Variations in the concentration, source and flux of polycyclic aromatic hydrocarbons in sediments of the Pearl River Estuary: Implications for anthropogenic impacts.
The Science of the total environment(2022)
摘要
Variations in the distribution, source composition, mass inventory and burial flux of polycyclic aromatic hydrocarbons (PAHs) in surface sediments from the Pearl River Estuary (PRE) collected in 2011 and 2019 were analyzed to investigate the influence of the anthropogenic activities. Total concentrations of 16 priority PAHs in 2019 (200.40 ± 188.86 ng g-1 dry weight on average) were at the medium level among global bays/estuaries/coastal areas. In 2019, PAH concentrations have decreased by about 50% compared to 2011 and the dominant composition has changed from low- to high-molecular-weight PAHs. The qualitative and quantitative source apportionment analysis indicates that the dominant source of PAHs has shifted from petroleum (40.33%) in 2011 to traffic emission (44.17%) in 2019. The source variation in the PRE can be attributed to the transformation of the energy source structure from petrogenic to pyrogenic in the Pearl River Delta. The estimated PAH mass inventory of the top 5-cm sediment was 38.70 metric tons in 2019, which was about 41 metric tons lower than that in 2011. The average deposition fluxes have dropped from 418.91 ± 261.02 ng cm-2 yr-1 in 2011 to 215.52 ± 246.63 ng cm-2 yr-1 in 2019. The decreasing PAH concentration is related to the sediment coarsening and decline of total organic carbon. These findings in the PRE can be applied to other estuarine environments influenced by anthropogenic activities.
更多查看译文
AI 理解论文
溯源树
样例
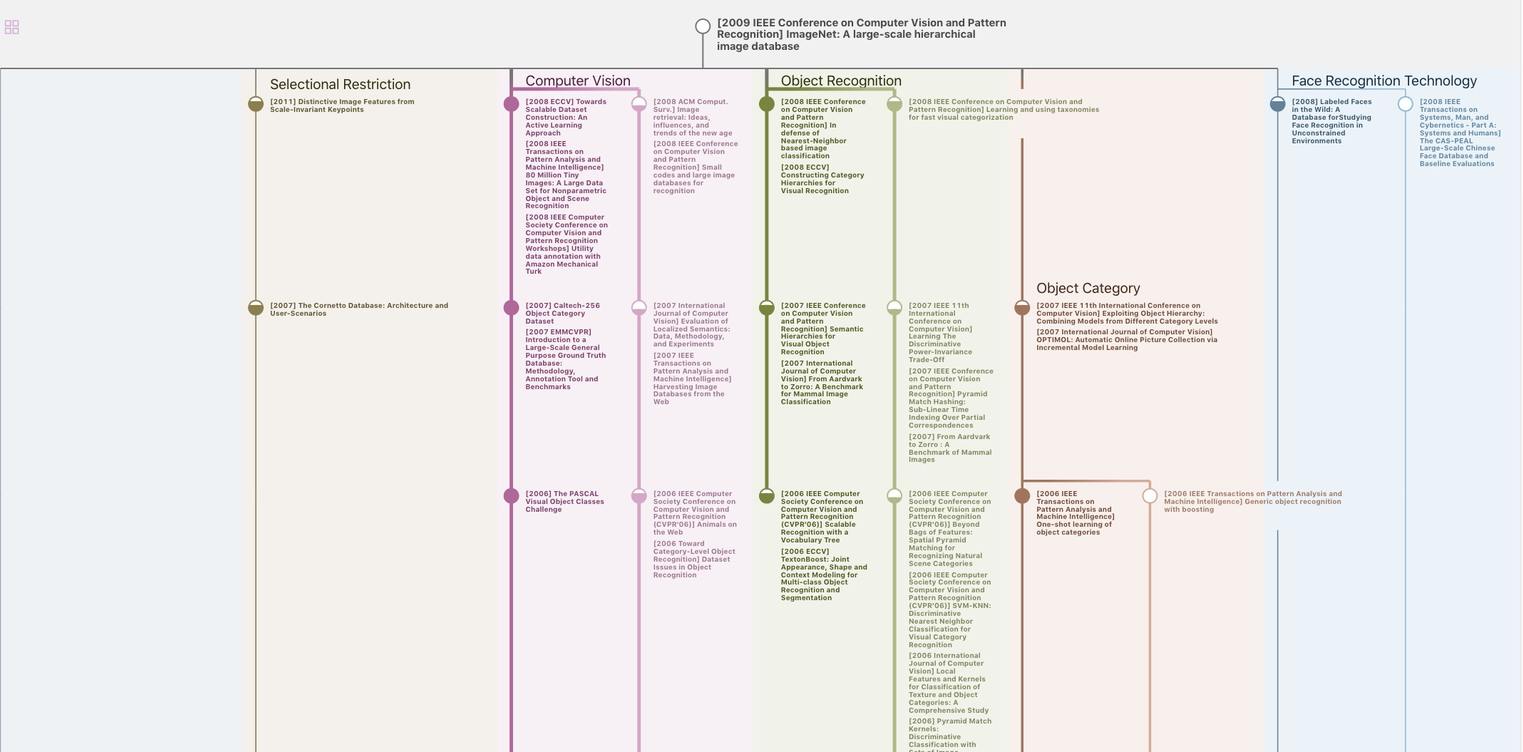
生成溯源树,研究论文发展脉络
Chat Paper
正在生成论文摘要