Multimodal CNN Networks for Brain Tumor Segmentation in MRI: A BraTS 2022 Challenge Solution
BRAINLESION: GLIOMA, MULTIPLE SCLEROSIS, STROKE AND TRAUMATIC BRAIN INJURIES, BRAINLES 2022(2023)
摘要
Automatic segmentation is essential for the brain tumor diagnosis, disease prognosis, and follow-up therapy of patients with gliomas. Still, accurate detection of gliomas and their sub-regions in multimodalMRI is very challenging due to the variety of scanners and imaging protocols. Over the last years, the BraTS Challenge has provided a large number of multi-institutional MRI scans as a benchmark for glioma segmentation algorithms. This paper describes our contribution to the BraTS 2022 Continuous Evaluation challenge. We propose a new ensemble of multiple deep learning frameworks namely, DeepSeg, nnU-Net, and DeepSCAN for automatic glioma boundaries detection in pre-operative MRI. It is worth noting that our ensemble models took first place in the final evaluation on the BraTS testing dataset with Dice scores of 0.9294, 0.8788, and 0.8803, and Hausdorf distance of 5.23, 13.54, and 12.05, for the whole tumor, tumor core, and enhancing tumor, respectively. Furthermore, the proposed ensemble method ranked first in the final ranking on another unseen test dataset, namely Sub-Saharan Africa dataset, achieving mean Dice scores of 0.9737, 0.9593, and 0.9022, and HD95 of 2.66, 1.72, 3.32 for the whole tumor, tumor core, and enhancing tumor, respectively. The docker image for the winning submission is publicly available at (https://hub.docker.com/r/razeineldin/camed22).
更多查看译文
关键词
BraTS,CNN,Ensemble,Glioma,MRI,Segmentation
AI 理解论文
溯源树
样例
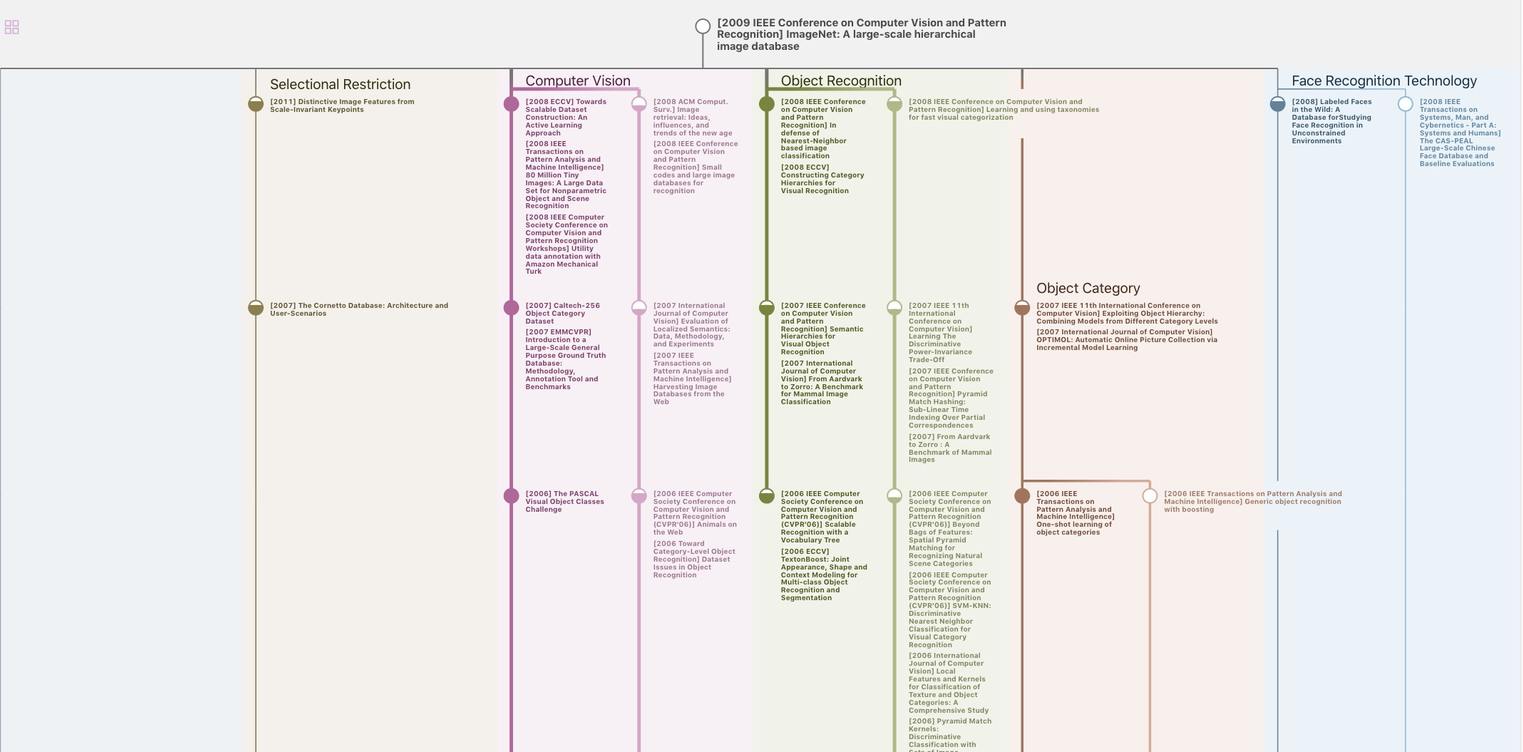
生成溯源树,研究论文发展脉络
Chat Paper
正在生成论文摘要