Computational chromatography: A machine learning strategy for demixing individual chemical components in complex mixtures
Proceedings of the National Academy of Sciences(2022)
摘要
Surface-enhanced Raman spectroscopy (SERS) holds exceptional promise as a streamlined chemical detection strategy for biological and environmental contaminants compared with current laboratory methods. Priority pollutants such as polycyclic aromatic hydrocarbons (PAHs), detectable in water and soil worldwide and known to induce multiple adverse health effects upon human exposure, are typically found in multicomponent mixtures. By combining the molecular fingerprinting capabilities of SERS with the signal separation and detection capabilities of machine learning (ML), we examine whether individual PAHs can be identified through an analysis of the SERS spectra of multicomponent PAH mixtures. We have developed an unsupervised ML method we call Characteristic Peak Extraction, a dimensionality reduction algorithm that extracts characteristic SERS peaks based on counts of detected peaks of the mixture. By analyzing the SERS spectra of two-component and four-component PAH mixtures where the concentration ratios of the various components vary, this algorithm is able to extract the spectra of each unknown component in the mixture of unknowns, which is then subsequently identified against a SERS spectral library of PAHs. Combining the molecular fingerprinting capabilities of SERS with the signal separation and detection capabilities of ML, this effort is a step toward the computational demixing of unknown chemical components occurring in complex multicomponent mixtures.
更多查看译文
关键词
machine learning,nanoparticles,nonnegative matrix factorization,polycyclic aromatic hydrocarbons,surface-enhanced Raman scattering
AI 理解论文
溯源树
样例
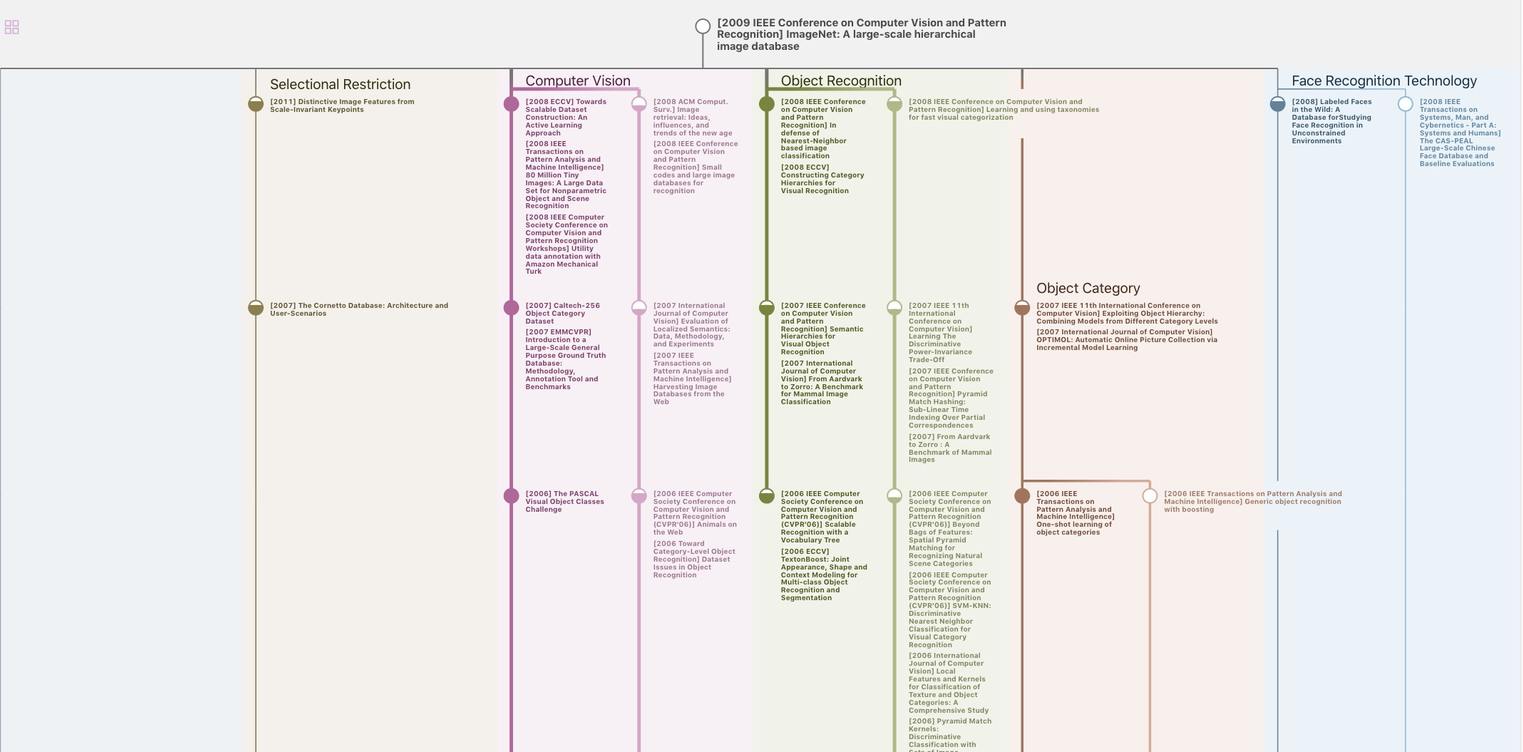
生成溯源树,研究论文发展脉络
Chat Paper
正在生成论文摘要