Synthetic Data Augmentation Using GAN For Improved Automated Visual Inspection
IFAC PAPERSONLINE(2023)
摘要
Quality control is a crucial activity manufacturing companies perform to ensure their products conform to the requirements and specifications. The introduction of artificial intelligence models enables to automate the visual quality inspection, speeding up the inspection process and ensuring all products are evaluated under the same criteria. In this research, we compare supervised and unsupervised defect detection techniques and explore data augmentation techniques to mitigate the data imbalance in the context of automated visual inspection. Furthermore, we use Generative Adversarial Networks for data augmentation to enhance the classifiers' discriminative performance. Our results show that state-of-the-art unsupervised defect detection does not match the performance of supervised models but can reduce the labeling workload if tolerating some labeling errors. Furthermore, the best classification performance was achieved considering GAN-based data generation with AUC ROC scores equal to or higher than 0,9898. We performed the research with real-world data provided by Philips Consumer Lifestyle BV. Copyright (C) 2023 The Authors.
更多查看译文
关键词
Manufacturing plant control,Intelligent manufacturing systems,Advanced manufacturing,Industry 4.0,Smart Manufacturing,Visual Inspection,Quality Inspection,Data Augmentation
AI 理解论文
溯源树
样例
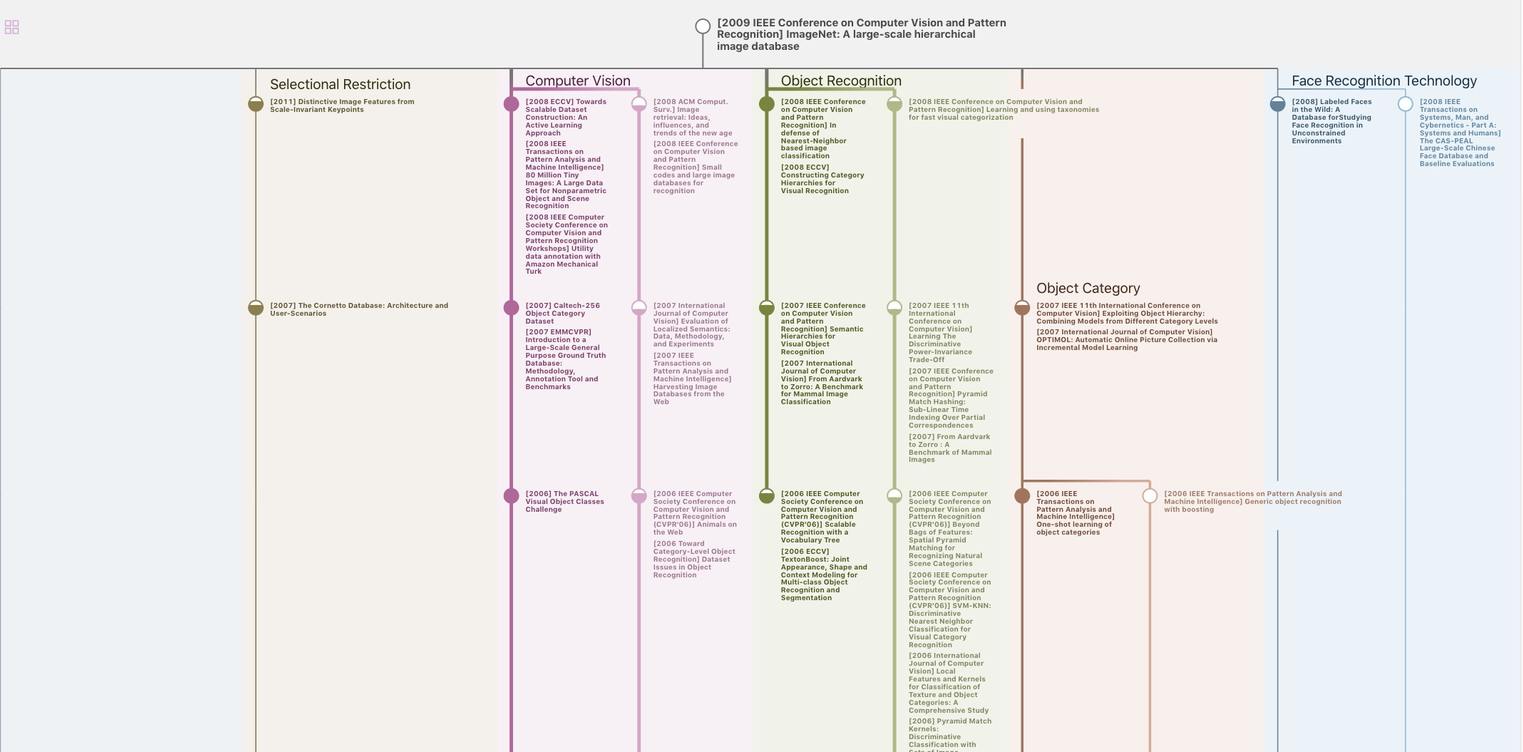
生成溯源树,研究论文发展脉络
Chat Paper
正在生成论文摘要