Most Probable Densest Subgraphs
arXiv (Cornell University)(2022)
摘要
Computing the densest subgraph is a primitive graph operation with critical applications in detecting communities, events, and anomalies in biological, social, Web, and financial networks. In this paper, we study the novel problem of Most Probable Densest Subgraph (MPDS) discovery in uncertain graphs: Find the node set that is the most likely to induce a densest subgraph in an uncertain graph. We further extend our problem by considering various notions of density, e.g., clique and pattern densities, studying the top-k MPDSs, and finding the node set with the largest containment probability within densest subgraphs. We show that it is #P-hard to compute the probability of a node set inducing a densest subgraph. We then devise sampling-based efficient algorithms, with end-to-end accuracy guarantees, to compute the MPDS. Our thorough experimental results and real-world case studies on brain and social networks validate the effectiveness, efficiency, and usefulness of our solution.
更多查看译文
关键词
Probabilistic Learning,Probabilistic Databases,Imprecise Probabilities,Probabilistic Graphical Models,Signal Processing on Graphs
AI 理解论文
溯源树
样例
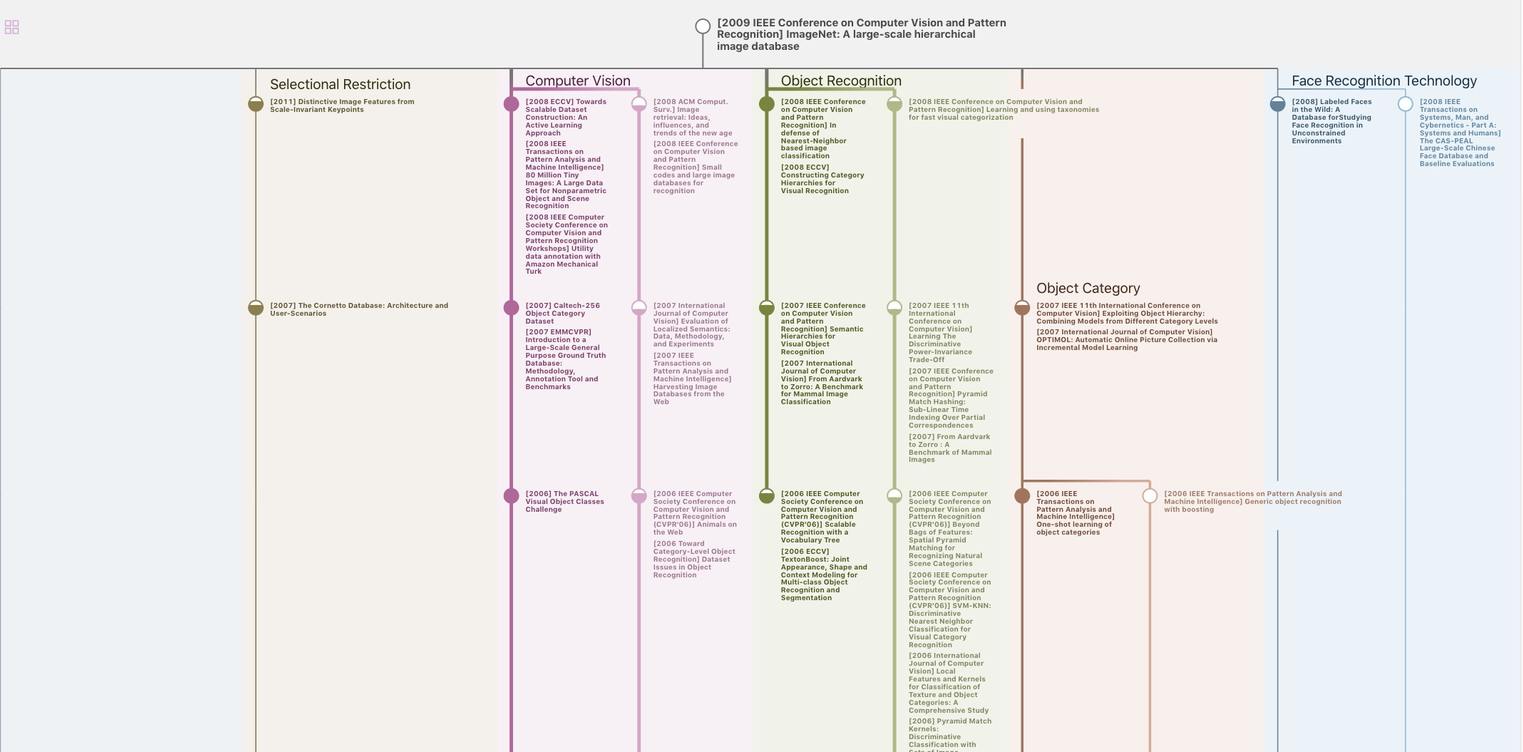
生成溯源树,研究论文发展脉络
Chat Paper
正在生成论文摘要