A Lagrange Programming Neural Network Approach with an l(0)-Norm Sparsity Measurement for Sparse Recovery and Its Circuit Realization
Mathematics(2022)
摘要
Many analog neural network approaches for sparse recovery were based on using l(1)-norm as the surrogate of l(0)-norm. This paper proposes an analog neural network model, namely the Lagrange programming neural network with l(p) objective and quadratic constraint (LPNN-LPQC), with an l(0)-norm sparsity measurement for solving the constrained basis pursuit denoise (CBPDN) problem. As the l(0)-norm is non-differentiable, we first use a differentiable lp-norm-like function to approximate the l(0)-norm. However, this l(p)-norm-like function does not have an explicit expression and, thus, we use the locally competitive algorithm (LCA) concept to handle the nonexistence of the explicit expression. With the LCA approach, the dynamics are defined by the internal state vector. In the proposed model, the thresholding elements are not conventional analog elements in analog optimization. This paper also proposes a circuit realization for the thresholding elements. In the theoretical side, we prove that the equilibrium points of our proposed method satisfy Karush Kuhn Tucker (KKT) conditions of the approximated CBPDN problem, and that the equilibrium points of our proposed method are asymptotically stable. We perform a large scale simulation on various algorithms and analog models. Simulation results show that the proposed algorithm is better than or comparable to several state-of-art numerical algorithms, and that it is better than state-of-art analog neural models.
更多查看译文
关键词
analog neural networks,LPNN,optimization,real-time solution
AI 理解论文
溯源树
样例
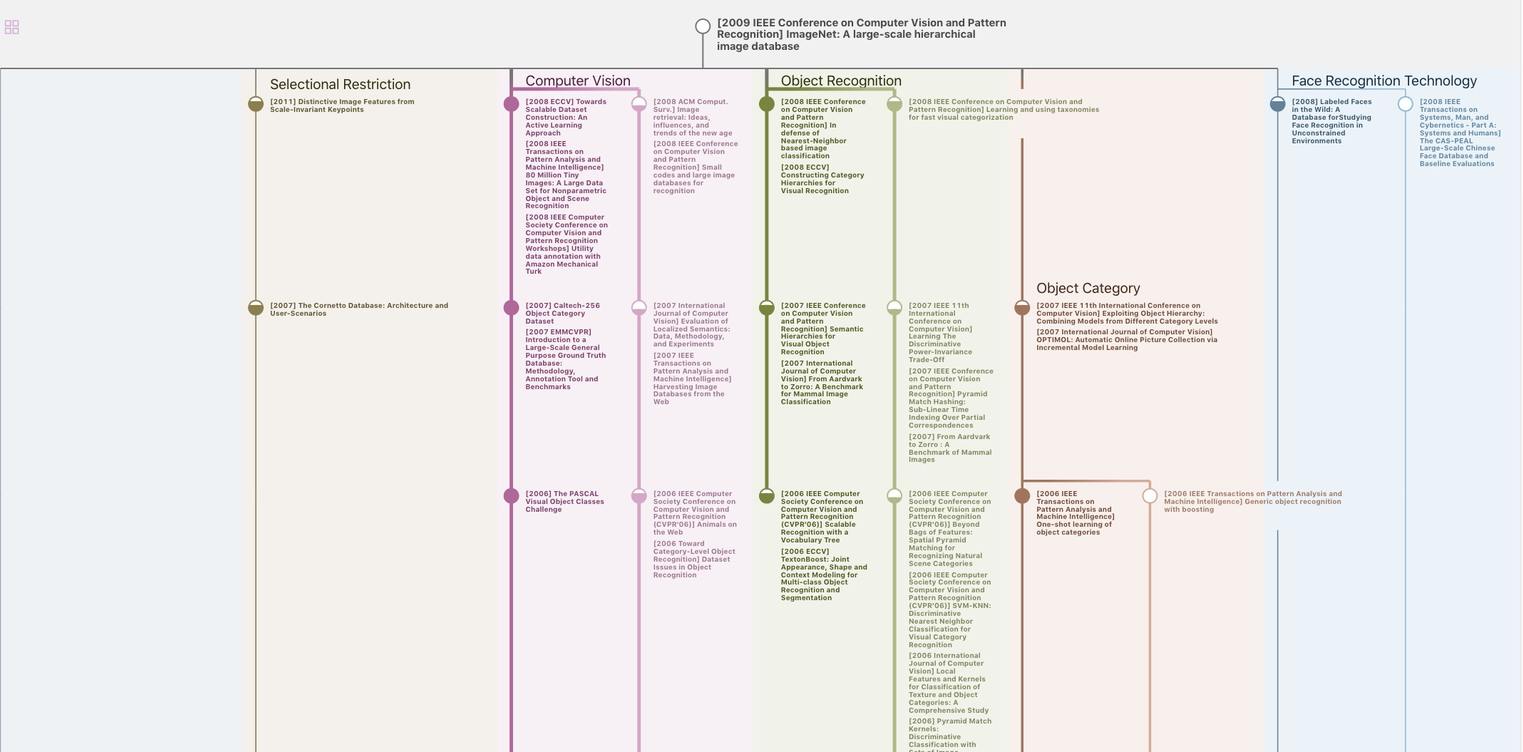
生成溯源树,研究论文发展脉络
Chat Paper
正在生成论文摘要