正在生成论文摘要