Deep Learning for Reliable Communication Optimization on Autonomous Vehicles
ACM SIGAda Ada Letters(2022)
摘要
Recent breakthroughs in the autonomous vehicle industry have brought this technology closer to consumers. However, the cost of self-driving solutions still constitutes an entry barrier to many potential users due to its reliance on powerful onboard computers. As an alternative, autonomous driving algorithm processing may be offloaded to remote machines, which requires a reliable connection to the cloud servers. However, despite significant 5G coverage in many countries, mobile network reliability and latency are still inadequate for this purpose. This work explores deep learning concepts to forecast signal quality as a vehicle moves, predicting when periods of degraded network quality will occur. We develop a Long Short-Term Memory (LSTM)-based neural network, trained on multivariate time series containing historical data on several mobile network parameters, and evaluate the results of multi-step Reference Signal Received Power (RSRP) prediction. Results show that our model achieves a rapidly increasing Root-Mean-Square Error (RMSE), reaching over 8 dBm after 25-time steps. This error does not allow for the accurate prediction of future signal quality.
更多查看译文
关键词
autonomous vehicle,deep learning,forecasting,signal quality
AI 理解论文
溯源树
样例
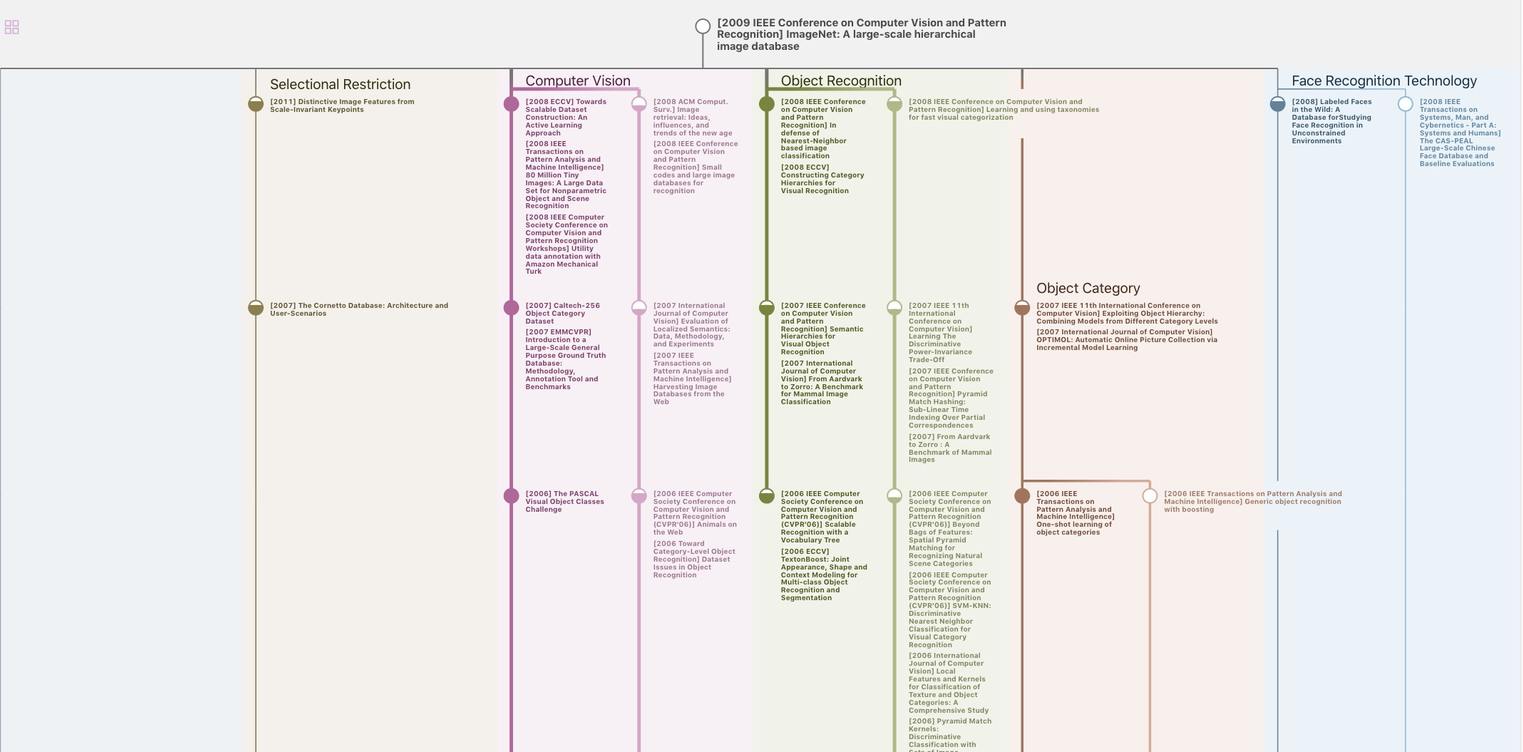
生成溯源树,研究论文发展脉络
Chat Paper
正在生成论文摘要