A federated and explainable approach for insider threat detection in IoT
INTERNET OF THINGS(2023)
摘要
An insider threat is a malicious action launched by authorized personnel inside the organization. Since insider actions may only leave a small digital footprint in the system, it is considered a major cybersecurity challenge in different applications. Along with the rapid growth of the Internet of Things (IoT) and the extensive attack surface in this technology, many concerns have been raised regarding the potential insider threats in IoT environments. Several studies have been conducted on Machine Learning (ML)-based insider threat detection solutions which are focused on the models' performance while the trustability of these models is neglected. Trustworthy Learning refers to a new trend in ML that focuses on ways to ensure that the data collection and data analysis procedures in ML techniques follow ethical applications and are trustable to human users. This approach enforces the acceptance and successful adoption of ML-based solutions. This study aims to propose an improved trustworthy insider threat detection method that ensures two of the trustworthy learning requirements: Privacy and Explainability. The proposed solution protects the privacy of the utilized data and is capable of explaining why certain behaviors are detected as a threat. The proposed solution also leverages data collaboration between different data owners to increase the volume of data used in the training process and enhance the performance of the ML model. Experimental results show the proposed solution outperforms the learning models trained by individual data holders.
更多查看译文
关键词
Insider threat detection,Internet of things,Trustworthy learning,Privacy,Security,Federated learning,Explainable artificial intelligence,Interpretable artificial intelligence,Anomaly detection
AI 理解论文
溯源树
样例
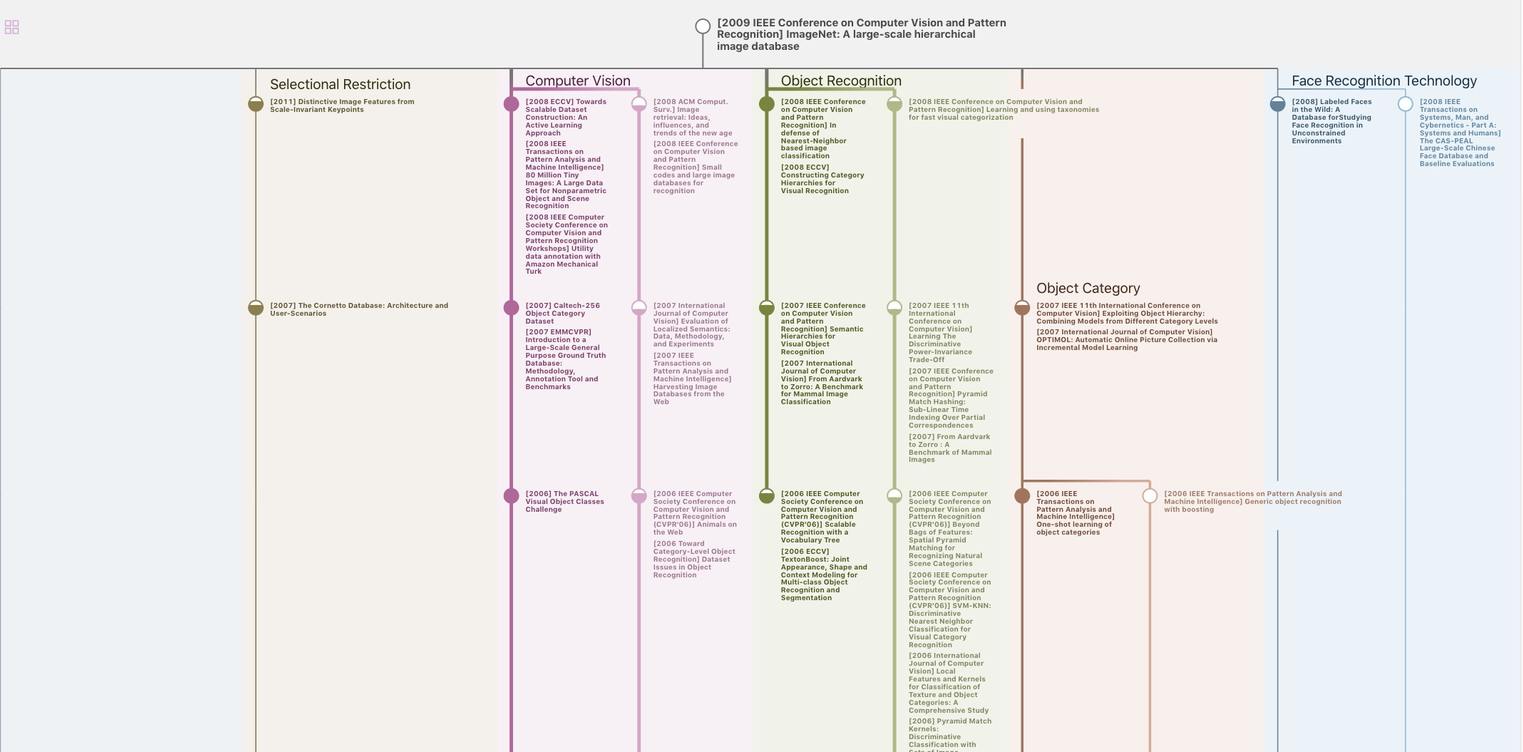
生成溯源树,研究论文发展脉络
Chat Paper
正在生成论文摘要