Week-ahead solar irradiance forecasting with deep sequence learning
Environmental Data Science(2022)
摘要
Abstract In order to enable widespread integration of solar energy into the power system, there is an increasing need to reduce the uncertainty associated with solar power output which requires major improvements in solar irradiance forecasting. While most recent works have addressed short-term (minutes or hours ahead) forecasting, through this work, we propose using deep sequence learning models for forecasting at longer lead times such as a week in advance, as this can play a significant role in future power system storage applications. Along with point forecasts, we also produce uncertainty estimates through probabilistic prediction and showcase the potential of our machine learning frameworks for a new and important application of longer lead time forecasting in this domain. Our study on the SURFRAD data over seven US cities compares various deep sequence models and the results are encouraging, demonstrating their superior performance against most benchmarks from the literature and a current machine learning-based probabilistic prediction baseline (previously applied to short-term solar forecasting).
更多查看译文
关键词
solar irradiance forecasting,deep sequence,week-ahead
AI 理解论文
溯源树
样例
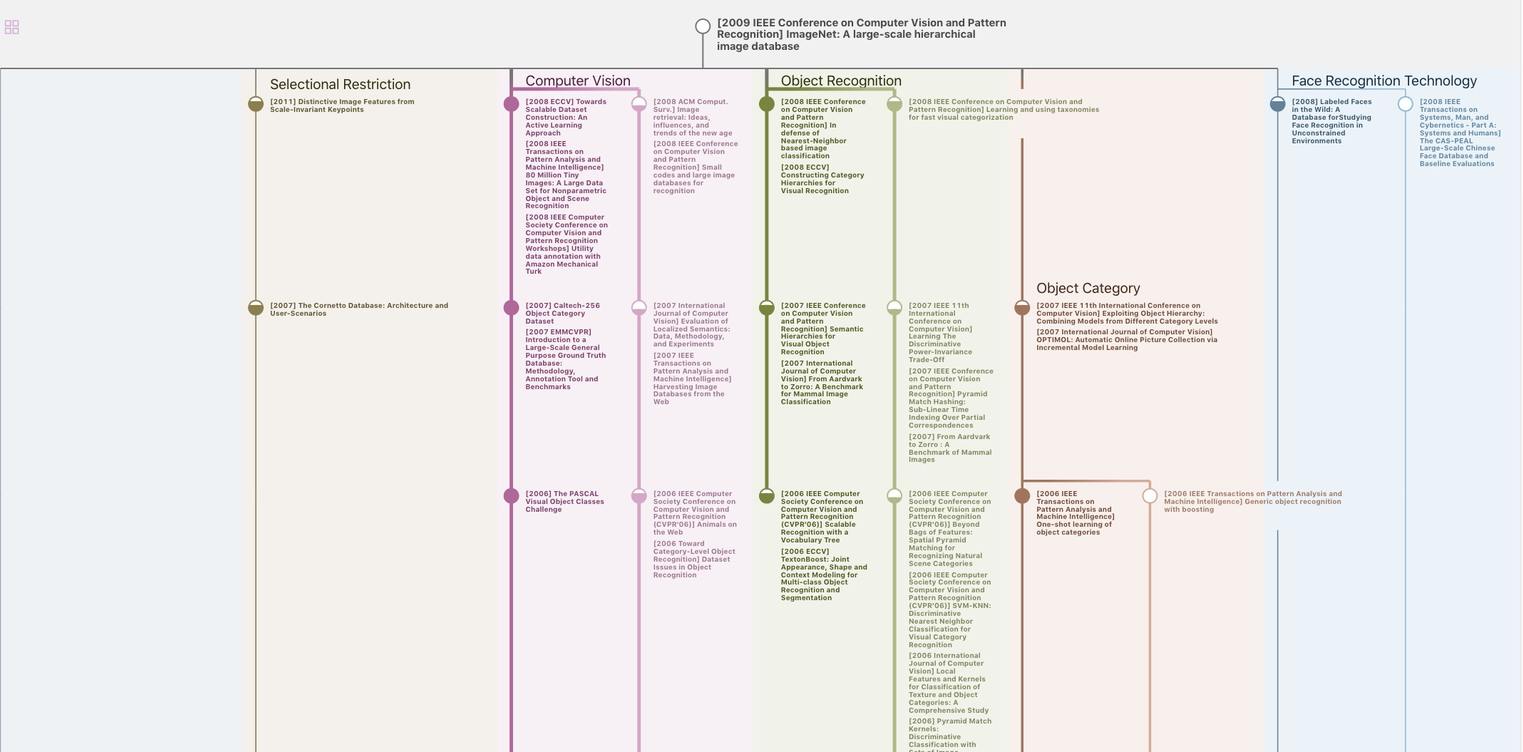
生成溯源树,研究论文发展脉络
Chat Paper
正在生成论文摘要