Deep Learning Subtraction Angiography: Improved Generalizability with Transfer Learning
Journal of vascular and interventional radiology : JVIR(2023)
摘要
Purpose: To investigate the utility and generalizability of deep learning subtraction angiography (DLSA) for generating synthetic digital subtraction angiography (DSA) images without misalignment artifacts. Materials and Methods: DSA images and native digital angiograms of the cerebral, hepatic, and splenic vasculature, both with and without motion artifacts, were retrospectively collected. Images were divided into a motion-free training set (n = 66 patients, 9,161 images) and a motion artifact-containing test set (n = 22 patients, 3,322 images). Using the motion-free set, the deep neural network pix2pix was trained to produce synthetic DSA images without misalignment artifacts directly from native digital angiograms. After training, the algorithm was tested on digital angiograms of hepatic and splenic vasculature with substantial motion. Four board-certified radiologists evaluated performance via visual assessment using a 5- grade Likert scale. Subgroup analyses were performed to analyze the impact of transfer learning and generalizability to novel vasculature. Results: Compared with the traditional DSA method, the proposed approach was found to generate synthetic DSA images with significantly fewer background artifacts (a mean rating of 1.9 [95% CI, 1.1-2.6] vs 3.5 [3.5-4.4]; P =.01) without a significant difference in foreground vascular detail (mean rating of 3.1 [2.6-3.5] vs 3.3 [2.8-3.8], P =.19) in both the hepatic and splenic vasculature. Transfer learning significantly improved the quality of generated images (P <.001). Conclusions: DLSA successfully generates synthetic angiograms without misalignment artifacts, is improved through transfer learning, and generalizes reliably to novel vasculature that was not included in the training data.
更多查看译文
关键词
BMI,DLSA,DSA,GAN,IQR,MSE,PSNR,SSIM
AI 理解论文
溯源树
样例
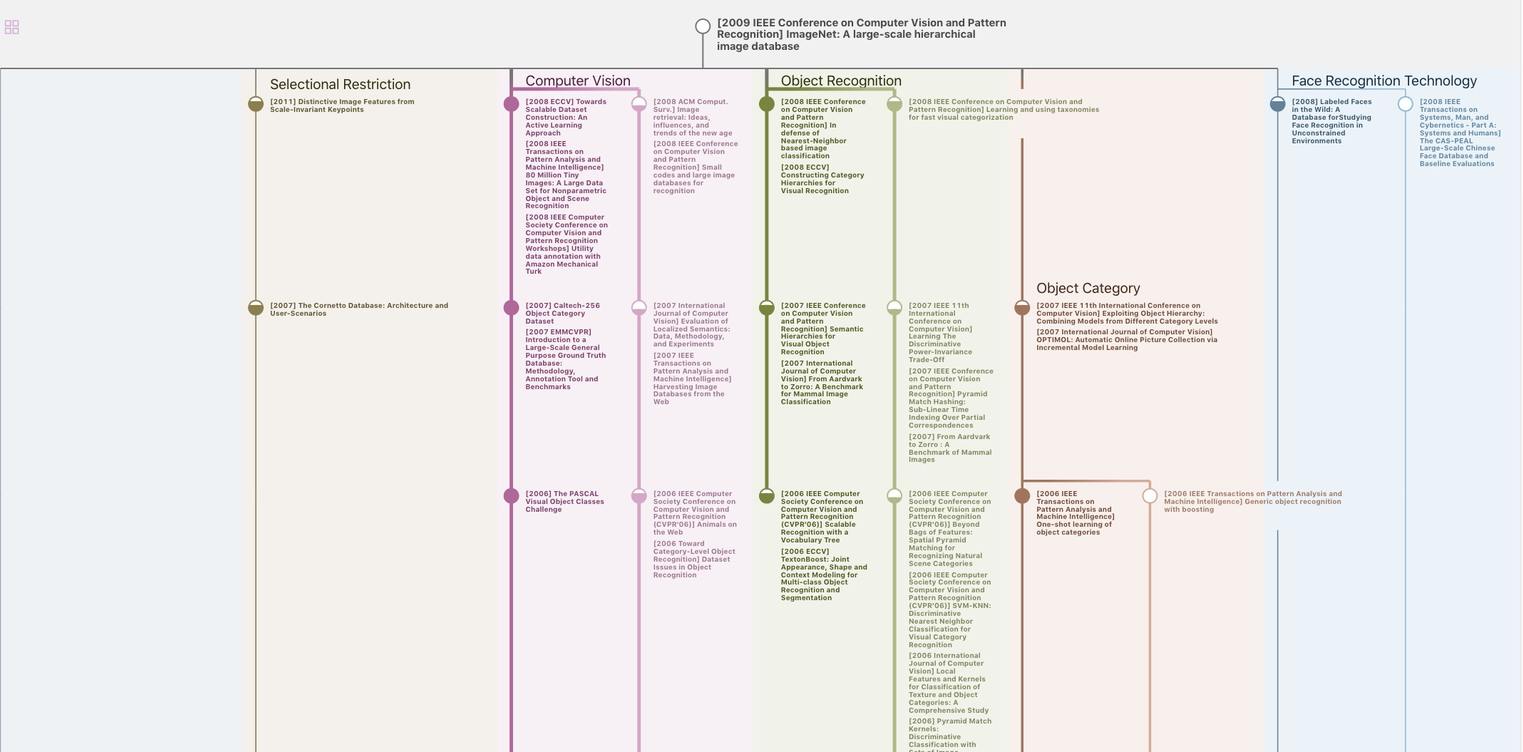
生成溯源树,研究论文发展脉络
Chat Paper
正在生成论文摘要